Rethinking multi-spatial information for transferable adversarial attacks on speaker recognition systems
CAAI TRANSACTIONS ON INTELLIGENCE TECHNOLOGY(2024)
摘要
Adversarial attacks have been posing significant security concerns to intelligent systems, such as speaker recognition systems (SRSs). Most attacks assume the neural networks in the systems are known beforehand, while black-box attacks are proposed without such information to meet practical situations. Existing black-box attacks improve transferability by integrating multiple models or training on multiple datasets, but these methods are costly. Motivated by the optimisation strategy with spatial information on the perturbed paths and samples, we propose a Dual Spatial Momentum Iterative Fast Gradient Sign Method (DS-MI-FGSM) to improve the transferability of black-box attacks against SRSs. Specifically, DS-MI-FGSM only needs a single data and one model as the input; by extending to the data and model neighbouring spaces, it generates adversarial examples against the integrating models. To reduce the risk of overfitting, DS-MI-FGSM also introduces gradient masking to improve transferability. The authors conduct extensive experiments regarding the speaker recognition task, and the results demonstrate the effectiveness of their method, which can achieve up to 92% attack success rate on the victim model in black-box scenarios with only one known model.
更多查看译文
关键词
speaker recognition,spoofing attacks
AI 理解论文
溯源树
样例
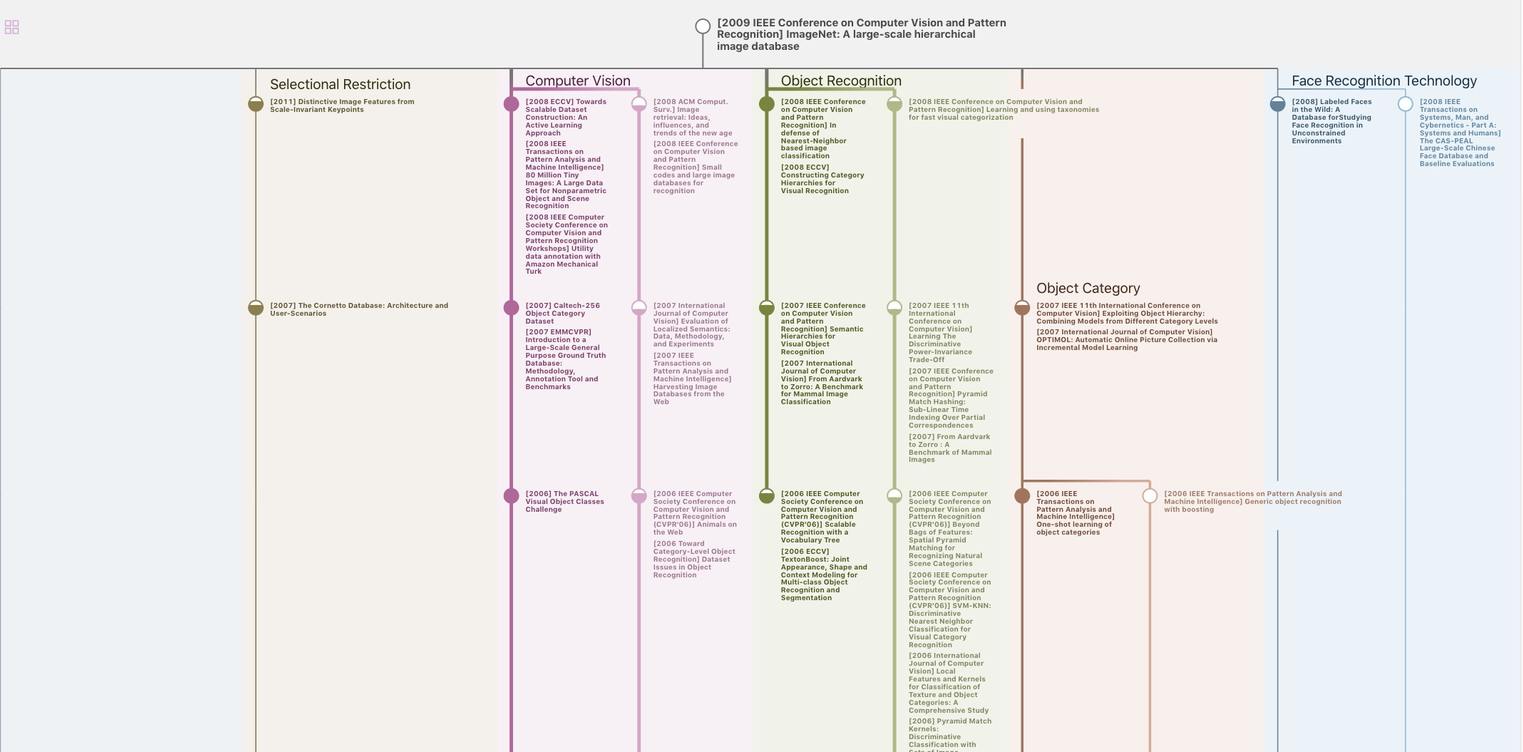
生成溯源树,研究论文发展脉络
Chat Paper
正在生成论文摘要