Enhancing Anomaly Detection for Cultural Heritage via Long Short-Term Memory with Attention Mechanism
ELECTRONICS(2024)
摘要
Cultural heritages are invaluable and non-renewable resources. Existing warning mechanisms usually rely on degradation analysis to assess environmental risk factors. However, they have limitations such as complex research, poor generalization, and inadequate warnings. To address these issues, we propose a hybrid model that combines the long short-term memory network (LSTM) and attention mechanisms with environmental factors to detect anomalies in cultural heritage. The attention mechanism extracts temporal dependencies, while LSTM captures global long-term patterns, enhancing the reconstruction capability. Additionally, we introduce the seasonal, trend, shapelet, and mixed anomalies to enrich the positive and negative samples and propose a novel threshold extraction method to reduce the reliance on prior knowledge. Extensive experiments demonstrate that LSTM-Attention outperforms previous methods, with a root mean square error (RMSE) of 34.328, mean absolute error (MAE) of 27.060, and the largest area under the receiver operating characteristic curve (AUC) value, highlighting the superiority and effectiveness of our method. The ablation study proves the contribution of the attention mechanism, and the reconstruction step can be flexibly adjusted according to practical needs. Finally, the algorithm has been successfully implemented in engineering and employed in practice, providing valuable guidelines for the preservation of ancient buildings worldwide.
更多查看译文
关键词
cultural heritage,anomaly warning,LSTM,attention mechanism,timber structure
AI 理解论文
溯源树
样例
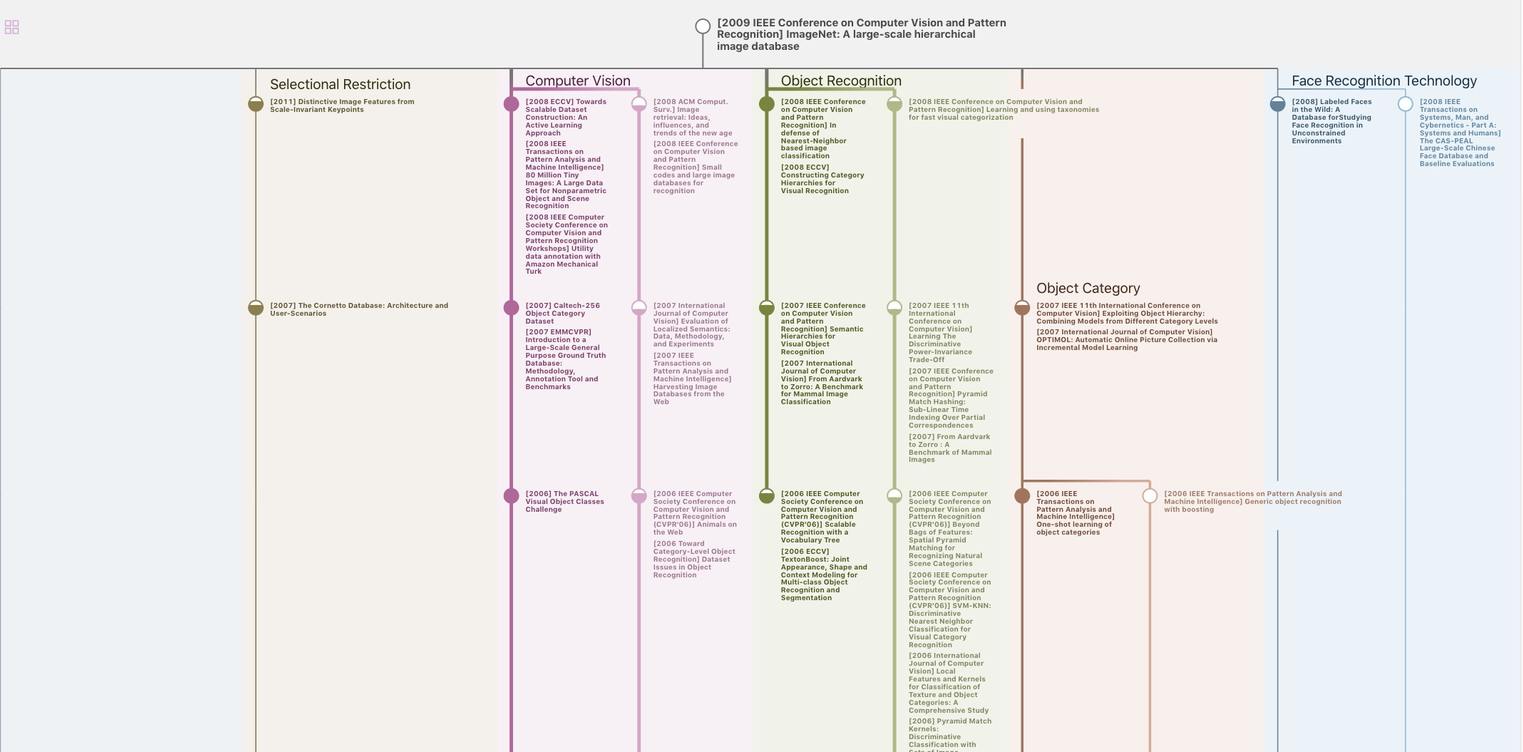
生成溯源树,研究论文发展脉络
Chat Paper
正在生成论文摘要