An Introduction to Machine and Deep Learning Methods for Cloud Masking Applications
APPLIED SCIENCES-BASEL(2024)
Abstract
Cloud cover assessment is crucial for meteorology, Earth observation, and environmental monitoring, providing valuable data for weather forecasting, climate modeling, and remote sensing activities. Depending on the specific purpose, identifying and accounting for pixels affected by clouds is essential in spectral remote sensing imagery. In applications such as land monitoring and various remote sensing activities, detecting/removing cloud-contaminated pixels is crucial to ensuring the accuracy of advanced processing of satellite imagery. Typically, the objective of cloud masking is to produce an image where every pixel in a satellite spectral image is categorized as either clear or cloudy. Nevertheless, there is also a prevalent approach in the literature that yields a multi-class output. With the progress in Machine and Deep Learning, coupled with the accelerated capabilities of GPUs, and the abundance of available remote sensing data, novel opportunities and methods for cloud detection have emerged, improving the accuracy and the efficiency of the algorithms. This paper provides a review of these last methods for cloud masking in multispectral satellite imagery, with emphasis on the Deep Learning approach, highlighting their benefits and challenges.
MoreTranslated text
Key words
cloud masking,deep learning,machine learning,image analysis
AI Read Science
Must-Reading Tree
Example
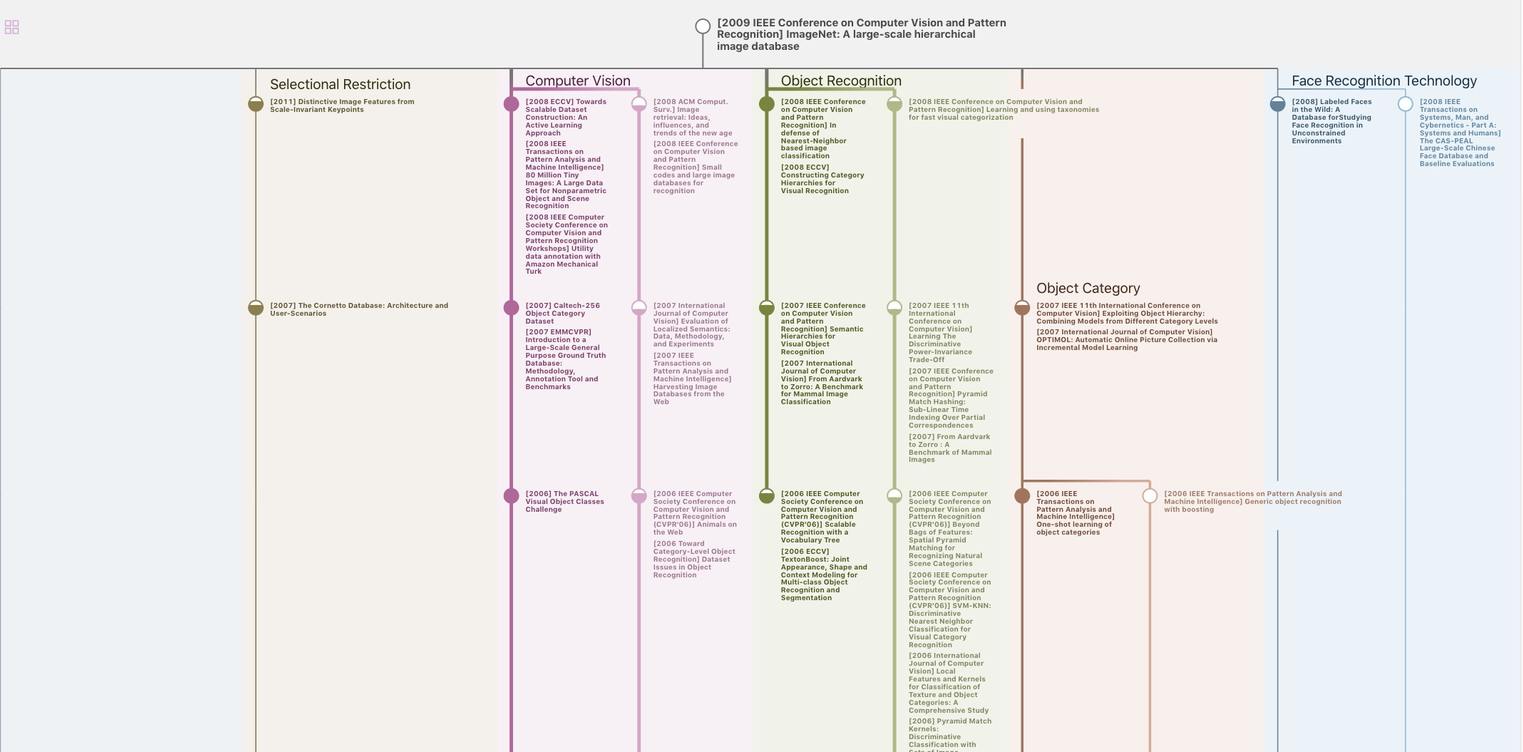
Generate MRT to find the research sequence of this paper
Chat Paper
Summary is being generated by the instructions you defined