A sequence to sequence prediction model for remaining useful life of lithium-ion batteries with Bayesian optimisation process visualization
Journal of Energy Storage(2024)
Abstract
Accurate battery remaining useful life (RUL) prediction plays an important role in ensuring reliable operation of electric vehicles. In this paper, a hybrid model based on Bayesian optimization of deep convolutional neural network and long short-term memory neural network (BO-DCNN-LSTM) is proposed for battery RUL prediction. Feature extraction of raw charging characteristic curves is performed by the multilayer CNN and preliminary capacity prediction is performed by the multilayer LSTM. The model performance is explored with different training, validation and testing strategies and different prediction starting points. Validation using NASA battery aging data shows that the mean absolute error (MAE) and root mean square error (RMSE) of the RUL prediction are 0.0139 Ah and 0.0195 Ah, respectively, when the prediction starting point is the 50th cycle. In addition, this paper visualizes the process of how the Bayesian optimization (BO) algorithm searches for the global optimal combinations in the high-dimensional hyperparameter space and discusses the impact of these hyperparameters on the prediction, filling the gap in this part of the research.
MoreTranslated text
Key words
Lithium-ion battery,Remaining useful life prediction,Bayesian optimization,Convolutional neural network,Long and short-term memory neural network
AI Read Science
Must-Reading Tree
Example
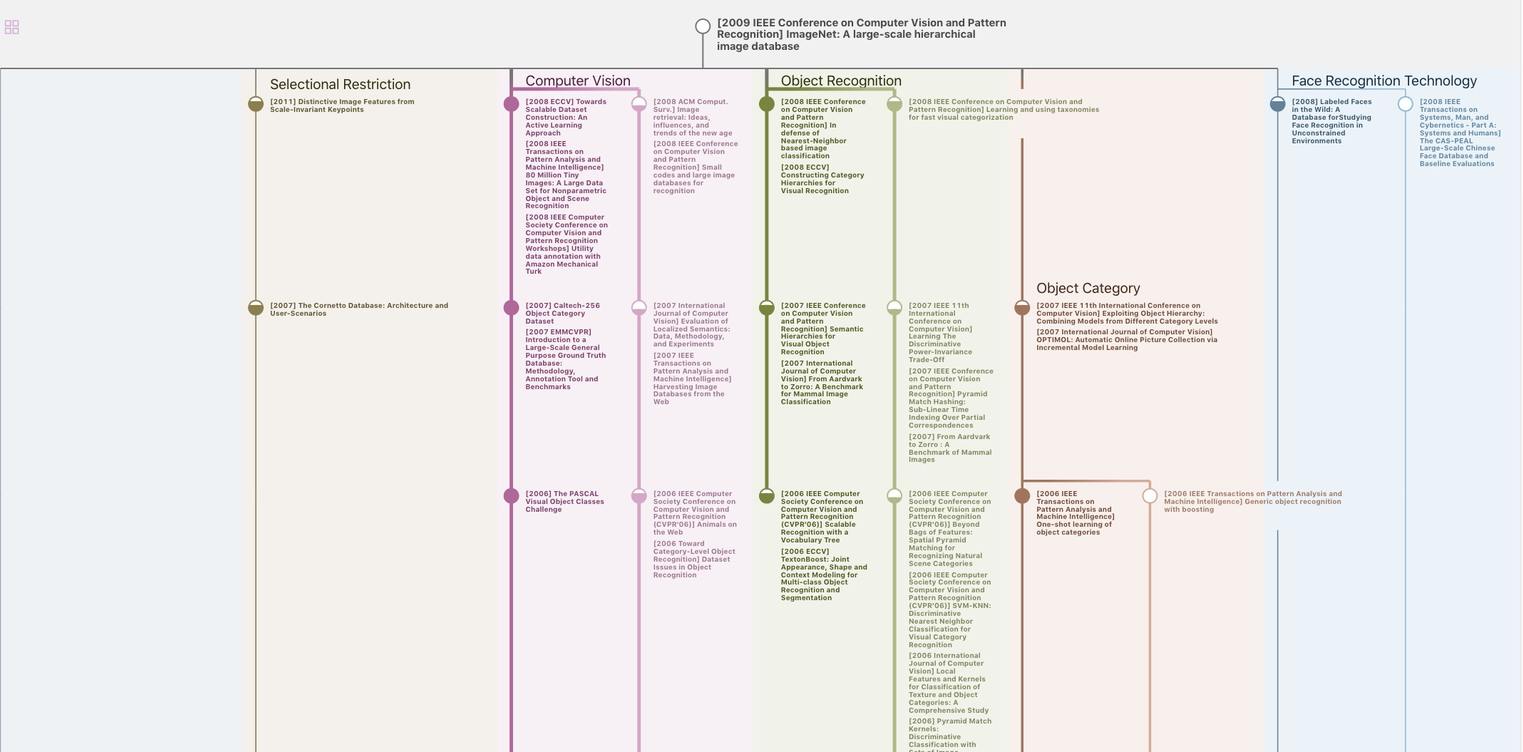
Generate MRT to find the research sequence of this paper
Chat Paper
Summary is being generated by the instructions you defined