Effective Video Summarization by Extracting Parameter-free Motion Attention
ACM Transactions on Multimedia Computing, Communications, and Applications(2024)
摘要
Video summarization remains a challenging task despite increasing research efforts. Traditional methods focus solely on long-range temporal modeling of video frames, overlooking important local motion information which can not be captured by frame-level video representations. In this paper, we propose the Parameter-free Motion Attention Module (PMAM) to exploit the crucial motion clues potentially contained in adjacent video frames, using a multi-head attention architecture. The PMAM requires no additional training for model parameters, leading to an efficient and effective understanding of video dynamics. Moreover, we introduce the Multi-feature Motion Attention Network (MMAN), integrating the parameter-free motion attention module with local and global multi-head attention based on object-centric and scene-centric video representations. The synergistic combination of local motion information, extracted by the proposed PMAM, with long-range interactions modeled by the local and global multi-head attention mechanism, can significantly enhance the performance of video summarization. Extensive experimental results on the benchmark datasets, SumMe and TVSum, demonstrate that the proposed MMAN outperforms other state-of-the-art methods, resulting in remarkable performance gains.
更多查看译文
AI 理解论文
溯源树
样例
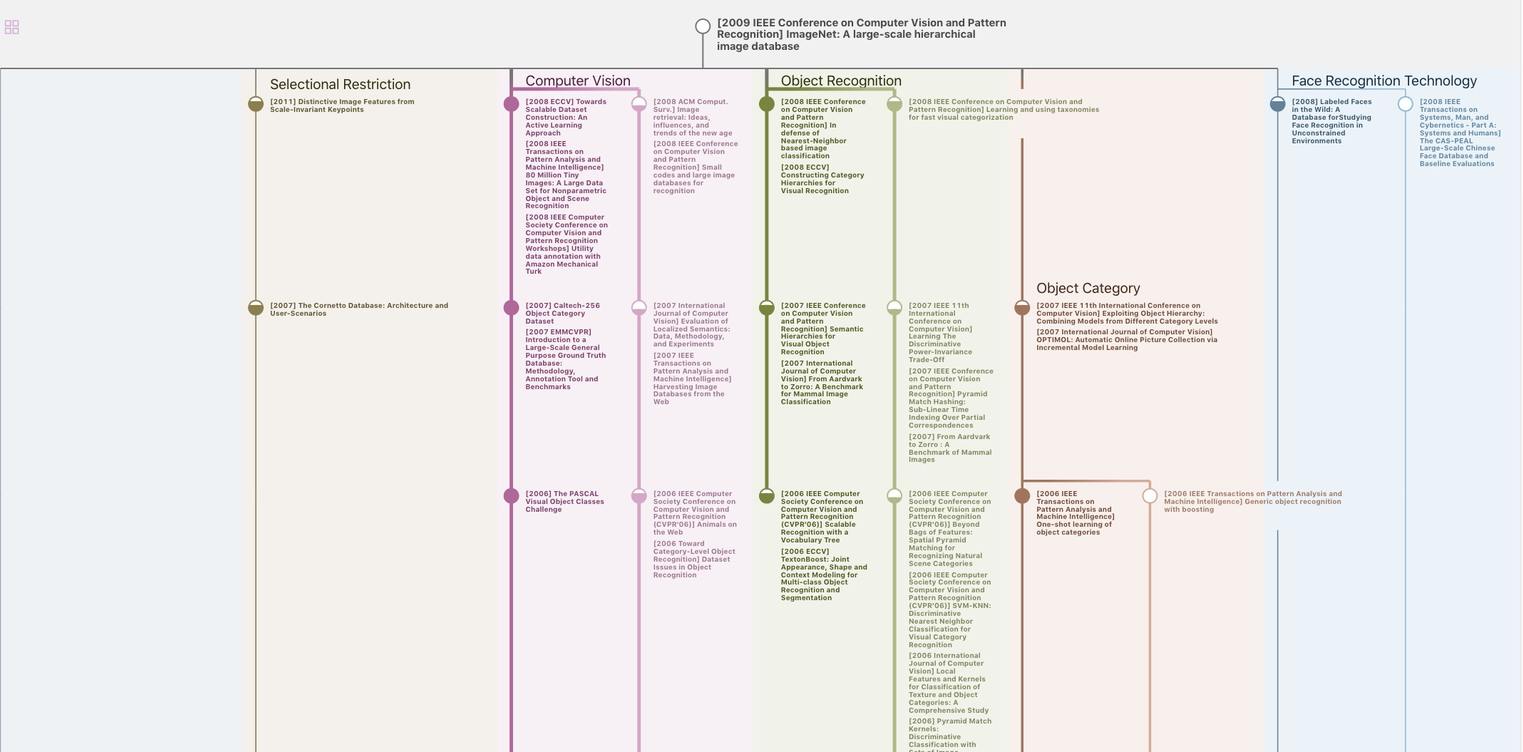
生成溯源树,研究论文发展脉络
Chat Paper
正在生成论文摘要