Takagi-Sugeno-Kang Fuzzy Systems for High-Dimensional Multi-Label Classification
IEEE Transactions on Fuzzy Systems(2024)
摘要
Multi-Label Classification (MLC) refers to associating each instance with multiple labels simultaneously. MLC has gained much importance due to its ability to better reflect the complexity of the real world classification problems. Fuzzy System (FS) has excellent nonlinear modeling capability and strong interpretability, which makes it a promising model for complex MLC problems. However, it is widely known that FS suffers from the “curse of dimensionality”. Here, an adaptive membership function (MF) along with its generalized version are proposed to address high-dimensional problems. These MFs can effectively overcome “numeric underflow” in FS while preserving interpretability as much as possible.On this basis, a novel fuzzy rule based multi-label classification framework called Multi-Label High-Dimensional Takagi-Sugeno-Kang Fuzzy System (ML-HDTSK FS) is proposed. This model can handle data with over ten thousand dimensionality. Additionally, MLHDTSK FS uses a decomposed label correlation learning strategy to efficiently capture both high and low levels of relationship between labels, and adopts a group L
21
penalty to realize the learning of label-specific features. Combining these two new multi-label learning strategies and the novel adaptive membership function, ML-HDTSK FS becomes a more powerful tool for various MLC problems. The effectiveness of ML-HDTSK FS is demonstrated on seventeen benchmark multi-label data sets, and its performance is compared with eleven MLC algorithms. The experimental results confirm the validity of the proposed MLHDTSK FS, and demonstrate the superiority of it in dealing with MLC problems, especially for high dimensional ones.
更多查看译文
关键词
Takagi-Sugeno-Kang fuzzy systems,multi-label classification,high-dimensional data,label correlation learning,label-specific feature learning
AI 理解论文
溯源树
样例
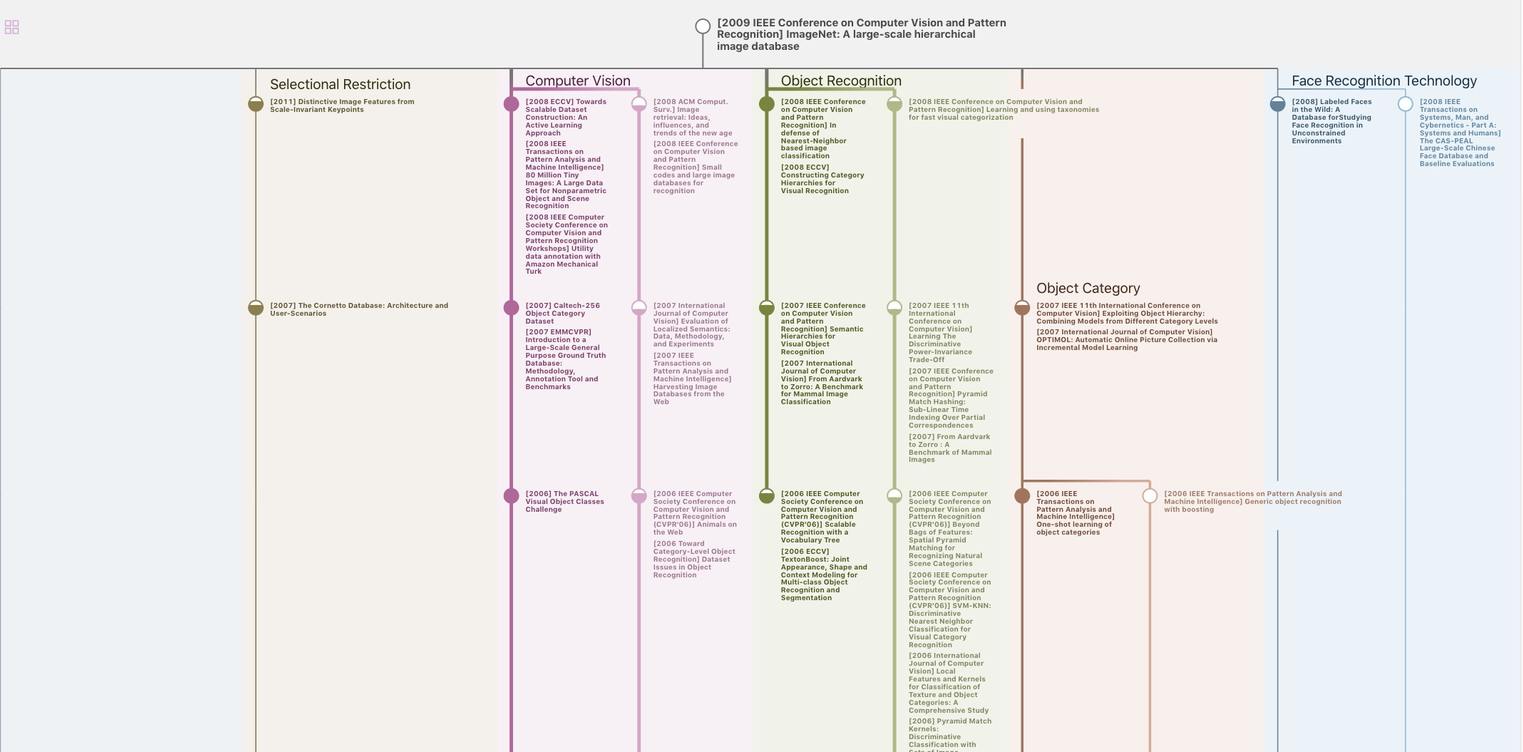
生成溯源树,研究论文发展脉络
Chat Paper
正在生成论文摘要