Knowledge distillation model for Acute Lymphoblastic Leukemia Detection: Exploring the impact of nesterov-accelerated adaptive moment estimation optimizer
Biomedical Signal Processing and Control(2024)
摘要
Acute lymphoblastic leukemia (ALL) is a malignancy that primarily affects the bone marrow and bloodstream, characterized by the rapid proliferation of immature lymphoblasts. In the realm of medical diagnosis, the integration of artificial intelligence (AI) presents an opportunity to revolutionize the detection of ALL. This integration has the potential to significantly enhance diagnostic accuracy, streamline the diagnostic process, and mitigate the risk of human error, which is particularly valuable when analyzing data collected from monitoring devices. This paper introduces two novel architectures designed for the detection of ALL. The first approach, known as Knowledge Nevestro Classification (KNC), incorporates the concept of knowledge distillation. This method leverages two teacher models, DenseNet and ResNet (50), which have undergone meticulous optimization employing both the Adam and Nadam optimizers. This dual optimization approach allows for a comprehensive evaluation of their respective outcomes. The second approach introduces the Nevestro DenseNet (50) Attention (DAN) architecture, which represents a variant of the DenseNet with Attention (NDA) architecture. This custom architecture is purpose-built for the specific task of ALL detection and has been meticulously fine-tuned using the Nadam optimizer. The results show impressive metrics, with accuracy, sensitivity, specificity, precision, and F1-Score measures reaching 0.991, 0.9945, 0.9924, 0.9945, and 0.9945, respectively.
更多查看译文
关键词
Leukaemia cancer,Knowledge distillation,Nevestro,DensNet,ResNet (50)
AI 理解论文
溯源树
样例
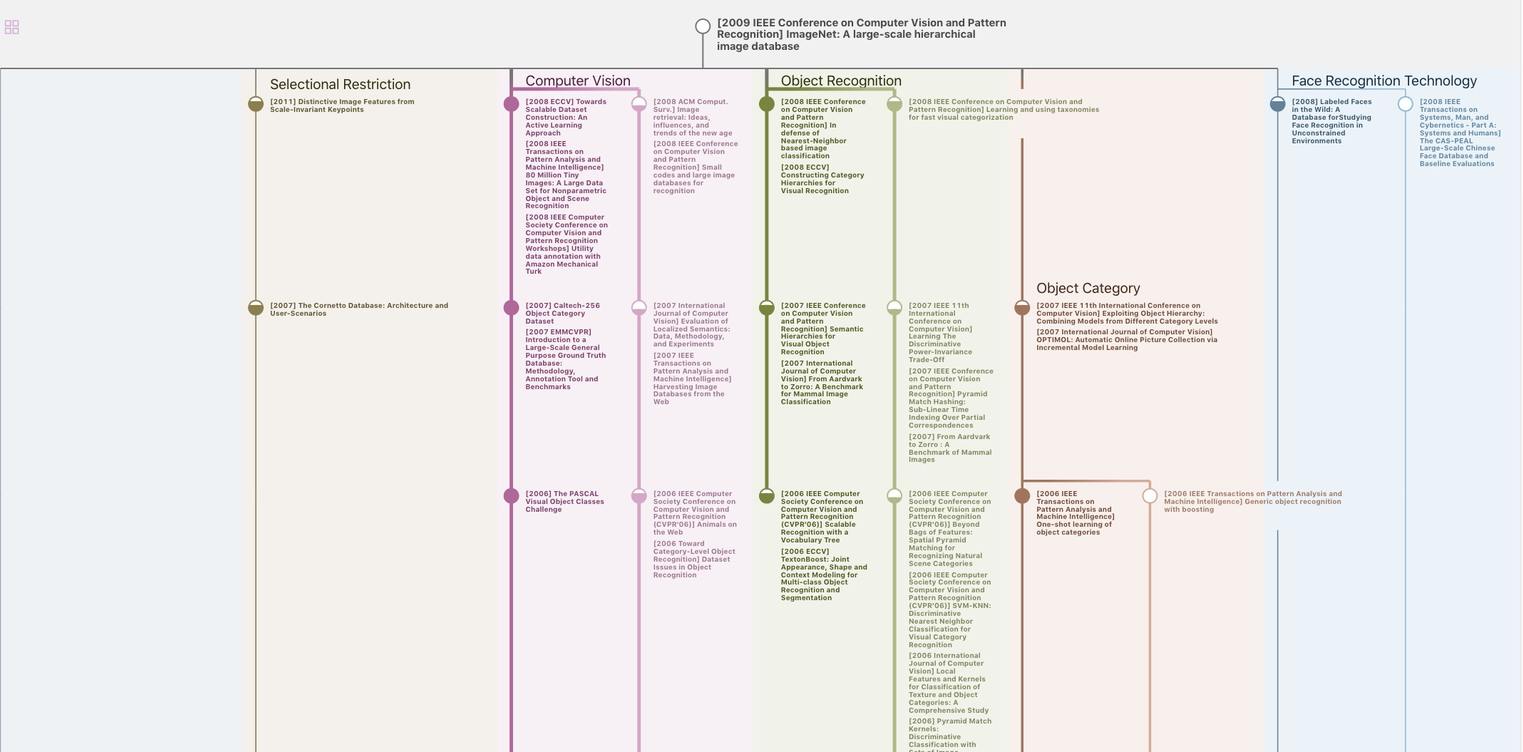
生成溯源树,研究论文发展脉络
Chat Paper
正在生成论文摘要