Sine Activated Low-Rank Matrices for Parameter Efficient Learning
CoRR(2024)
摘要
Low-rank decomposition has emerged as a vital tool for enhancing parameter
efficiency in neural network architectures, gaining traction across diverse
applications in machine learning. These techniques significantly lower the
number of parameters, striking a balance between compactness and performance.
However, a common challenge has been the compromise between parameter
efficiency and the accuracy of the model, where reduced parameters often lead
to diminished accuracy compared to their full-rank counterparts. In this work,
we propose a novel theoretical framework that integrates a sinusoidal function
within the low-rank decomposition process. This approach not only preserves the
benefits of the parameter efficiency characteristic of low-rank methods but
also increases the decomposition's rank, thereby enhancing model accuracy. Our
method proves to be an adaptable enhancement for existing low-rank models, as
evidenced by its successful application in Vision Transformers (ViT), Large
Language Models (LLMs), Neural Radiance Fields (NeRF), and 3D shape modeling.
This demonstrates the wide-ranging potential and efficiency of our proposed
technique.
更多查看译文
AI 理解论文
溯源树
样例
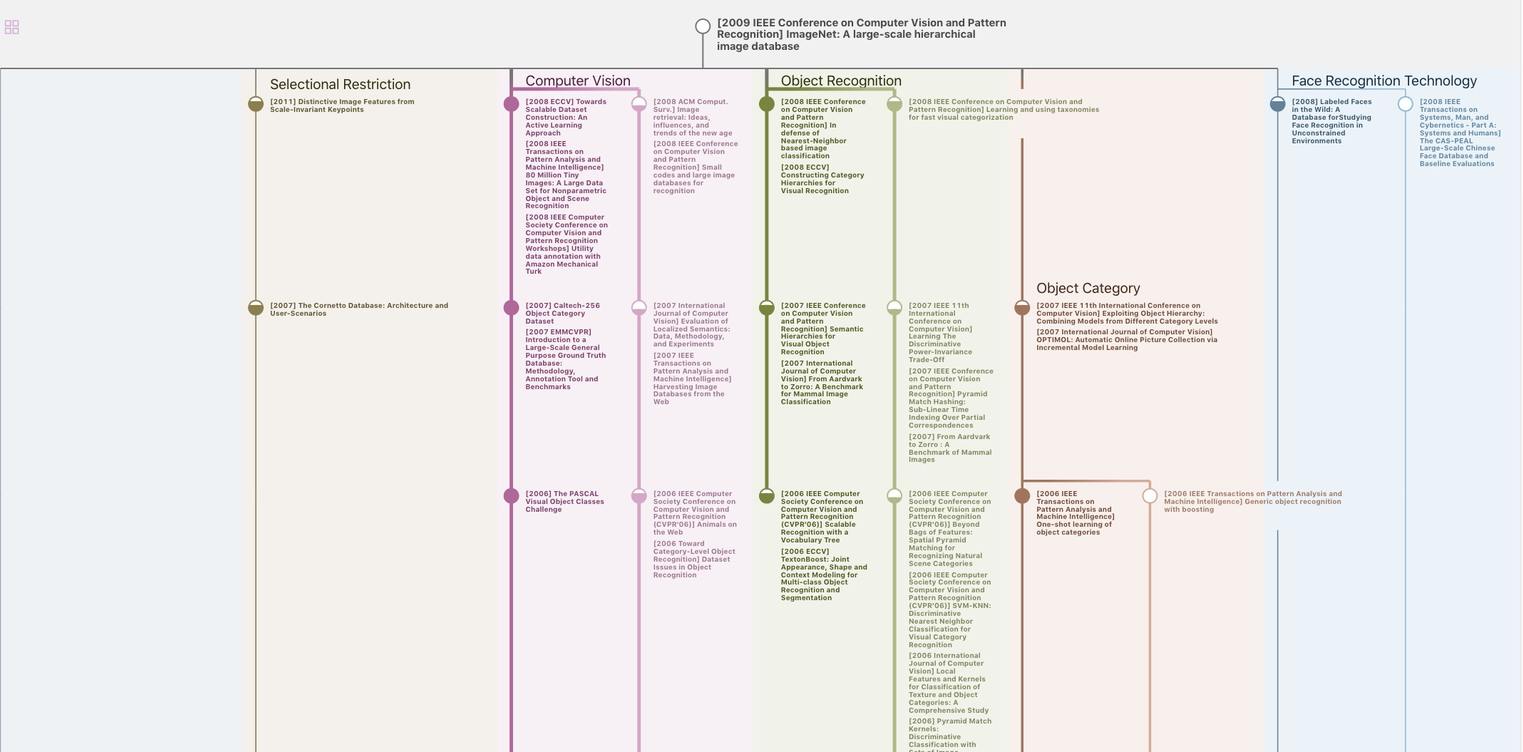
生成溯源树,研究论文发展脉络
Chat Paper
正在生成论文摘要