LCSNet: Light-Weighted Convolution-Based Segmentation Method with Separable Multi-Directional Convolution Module for Concrete Crack Segmentation in Drones
ELECTRONICS(2024)
摘要
Concrete cracks pose significant safety hazards to buildings, and semantic segmentation models based on deep learning have achieved state-of-the-art results in concrete crack detection. However, these models usually have a large model size which is impossible to use in drones. To solve this problem, we propose a Light-Weighted Convolution-Based Segmentation Method with a Separable Multi-Directional Convolution Module (LCSNet). In our proposed method, light-weighted convolution is used to substitute all traditional convolutions. In addition, a light-weighted structure named a Separable Multi-Directional Convolution Module (SMDCM) is used to substitute traditional parallel structures or attention modules to learn contextual or detail features. Thus, the ability to extract the contextual feature information of the model can be retained while the computational complexity is largely reduced. Through these two improvements, the model size of the proposed model can have a lower computational complexity. The experimental results show that our proposed LCSNet can achieve accuracies of 94.2%, 83.6%, 99.2%, and 83.3% on the Cracktree200, CRACK500, CFD, and RECrack datasets, respectively, which are higher than those of traditional models. However, the model size of our LCSNet is only 2M.
更多查看译文
关键词
artificial intelligence,machine learning,deep neural network,image segmentation application,crack segmentation
AI 理解论文
溯源树
样例
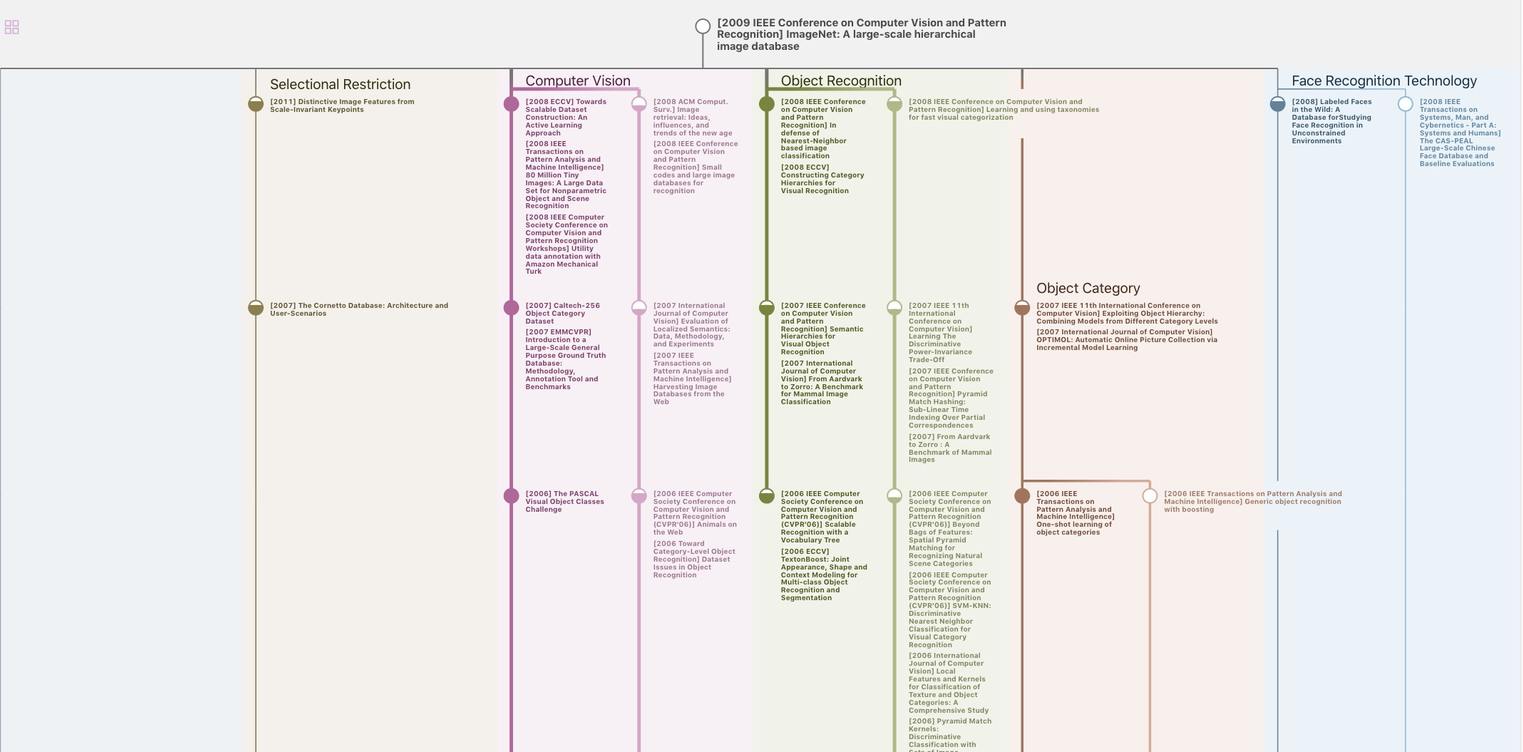
生成溯源树,研究论文发展脉络
Chat Paper
正在生成论文摘要