Land Cover Mapping of Mixed Classes using 2D CNN with Multi-Frequency SAR Data
Advances in Space Research(2024)
摘要
Synthetic aperture radar (SAR) data obtained at multiple frequencies and polarizations offers valuable complementary information for classifying mixed classes that exhibit similar backscattering response. Although deep learning-based convolutional neural networks (CNNs) effectively extract features from multi-frequency SAR data, the arbitrary ordering of SAR features may hinder optimal convolution of the best feature sub-space for a specific class and underutilize available multi-frequency data. To address this, a novel CNN transforming SAR feature-space from 1-D to 2-D and employing varied dilation-rate convolutions is introduced. This transformation maximizes unique and localized feature combinations, efficiently utilizing the available feature sub-spaces and extracting discriminative features for accurate classifications, addressing the challenge of arbitrary band neighborhoods. Utilizing dual-polarization SAR data from ALOS-2 PALSAR-2 and Sentinel-1 sensors, the proposed CNN achieves an average f-score of 0.97 and a kappa coefficient of 0.97, an improvement of 11%, 7% and 3% in OA compared to the 1-D, 2-D and 3-D CNN classifiers, without feature transformation. The classifier's generalization ability is evaluated using ground truth knowledge of various heterogeneous classes, and the proposed CNN classifier outperforms others in terms of accuracy metrics and generalization ability.
更多查看译文
关键词
Multi frequency SAR,mixed classes,deep learning,feature transformation,dilated CNNs
AI 理解论文
溯源树
样例
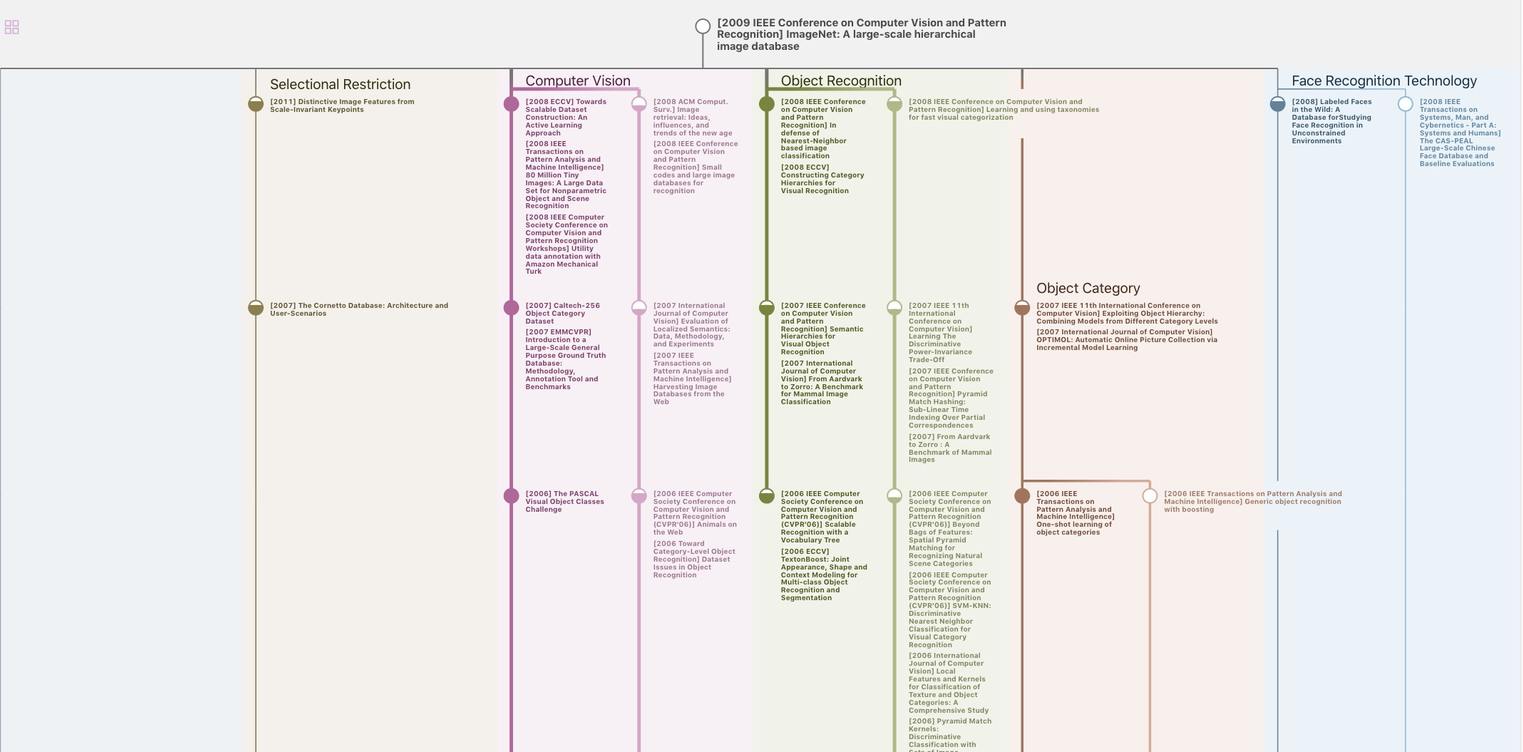
生成溯源树,研究论文发展脉络
Chat Paper
正在生成论文摘要