Active-learning for Global Optimization of Ni-Ceria Nanoparticles: the Case of Ce4-xNixO8-x (x=1, 2, 3)
Journal of Computational Chemistry(2024)
Key words
active learning,DFT,global optimization,machine learning,Ni-ceria nanoparticles
AI Read Science
Must-Reading Tree
Example
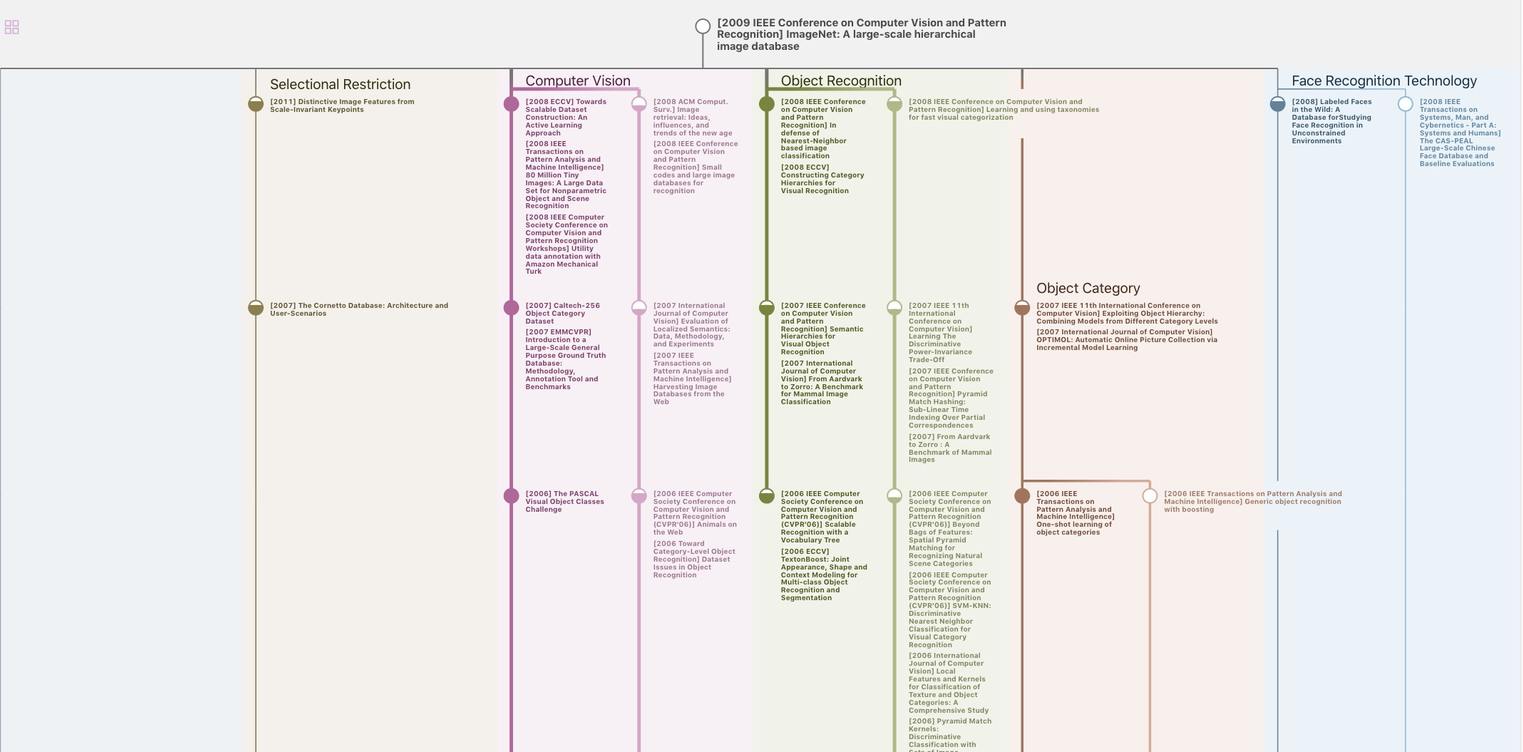
Generate MRT to find the research sequence of this paper
Chat Paper
Summary is being generated by the instructions you defined