Predicting A/B compartments from histone modifications using deep learning
iScience(2024)
摘要
The three-dimensional organization of genomes plays a crucial role in essential biological processes. The segregation of chromatin into A and B compartments highlights regions of activity and inactivity, providing a window into the genomic activities specific to each cell type. Yet, the steep costs associated with acquiring Hi-C data, necessary for studying this compartmentalization across various cell types, pose a significant barrier in studying cell type specific genome organization. To addres this, we present a prediction tool called Compartment prediction using Recurrent Neural Networks (CoRNN), which predicts compertmantalization of 3D genome using histone modification enrichment. CoRNN demonstrates robust cross-cell type prediction of A/B compartments with an average AuROC of 90.9%. Cell type-specific predictions align well with known functional elements, with H3K27ac and H3K36me3 identified as highly predictive histone marks. We further investigate our mispredictions and found that they are located in regions with ambiguous compartmental status. Furthermore, our model’s generalizability is validated by predicting compartments in independent tissue samples, which underscores its broad applicability.
更多查看译文
AI 理解论文
溯源树
样例
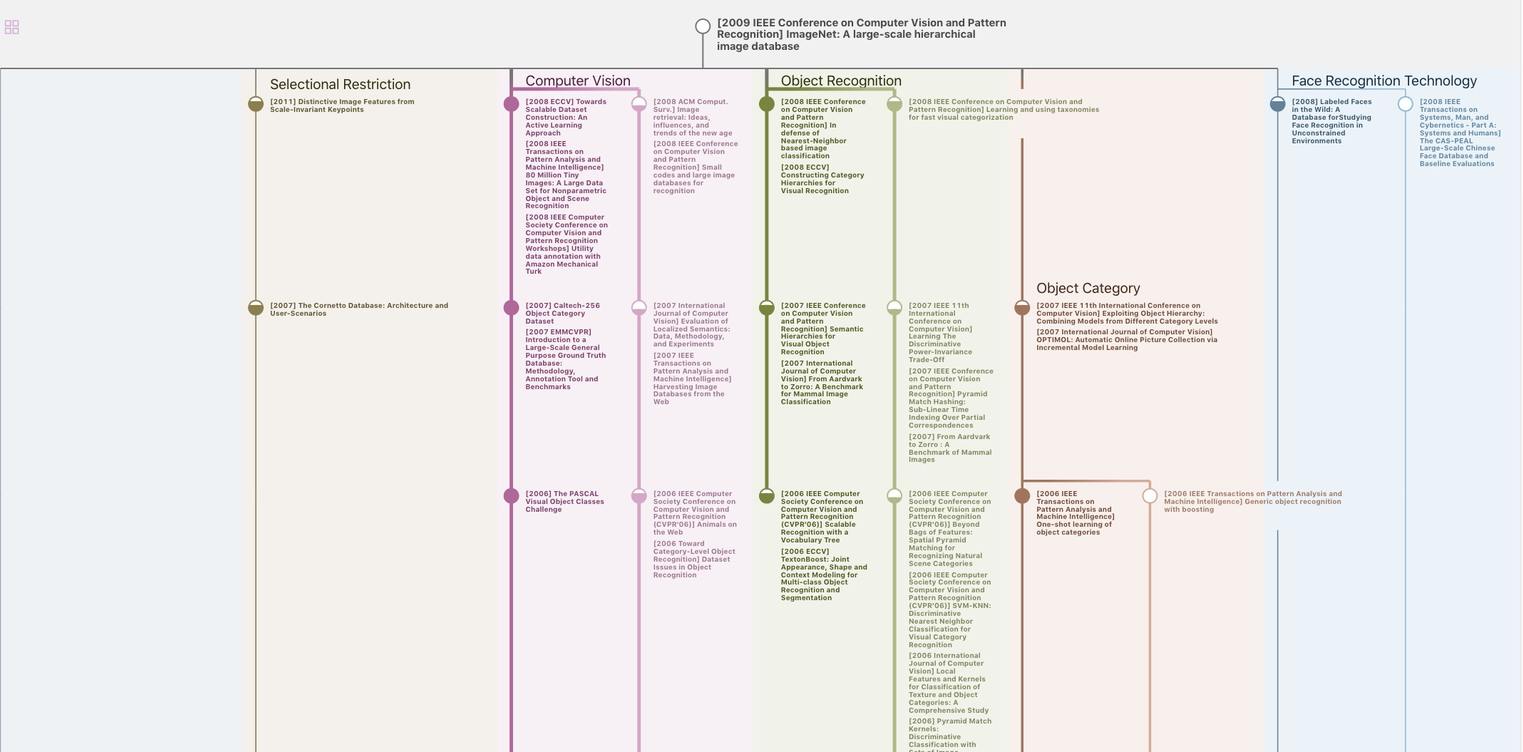
生成溯源树,研究论文发展脉络
Chat Paper
正在生成论文摘要