Knowledge-Based Effective Dispatch for Job Shop Scheduling
JOURNAL OF CIRCUITS SYSTEMS AND COMPUTERS(2024)
Abstract
Although Deep Reinforcement Learning (DRL) is promising in solving Job Shop Scheduling Problems (JSPs), existing DRL-based methods still have large optimality gaps when learning job-to-machine solutions. This is mainly because: (i) existing state representations autonomously learned from graph-structured data cannot fully capture node information to support agents in making optimal decisions; and (ii) existing reward functions cannot accurately reflect some actions that will seriously worsen the current state. Aiming to address these issues, we propose a knowledge-based DRL method that selects nine well-known priority dispatching rules (PDRs) as state features, which can achieve effective model training. To avoid feature over-redundancy, we discard significantly correlated features based on the Pearson correlation relationship analysis, which can help to identify the key factors that affect the agents' decision-making. Furthermore, since it is difficult to design a reward function that can accurately distinguish actions, we mask poor-performing actions based on problem-specific knowledge to prevent them from being selected at the current decision point. Comprehensive experimental results demonstrate the superiority of our approach over four PDRs and four state-of-the-art methods on various benchmarks.
MoreTranslated text
Key words
Job shop scheduling,priority knowledge,Pearson correlation relationship analysis,feature selection,action masking,deep reinforcement learning
AI Read Science
Must-Reading Tree
Example
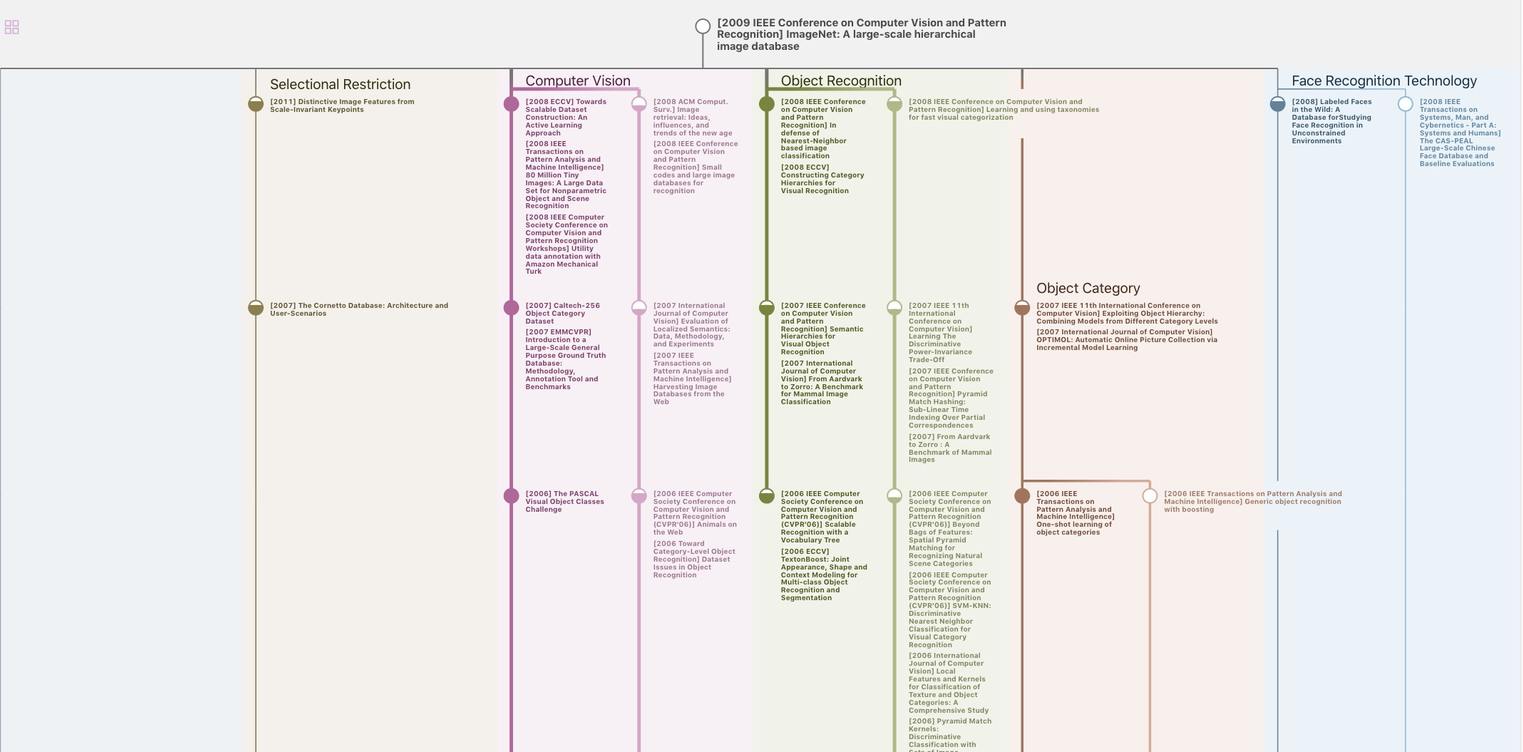
Generate MRT to find the research sequence of this paper
Chat Paper
Summary is being generated by the instructions you defined