Study on Coal and Gangue Recognition by Vis-NIR Spectroscopy Under Different Working Conditions
SPECTROSCOPY AND SPECTRAL ANALYSIS(2024)
摘要
In the process of realizing the efficient utilization of coal, coal and gangue separation is a very important step, but the existing coal and gangue separation technology has the problems of resource waste and low efficiency. It can be seen that Vis-NIR(visible near-infrared) spectroscopy identification technology has the advantages of being fast and reliable and has a certain research foundation in the field of coal and gangue recognition, but most of the studies have not been effectively analyzed in combination with actual conditions. Firstly, this paper set up a Vis-NIR spectrum acquisition device in the laboratory to simulate three conditions in the actual environment: different detection angles (0 degrees, 10 degrees, 20 degrees, 30 degrees), different detection distances (10, 15, 20 and 25 cm), and different illumination angles (15 degrees, 25 degrees, 35 degrees, 45 degrees). The spectral data of coal and gangue samples from Ximing Coal Mine in Shanxi are collected in the Vis-NIR spectrum band under the single-factorandmulti-factor conditions of orthogonal experimental design. Secondly, the collected spectral data were analyzed and successively underwent standard normal variable transformation and Savitzky-Golay convolution smoothing to reduce the impact of noise and error on the data. Finally, in the single factor experiment, the spectral data were trained based on five machine learning models, including decision tree (DT), k-nearest neighbor (KNN), partial least squares discriminant analysis (PLS-DA), support vector machine (SVM) and AdaBoost, combined with the preprocessing algorithm. The results of the single factor experiment show that the AdaBoost algorithm adopted in this paper has strong learning ability, and the recognition accuracy of coal and gangue under different working conditions is 100%, which is better than other recognition models. In orthogonal experiments, a support vector machine (SVM) is used as the recognition model for training. The results show that, in the rawand preprocessed data, the three conditions have different degrees of influence on the recognition accuracy of coal and gangue, and the order of influence from large to small is different illumination angles, different detection distances and different detection angles. In the preprocessed data, the optimal combination of working conditions is a detection angle of 0 degrees, detection distance of 20 cm and illumination angle of 35 degrees. At the same time, by comparing the experimental results, it can be found that the appropriate pretreatment and modeling methods can reduce the influence of different working conditions on recognition accuracy. A group of conditions were randomly selected to perform three repeated control trials with the optimal group. The results showed that the optimal group had better recognition performance than the random control group. The research results have reference significance for finding the optimal working conditions of coal and gangue identification and provide a theoretical basis for the practical application of Vis-NIR spectroscopy in coal and gangue identification.
更多查看译文
关键词
Vis-NIR spectroscopy,Different working conditions,Coal and gangue identification,AdaBoost,Orthogonal experiment
AI 理解论文
溯源树
样例
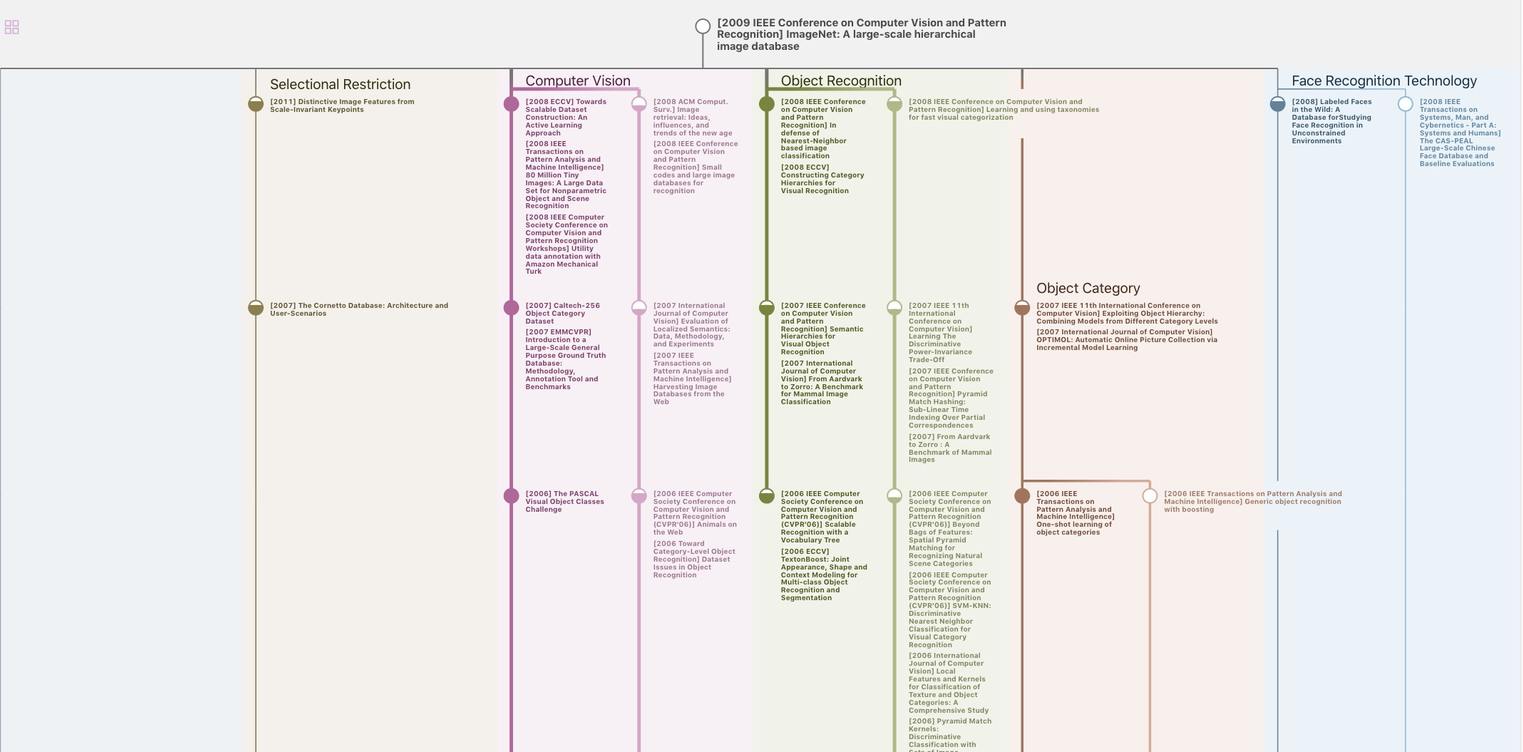
生成溯源树,研究论文发展脉络
Chat Paper
正在生成论文摘要