A Data-Efficient Deep Learning Method for Rough Surface Clutter Reduction in GPR Images
IEEE TRANSACTIONS ON GEOSCIENCE AND REMOTE SENSING(2024)
摘要
In ground penetrating radar (GPR) B-scan images, ground surface clutter is the main source of interference, often obscuring or distorting subsurface target signals. We propose a deep autoencoder-based method to mitigate rough surface clutter in GPR images by treating it as an anomaly detection problem. First, the rough surface region in a B-scan image is partitioned into small patches, which act as the training dataset for the deep autoencoder. Through the training process, the autoencoder learns and captures the patterns associated with the rough surface patches. Following training, the entire B-scan image is divided into small patches of the same size as the training patches, and each of them is fed into the autoencoder to compute an anomaly score. To reconstruct a clutter-reduced B-scan image, we employ a weighted sum approach to aggregate all patches based on their anomaly scores. We evaluate our method against conventional subspace projection techniques using simulated and field-collected B-scans. The results clearly indicate that our approach surpasses these subspace methods. Furthermore, we employ t-distributed stochastic neighbor embedding (t-SNE) analysis to gain deeper insights into our method's effectiveness in reducing rough surface clutter. The outcomes of this analysis reinforce the practical viability of our approach for GPR image processing.
更多查看译文
关键词
Clutter,Surface roughness,Rough surfaces,Training,Surface reconstruction,Surface treatment,Image reconstruction,Autoencoder,data efficient,deep learning (DL),ground penetrating radar (GPR),rough surface clutter
AI 理解论文
溯源树
样例
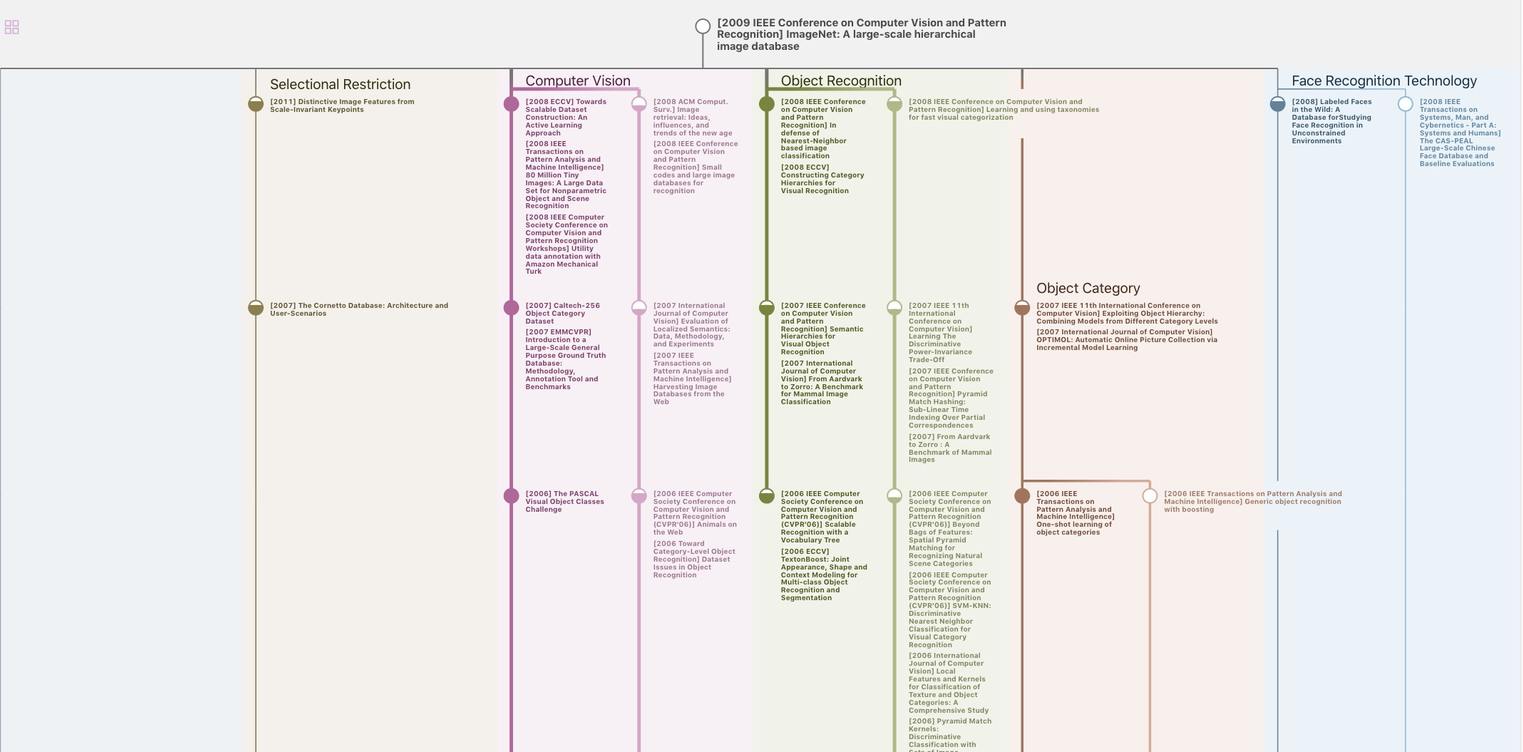
生成溯源树,研究论文发展脉络
Chat Paper
正在生成论文摘要