Multi-level joint distributed alignment-based domain adaptation for cross-scenario strip defect recognition
Journal of Intelligent Manufacturing(2024)
摘要
Deep learning-based methods for object recognition under closed situation have achieved remarkable progress. However, the data distribution of cross-scenario images of strip steel defects varies considerably due to imaging factors such as camera type, parameters, and unstable illumination in different scenarios. Large domain gap and limited diversity may jeopardize the generalization of model over the cross-scenario target domain. In this article, we propose a novel Multi-Level Joint Distribution Alignment Domain Adaptation model (MLJDA). The model unites different granularity perspectives from pixel-, feature-, and category-level to align cross-domain data. First, we propose a parameter sharing style-expansion module based on pixel-level feature mapping, which synthesizes multi-source domain data with different distributions compared to source domain to improve the generalization performance of model towards unknown scenarios and synthesizes similar multi-target domain data. Second, the generated multi-source domain and multi-target domain data are subjected to feature-level adversarial learning to obtain domain-invariant representations. Finally, we design multi-domain collaborative pseudo-label verification based on a voting mechanism to purify cross-scenario target domain category labels. Experimental results on the Handan Steel Defect Dataset and the public Severstal Steel Defect Dataset show that MLJDA can effectively solve the problem of recognizing strip steel defects across scenarios. The MLJDA achieve a F1 score of 98 % and 90 % on the Handan Steel Defect Dataset and Severstal Steel Defect, respectively.
更多查看译文
关键词
Cross-scenario,Strip surface defect recognition,Unsupervised domain adaptation (UDA),Data distribution alignment
AI 理解论文
溯源树
样例
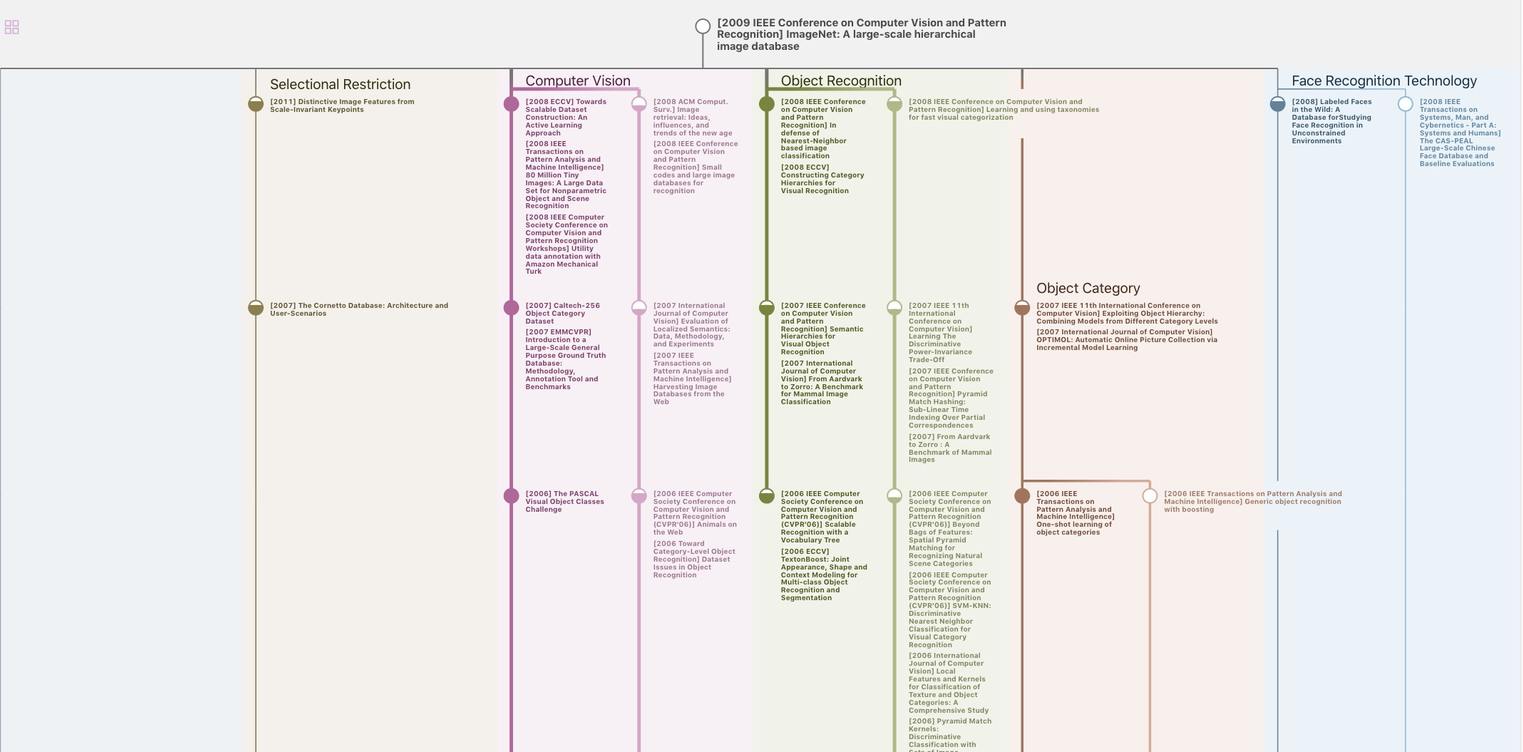
生成溯源树,研究论文发展脉络
Chat Paper
正在生成论文摘要