Robust Federated Contrastive Recommender System against Model Poisoning Attack
CoRR(2024)
摘要
Federated Recommender Systems (FedRecs) have garnered increasing attention
recently, thanks to their privacy-preserving benefits. However, the
decentralized and open characteristics of current FedRecs present two dilemmas.
First, the performance of FedRecs is compromised due to highly sparse on-device
data for each client. Second, the system's robustness is undermined by the
vulnerability to model poisoning attacks launched by malicious users. In this
paper, we introduce a novel contrastive learning framework designed to fully
leverage the client's sparse data through embedding augmentation, referred to
as CL4FedRec. Unlike previous contrastive learning approaches in FedRecs that
necessitate clients to share their private parameters, our CL4FedRec aligns
with the basic FedRec learning protocol, ensuring compatibility with most
existing FedRec implementations. We then evaluate the robustness of FedRecs
equipped with CL4FedRec by subjecting it to several state-of-the-art model
poisoning attacks. Surprisingly, our observations reveal that contrastive
learning tends to exacerbate the vulnerability of FedRecs to these attacks.
This is attributed to the enhanced embedding uniformity, making the polluted
target item embedding easily proximate to popular items. Based on this insight,
we propose an enhanced and robust version of CL4FedRec (rCL4FedRec) by
introducing a regularizer to maintain the distance among item embeddings with
different popularity levels. Extensive experiments conducted on four commonly
used recommendation datasets demonstrate that CL4FedRec significantly enhances
both the model's performance and the robustness of FedRecs.
更多查看译文
AI 理解论文
溯源树
样例
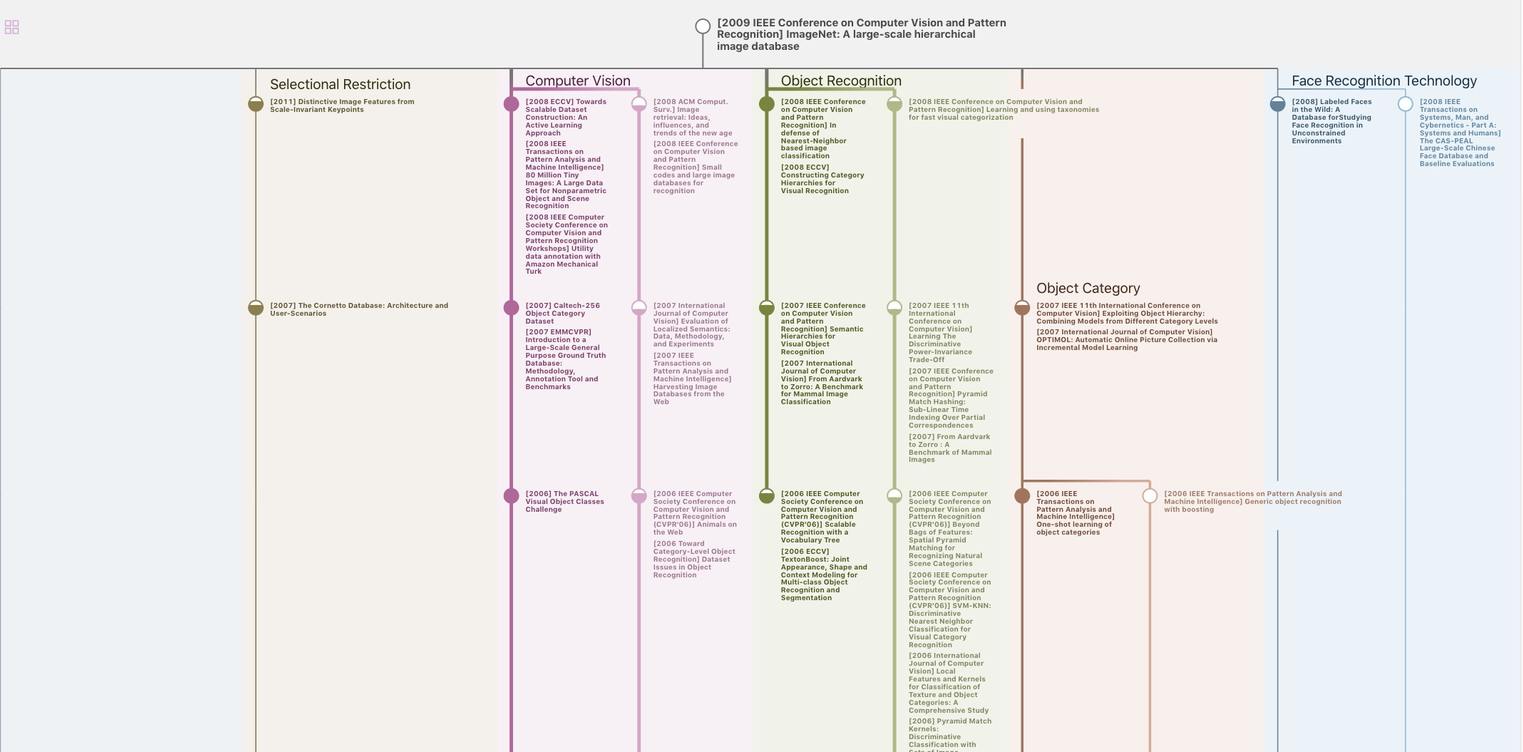
生成溯源树,研究论文发展脉络
Chat Paper
正在生成论文摘要