Lexicon-based fine-tuning of multilingual language models for low-resource language sentiment analysis
CAAI TRANSACTIONS ON INTELLIGENCE TECHNOLOGY(2024)
摘要
Pre-trained multilingual language models (PMLMs) such as mBERT and XLM-R have shown good cross-lingual transferability. However, they are not specifically trained to capture cross-lingual signals concerning sentiment words. This poses a disadvantage for low-resource languages (LRLs) that are under-represented in these models. To better fine-tune these models for sentiment classification in LRLs, a novel intermediate task fine-tuning (ITFT) technique based on a sentiment lexicon of a high-resource language (HRL) is introduced. The authors experiment with LRLs Sinhala, Tamil and Bengali for a 3-class sentiment classification task and show that this method outperforms vanilla fine-tuning of the PMLM. It also outperforms or is on-par with basic ITFT that relies on an HRL sentiment classification dataset.
更多查看译文
关键词
deep learning,natural languages,natural language processing
AI 理解论文
溯源树
样例
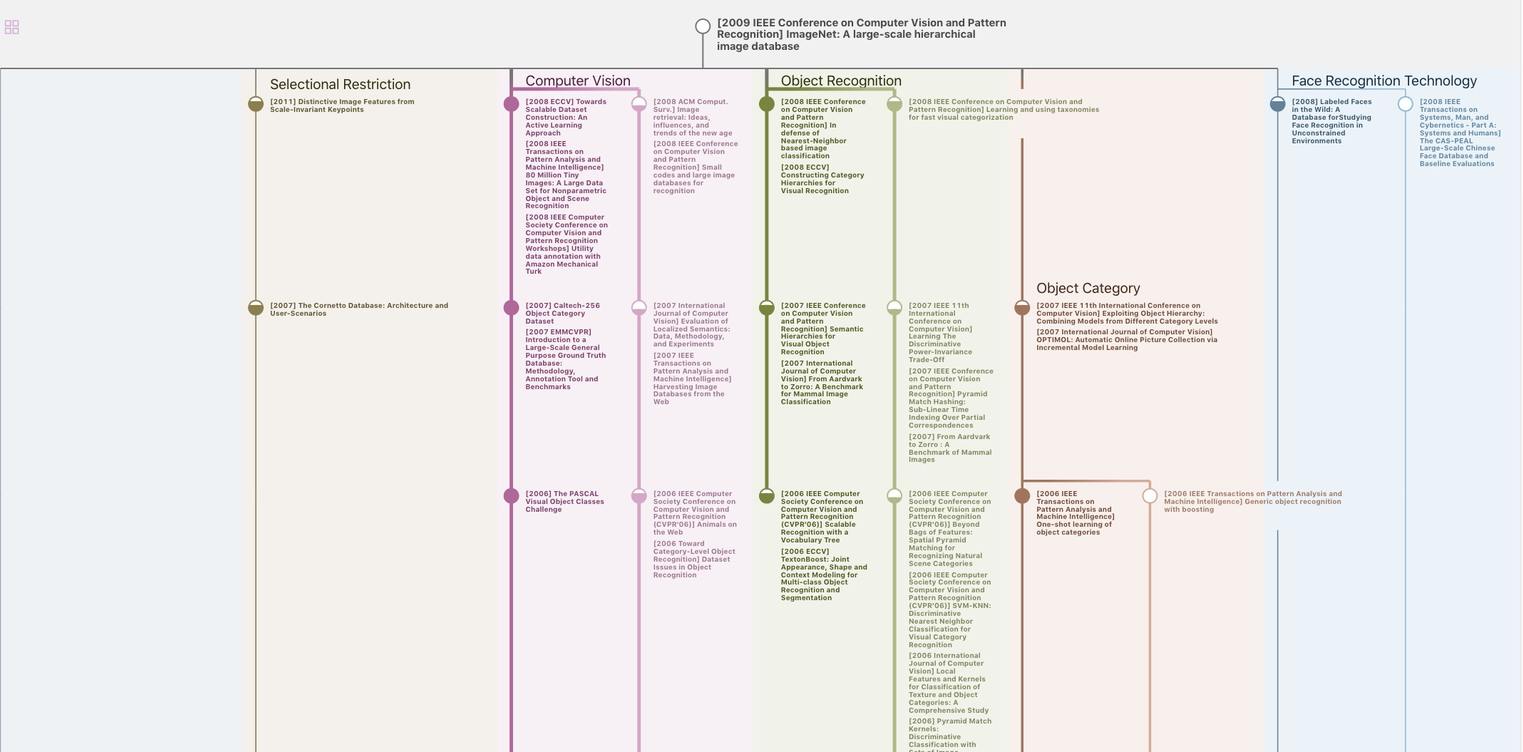
生成溯源树,研究论文发展脉络
Chat Paper
正在生成论文摘要