Mix Convolutional Neural Networks for Hyperspectral Wheat Variety Discrimination
SPECTROSCOPY AND SPECTRAL ANALYSIS(2024)
Abstract
Different varieties of wheat meet the market's diverse needs, but it also brings the risk of mixed wheat varieties. To improve the purity of wheat varieties and thus the economic value of the selection, breeding, and processing, the identification of wheat seeds plays a key role. Traditional methods of physical and chemical analysis of wheat variety purity take a long time to identify and destroy seeds, which can no longer meet the urgent needs of modern agriculture. Hyperspectral imaging is a new, fast, efficient, and nondestructive identification technique that has achieved remarkable results in seed variety identification. Unfortunately, most existing methods use only spectral information without considering spatial information sufficiently to produce unsatisfactory classification results. A hyperspectral imaging device was used to acquire hyperspectral images of the front and back of wheat seeds of eight varieties in the paper. Meanwhile, we propose a hyperspectral wheat variety identification method based on a hybrid convolutional neural network with an attention mechanism, which mainly uses the advantageous complementary features of 3D convolution and 2D convolution to extract valuable features of wheat for driving the identification of wheat varieties. Precisely, we first extract the regions of interest of wheat varieties and use multiple scattering correction methods to weaken the spectral differences of the same variety due to differences in scattering levels. Meanwhile, we use principal component analysis to reduce the useless spectral bands of 3D data and thus retain and compress the valuable features for identifying wheat varieties. Subsequently, a 3D convolution module acquires spatial dimension and feature information between different spectra, a 2D convolution module is used to obtain spatial features and the image's inherent feature information, and an attention mechanism is introduced into the 2D convolution model to refine the features. Finally, the identification of different wheat varieties in the same region is achieved at the fully connected layer. Extensive experiments on our collected dataset show that the proposed method performs better than the state-of-the-art methods, and its classification accuracy reaches 97. 92%. Besides, the proposed method has better classification performance for a small sample. In short, the proposed method has good effectiveness and robustness for hyperspectral wheat seed identification and provides a new method for the online identification of wheat seeds.
MoreTranslated text
Key words
Hyperspectral imaging,Wheat varieties,Attention mechanism,Mixed convolution
AI Read Science
Must-Reading Tree
Example
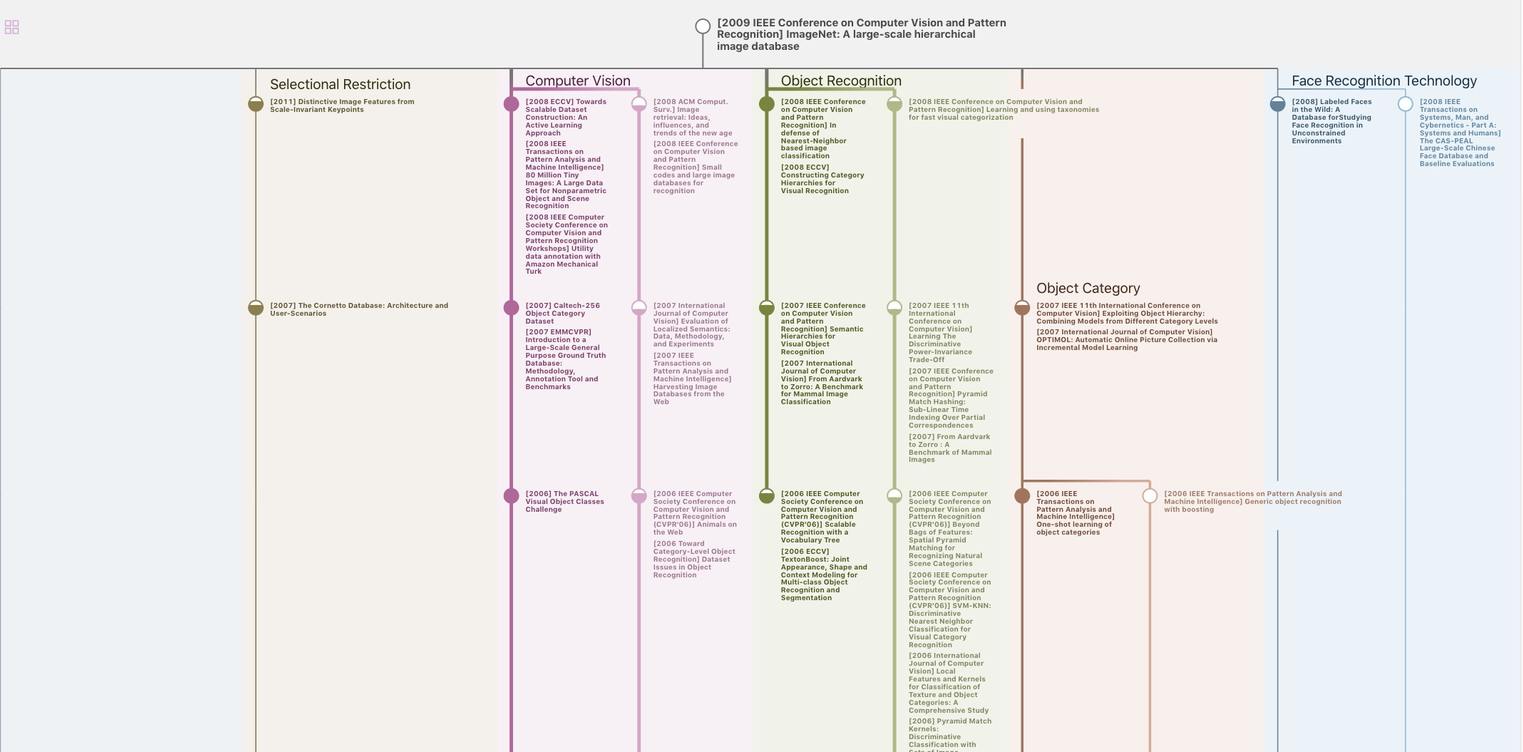
Generate MRT to find the research sequence of this paper
Chat Paper
Summary is being generated by the instructions you defined