Spatiotemporal Correlation Analysis for Predicting Current Transformer Errors in Smart Grids
ENERGIES(2024)
摘要
The online calibration method for current transformers is an important research direction in the field of smart grids. This article constructs a transformer error prediction model based on spatiotemporal integration. This model draws inspiration from the structure of forgetting gates in gated loop units and combines it with a graph convolutional network (GCN) that is good at capturing the spatial relationships within the graph attention network to construct an adaptive GCN. The spatial module formed by this adaptive GCN is used to model the spatial relationships in the circuit network, and the attention mechanism and gated time convolutional network are combined to form a time module to learn the temporal relationships in the circuit network. The layer that combines the time and space modules is used, which consists of a gating mechanism for spatiotemporal fusion, and a transformer error prediction model based on a spatiotemporal correlation analysis is constructed. Finally, it is verified on a real power grid operation dataset, and compared with the existing prediction methods to analyze its performance.
更多查看译文
关键词
transformer error prediction,graph convolutional neural network,graph attention network,gating mechanism
AI 理解论文
溯源树
样例
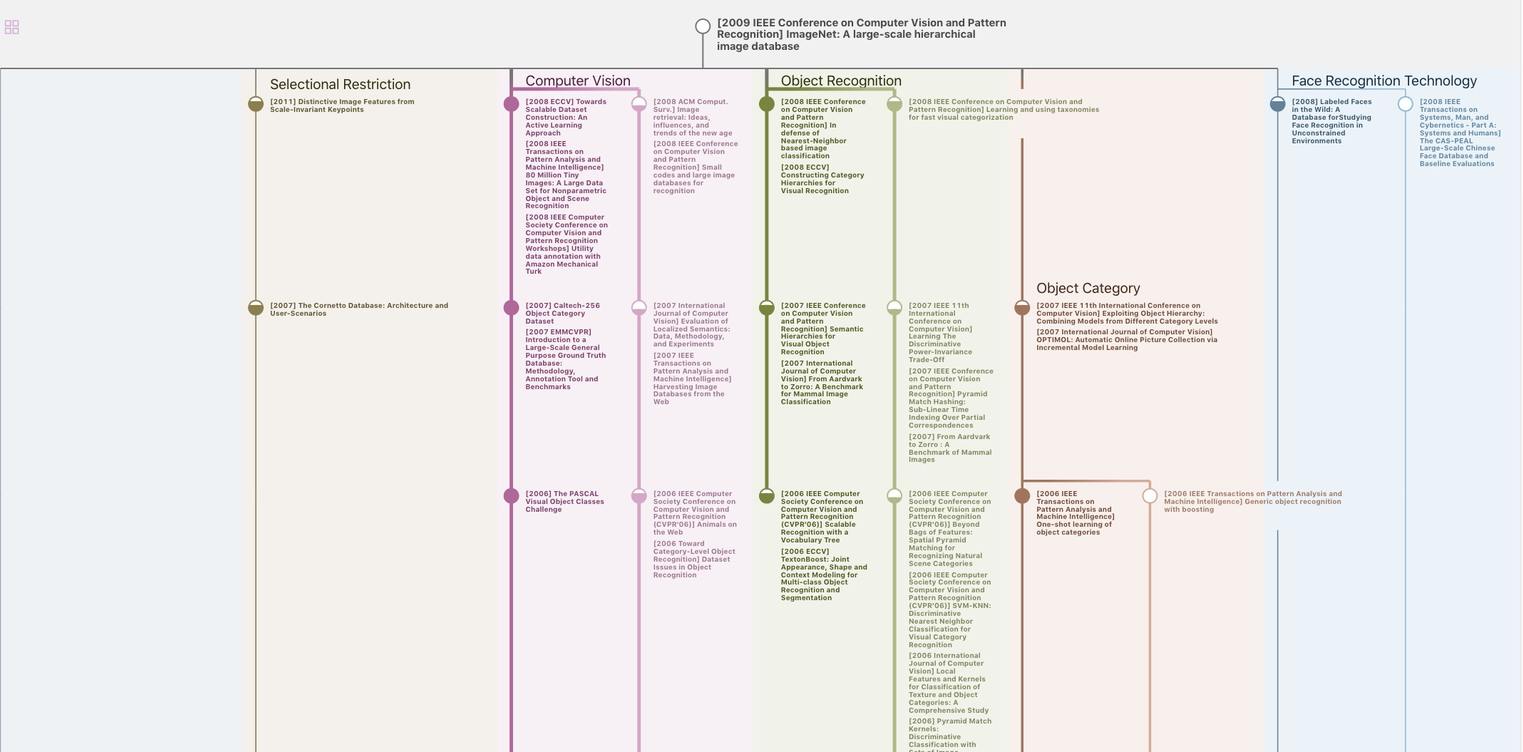
生成溯源树,研究论文发展脉络
Chat Paper
正在生成论文摘要