Imputing missing not-at-random longitudinal marker values in time-to-event analysis: fully conditional specification multiple imputation in joint modeling
arxiv(2024)
AI Read Science
Must-Reading Tree
Example
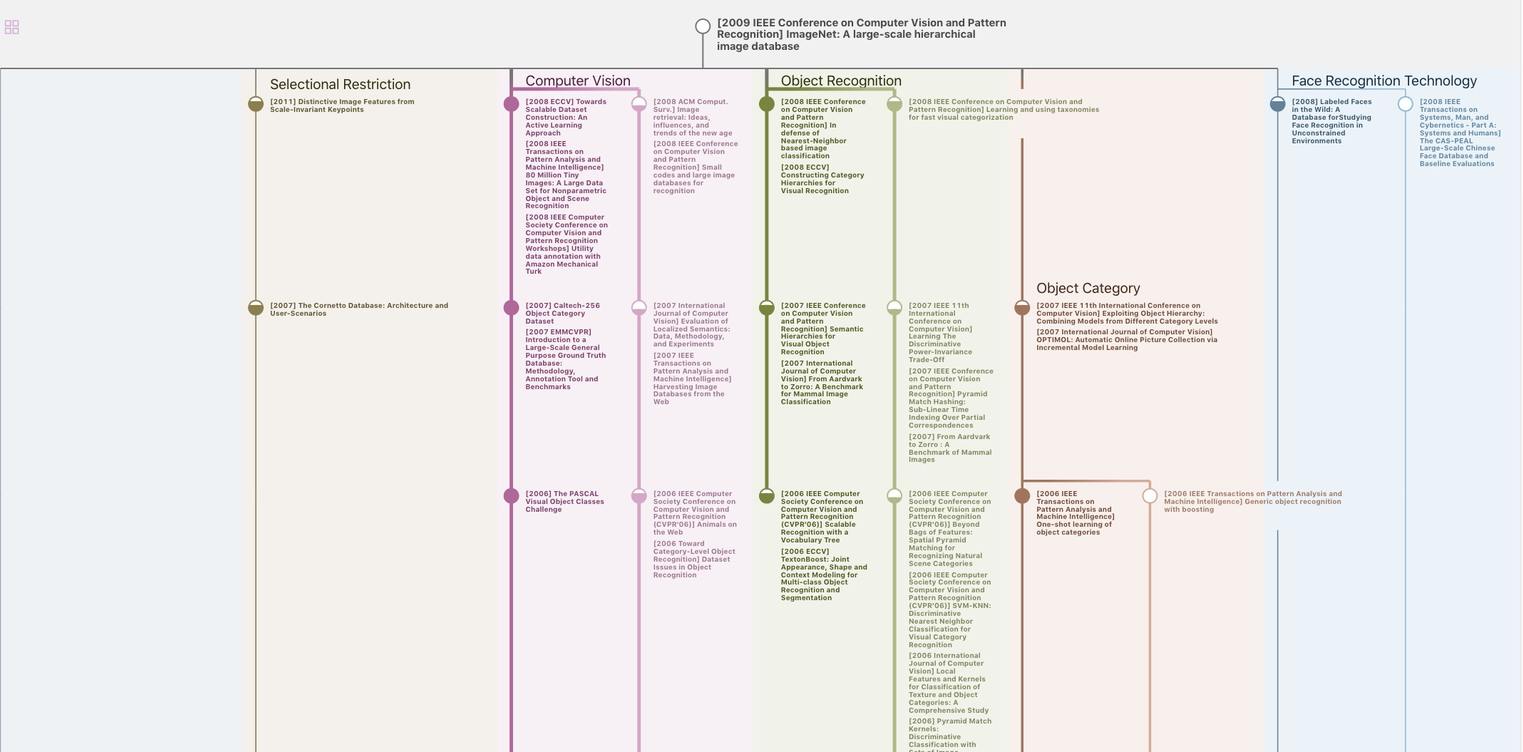
Generate MRT to find the research sequence of this paper
Chat Paper
Summary is being generated by the instructions you defined