Optimizing machine learning techniques and SHapley Additive exPlanations (SHAP) analysis for the compressive property of self-compacting concrete
Materials Today Communications(2024)
摘要
This study undertook an examination of the effectiveness of employing machine learning (ML) techniques to estimate the compressive strength (CS) of self-compacting concrete (SCC). Multiple techniques were utilized, such as a decision tree (DT), a random forest regressor (RFR), an AdaBoost regressor (AR), and a gradient boosting regressor (GBR). Additionally, the research investigated the impacts and correlations among input variables and the CS of SCC using the SHapley Additive exPlanations (SHAP) technique. A full dataset was created, including a single dependent variable and ten independent ones. Although GBR and DT methods proved effective, the research indicated that AR and RFR provided the most accurate predictions of SCC's CS. The AR and RFR models demonstrated superior performance compared to the DT and GBR models, as evidenced by their R2 values of 0.90 and 0.91, respectively, outperforming the DT and GBR models with R2 values of 0.85 and 0.88, respectively. The SHAP investigation revealed that the concentration of superplasticizer and cement in the mixture had an extensive impact on the CS of SCC. The findings of this study indicate that both independent and ensemble ML methods had comparable predictive abilities in estimating SCC's CS. The effectiveness of guaranteeing SCC quality will be greatly enhanced by using these procedures.
更多查看译文
关键词
Compressive strength,Machine learning,Self-compacting concrete,Modeling,Prediction models
AI 理解论文
溯源树
样例
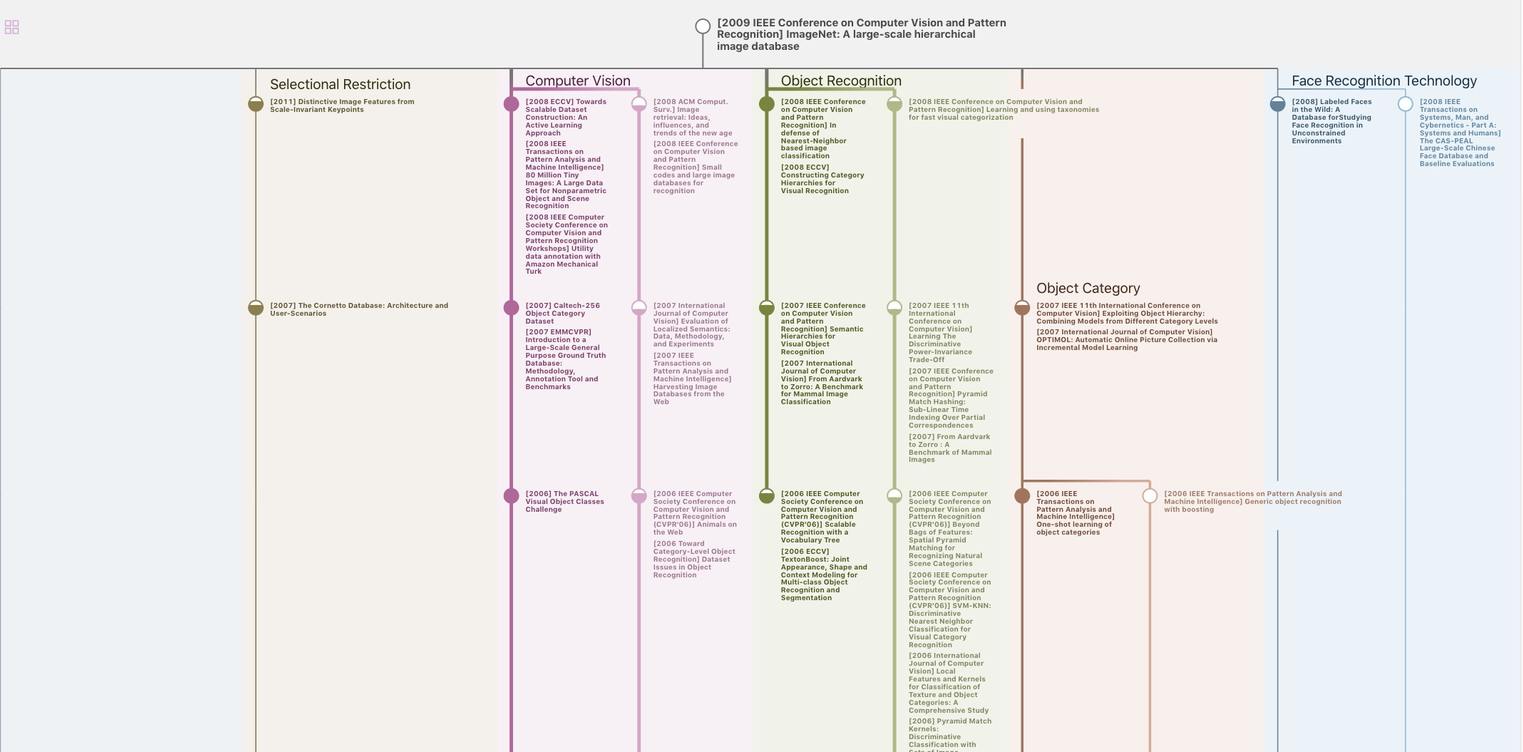
生成溯源树,研究论文发展脉络
Chat Paper
正在生成论文摘要