Fairness in Ranking: Robustness through Randomization without the Protected Attribute
arxiv(2024)
摘要
There has been great interest in fairness in machine learning, especially in
relation to classification problems. In ranking-related problems, such as in
online advertising, recommender systems, and HR automation, much work on
fairness remains to be done. Two complications arise: first, the protected
attribute may not be available in many applications. Second, there are multiple
measures of fairness of rankings, and optimization-based methods utilizing a
single measure of fairness of rankings may produce rankings that are unfair
with respect to other measures. In this work, we propose a randomized method
for post-processing rankings, which do not require the availability of the
protected attribute. In an extensive numerical study, we show the robustness of
our methods with respect to P-Fairness and effectiveness with respect to
Normalized Discounted Cumulative Gain (NDCG) from the baseline ranking,
improving on previously proposed methods.
更多查看译文
AI 理解论文
溯源树
样例
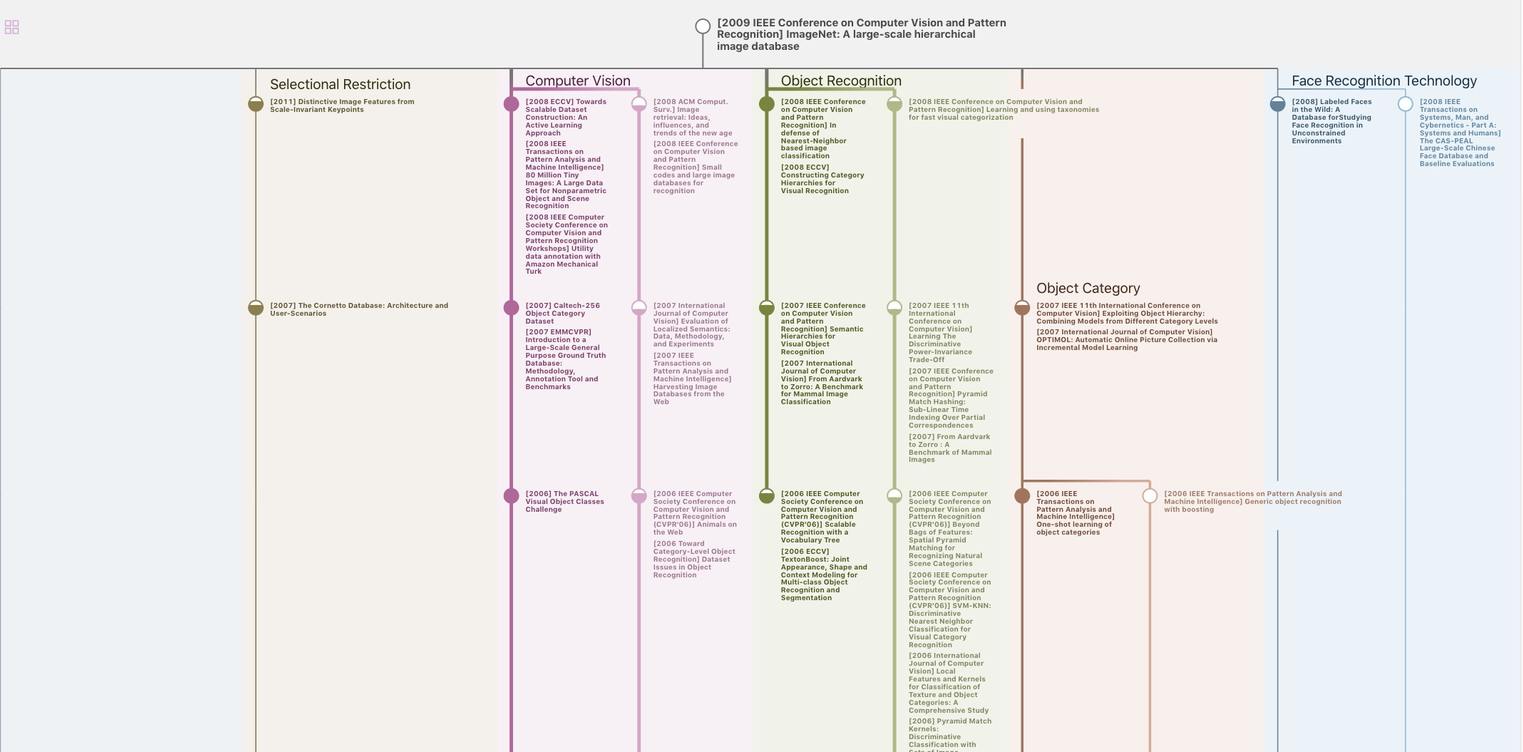
生成溯源树,研究论文发展脉络
Chat Paper
正在生成论文摘要