A Simple Neural Network for Nonlinear Self-Interference Cancellation in Full-Duplex Radios
IEEE Transactions on Vehicular Technology(2024)
摘要
In-band full-duplex (FD) technology has emerged as one of the most promising solutions for spectrum scarcity, allowing users to transmit and receive simultaneously at the same center frequency. However, nonlinear self-interference (SI) introduced by nonideal transceiver components is considered as the main challenge in FD systems. Traditionally, nonlinear SI is characterized by polynomial models, which are computationally expensive. In this letter, a novel neural network (NN) structure, refered to as the convolutional NN with Mish activation function based on knowledge distillation (KD-MCNN), as well as three practical methods for NN design and training are proposed to model nonlinear SI with lower computational complexity. To accommodate hardware with varying computational capabilities, nine KD-MCNN models with different scales are provided. The numerical simulations show that KD-MCNN cancellers exhibit superior performance and computational efficiency compared to both polynomial-based cancellers and existing NN-based cancellers. In particular, the nonlinear SI power can be further reduced by approximately 7.70 dB via the KD-MCNN teacher canceller, which is only 2.48 dB above the receiver's noise floor.
更多查看译文
关键词
In-band full-duplex systems,nonlinear self interference,knowledge distillation,convolutional neural networks
AI 理解论文
溯源树
样例
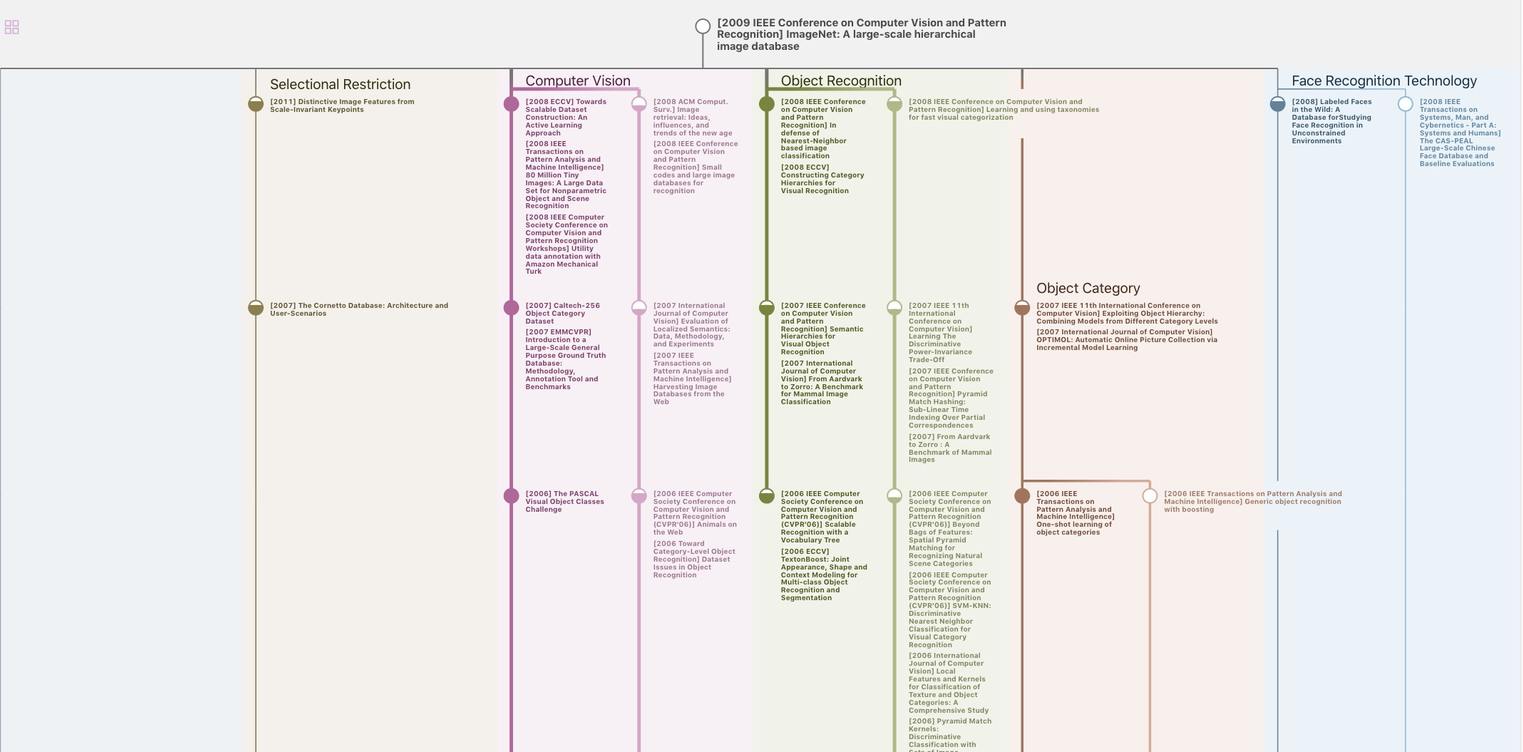
生成溯源树,研究论文发展脉络
Chat Paper
正在生成论文摘要