Numerically Optimized Fourier Transform-Based Beamforming Accelerated by Neural Networks
APPLIED SCIENCES-BASEL(2024)
摘要
Conventional beamforming methods for reconfigurable reflector antennas assume full control over the amplitude and phase of the reflected field. Here, we develop a novel beamforming methodology for reflecting Programmable Metasurfaces (PMS) with capacitive memory. Although utilizing such fully reactive PMS simplifies antenna design and reduces energy consumption, the PMS reflection magnitude is unity and thus a global optimization of the reflection phases over the PMS unit cells is required in each beamforming scenario. We propose an implementation of such an optimization method rooted in the traditional Fourier transform-based beamforming and evaluate its performance. Additionally, we show that a pair of trained feed-forward neural networks (FFNN) with one input, one hidden, and one output layer can replace time-consuming global optimizations in the case of a PMS comprising 3x10 unit cells. We train the FFNNs on a dataset obtained for typical single- and dual-beam beamforming scenarios. After training, the FFNNs perform requested beamforming tasks within a fraction of second and with about the same accuracy as the original optimization algorithm. The proposed methodology may find applications in future mobile telecommunication systems that require real-time beamforming on low-end hardware. The same beamforming methodology can be also employed in short-range wireless power transfer systems.
更多查看译文
关键词
metasurface,Fourier transform-based beamforming,optimization,neural networks
AI 理解论文
溯源树
样例
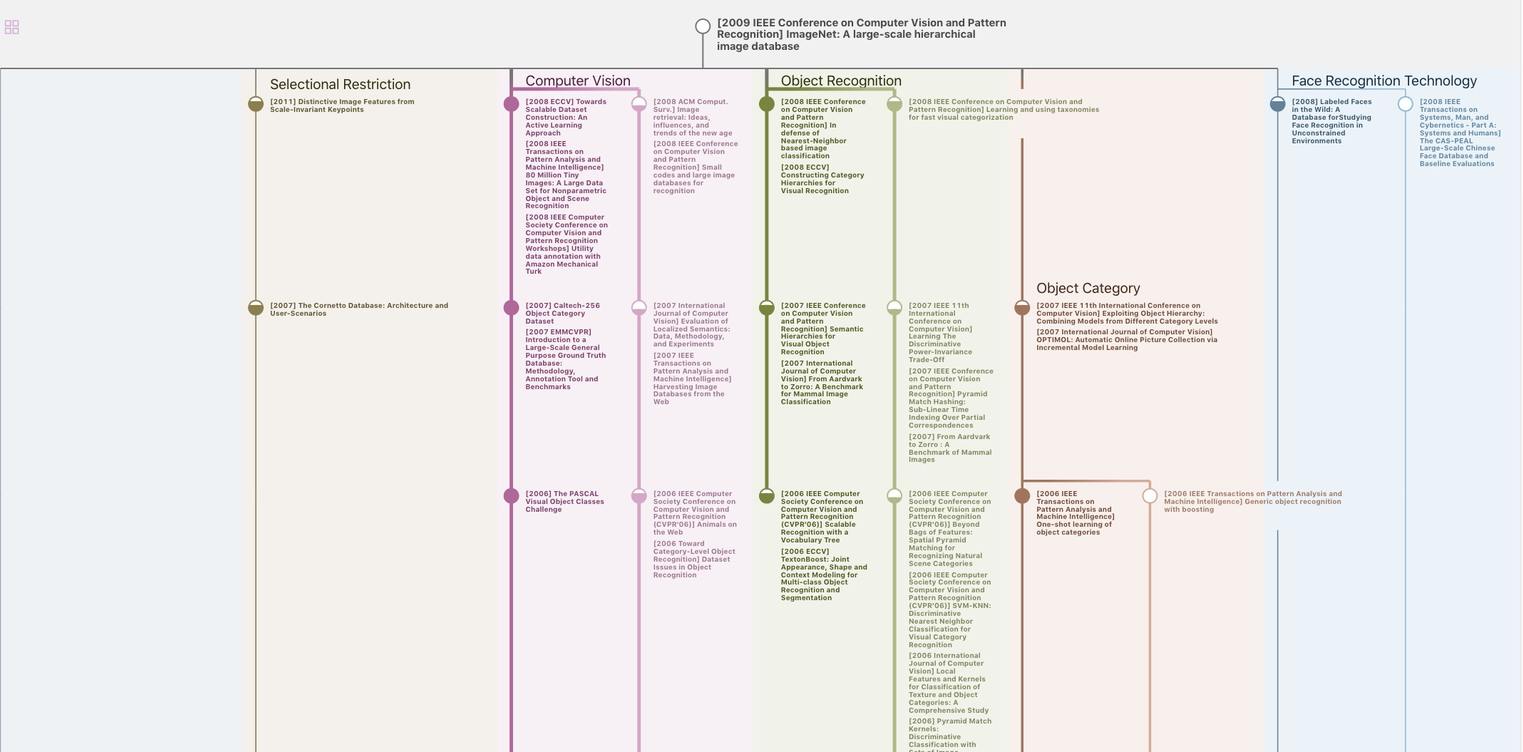
生成溯源树,研究论文发展脉络
Chat Paper
正在生成论文摘要