Top-k Classification and Cardinality-Aware Prediction
CoRR(2024)
摘要
We present a detailed study of top-k classification, the task of predicting
the k most probable classes for an input, extending beyond single-class
prediction. We demonstrate that several prevalent surrogate loss functions in
multi-class classification, such as comp-sum and constrained losses, are
supported by H-consistency bounds with respect to the top-k loss. These
bounds guarantee consistency in relation to the hypothesis set H, providing
stronger guarantees than Bayes-consistency due to their non-asymptotic and
hypothesis-set specific nature. To address the trade-off between accuracy and
cardinality k, we further introduce cardinality-aware loss functions through
instance-dependent cost-sensitive learning. For these functions, we derive
cost-sensitive comp-sum and constrained surrogate losses, establishing their
H-consistency bounds and Bayes-consistency. Minimizing these losses leads to
new cardinality-aware algorithms for top-k classification. We report the
results of extensive experiments on CIFAR-100, ImageNet, CIFAR-10, and SVHN
datasets demonstrating the effectiveness and benefit of these algorithms.
更多查看译文
AI 理解论文
溯源树
样例
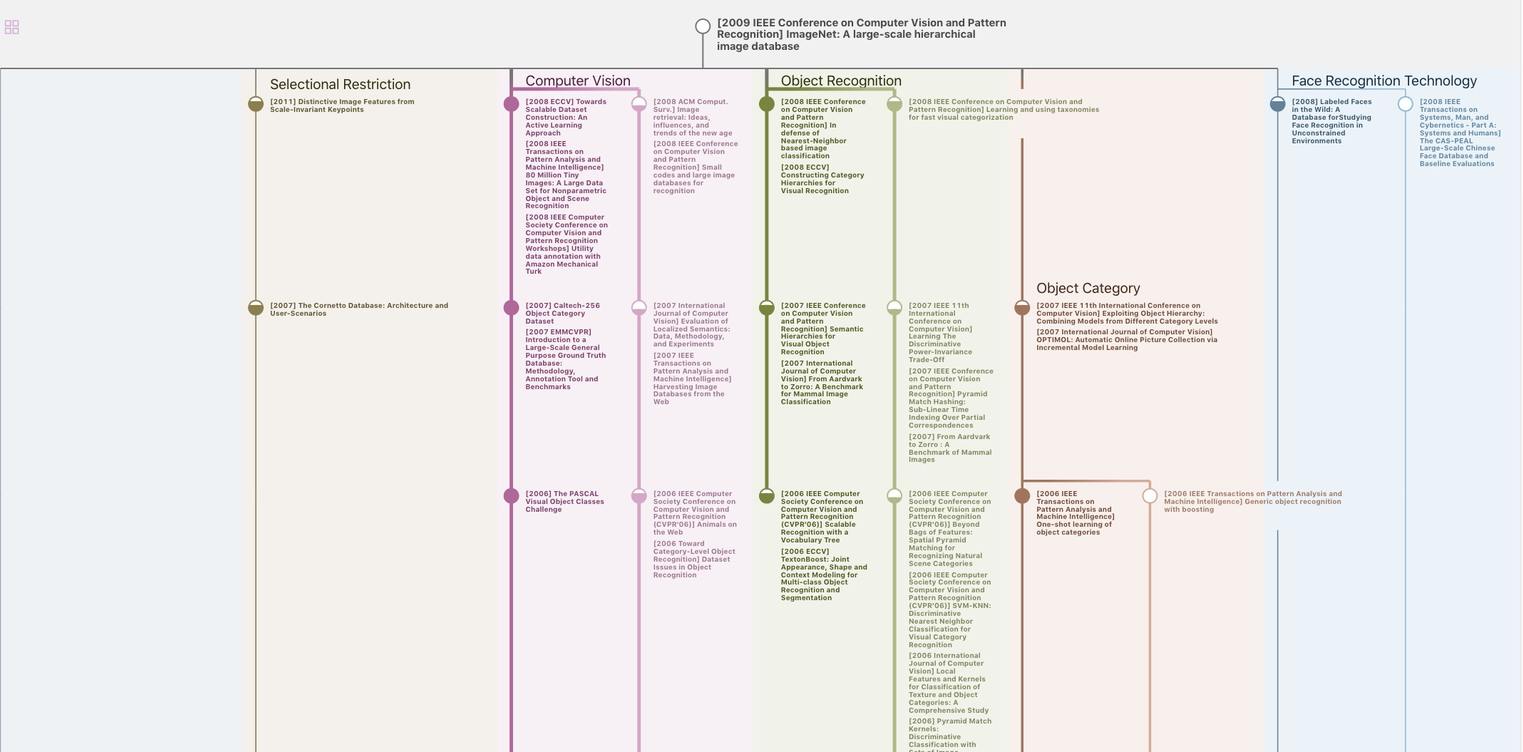
生成溯源树,研究论文发展脉络
Chat Paper
正在生成论文摘要