A Traffic Flow Data Restoration Method Based on an Auxiliary Discrimination Mechanism-Oriented GAN Model
IEEE Internet of Things Journal(2024)
摘要
High-quality traffic flow data is foundational to the study of traffic issues and practical engineering applications. The development of traffic flow detection methods has greatly facilitated the collection of traffic data; however, anomalies caused by factors such as equipment, networks, and environmental conditions present a common challenge. Traditional data restoration methods, which require extensive complete historical data as prior knowledge, are difficult to implement. In this study, we first analyze and categorize the causes of anomalous data, and introduce strategies for anomaly identification in multi-source freeway data. Based on the unsupervised learning framework of Generative Adversarial Network (GAN), and leveraging their advantages in data generation, we propose an Auxiliary Discrimination Mechanism-based Generative Adversarial Network (ADM-GAN) for the task of traffic flow data restoration. The optimization of the generator and discriminator and the auxiliary discrimination matrix, constructed from the generator’s output and the original data features, enhances the model’s utilization of original data. We tested our model using ETC traffic flow data from the G50 freeway near Suzhou, China. The results demonstrate that ADM-GAN outperforms other state-of-the-art methods in data restoration tasks across various data missing rates. This research offers an efficient and reliable method for freeway traffic data restoration.
更多查看译文
关键词
Traffic engineering,traffic flow,data restoration,Generative Adversarial Network
AI 理解论文
溯源树
样例
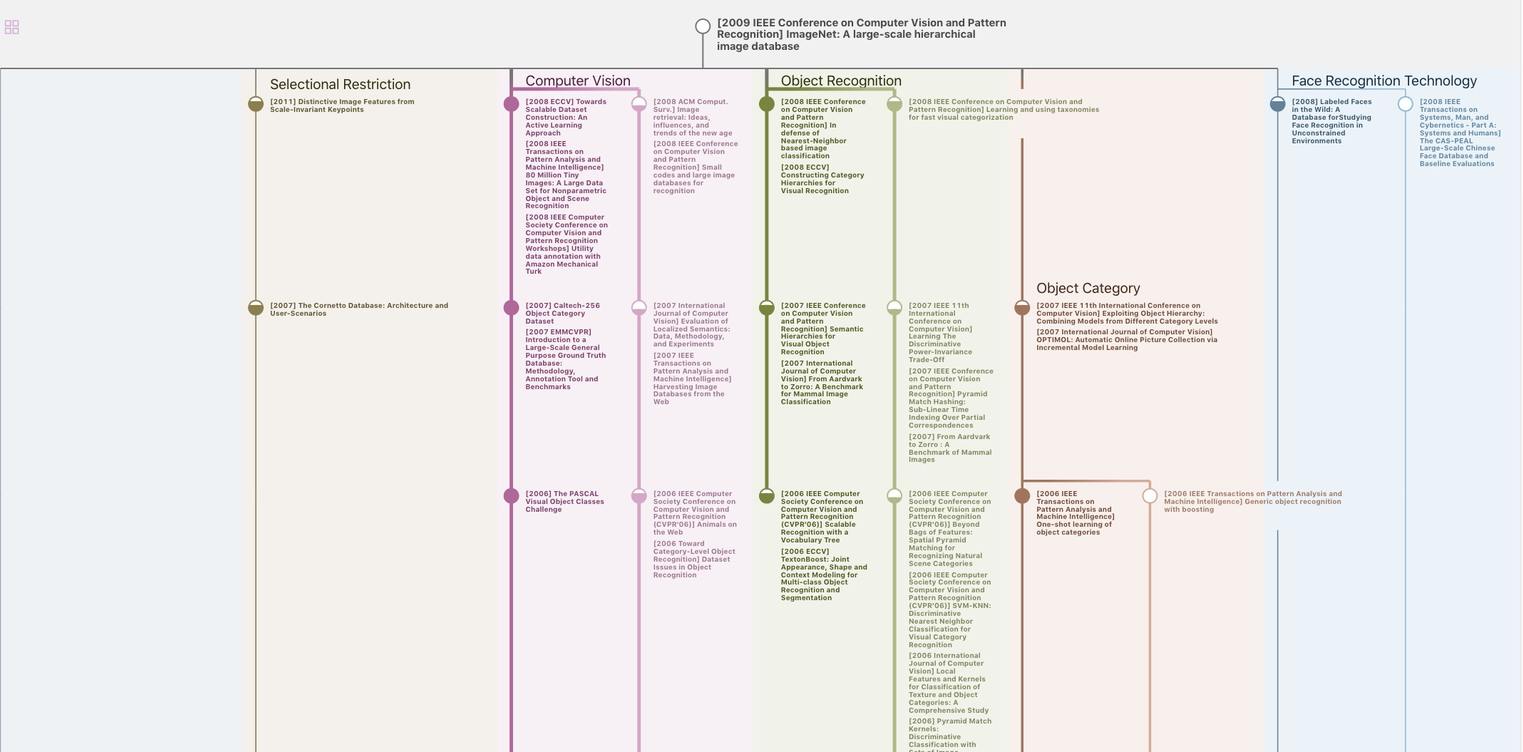
生成溯源树,研究论文发展脉络
Chat Paper
正在生成论文摘要