Real-World Light Field Image Super-Resolution Via Degradation Modulation.
IEEE transactions on neural networks and learning systems(2024)
摘要
Recent years have witnessed the great advances of deep neural networks (DNNs) in light field (LF) image super-resolution (SR). However, existing DNN-based LF image SR methods are developed on a single fixed degradation (e.g., bicubic downsampling), and thus cannot be applied to super-resolve real LF images with diverse degradation. In this article, we propose a simple yet effective method for real-world LF image SR. In our method, a practical LF degradation model is developed to formulate the degradation process of real LF images. Then, a convolutional neural network is designed to incorporate the degradation prior into the SR process. By training on LF images using our formulated degradation, our network can learn to modulate different degradation while incorporating both spatial and angular information in LF images. Extensive experiments on both synthetically degraded and real-world LF images demonstrate the effectiveness of our method. Compared with existing state-of-the-art single and LF image SR methods, our method achieves superior SR performance under a wide range of degradation, and generalizes better to real LF images. Codes and models are available at https://yingqianwang.github.io/LF-DMnet/.
更多查看译文
关键词
Degradation modulation,dynamic convolution,image super-resolution (SR),light field (LF)
AI 理解论文
溯源树
样例
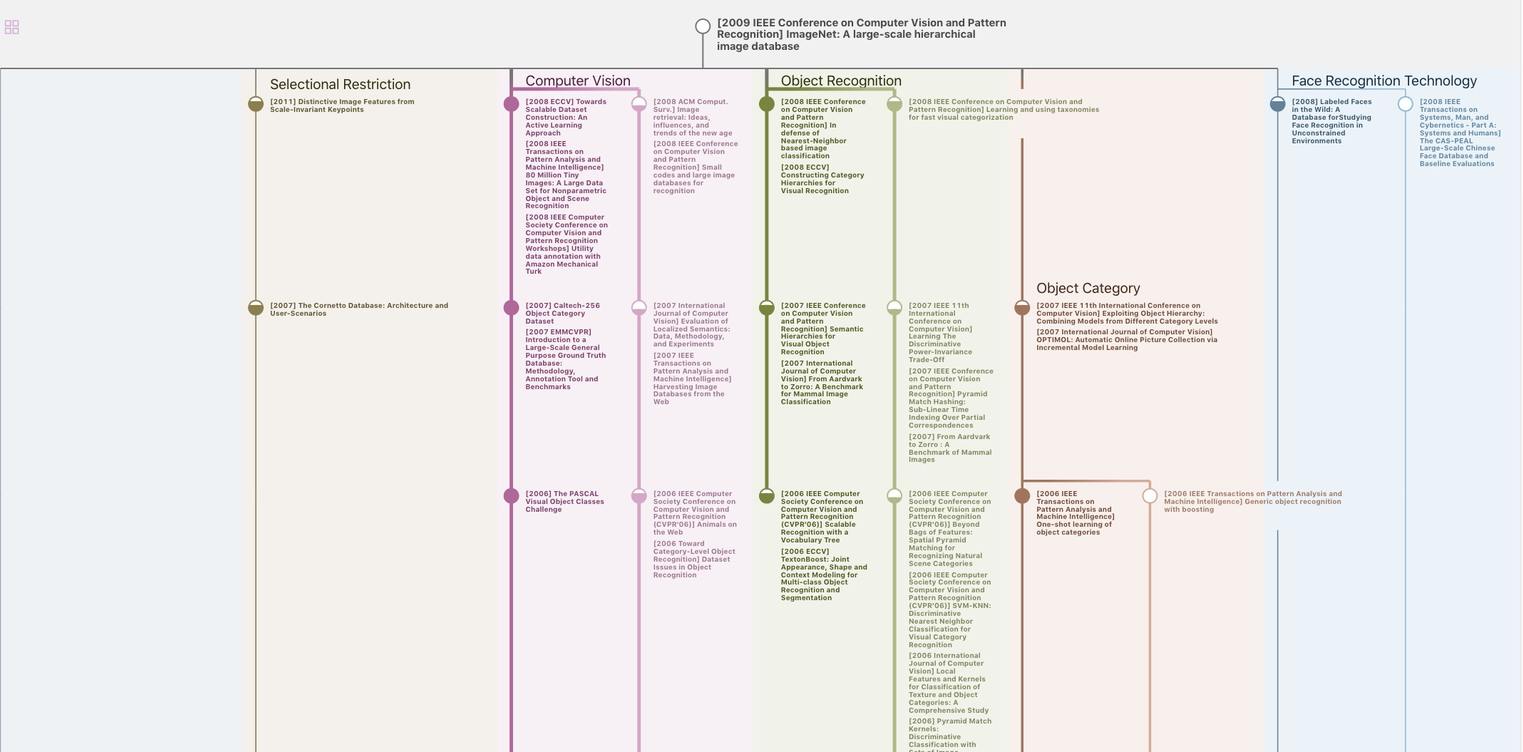
生成溯源树,研究论文发展脉络
Chat Paper
正在生成论文摘要