A Framework for Time-Varying Optimization Via Derivative Estimation
European Control Conference(2024)
Key words
Optimization Framework,Time-varying Optimization,Time-variant,Optimization Algorithm,Cost Function,Ordinary Differential Equations,Time Derivative,Online Optimization,Optimal Guaranteed,Trajectory Tracking Problem,Gaussian Noise,Finite Difference,Control Theory,Asymptotically Stable,Convex Optimization,Tracking Error,Newton Method,Knowledge Variables,Error Bounds,Gradient Flow,K-function,Optimization Task
AI Read Science
Must-Reading Tree
Example
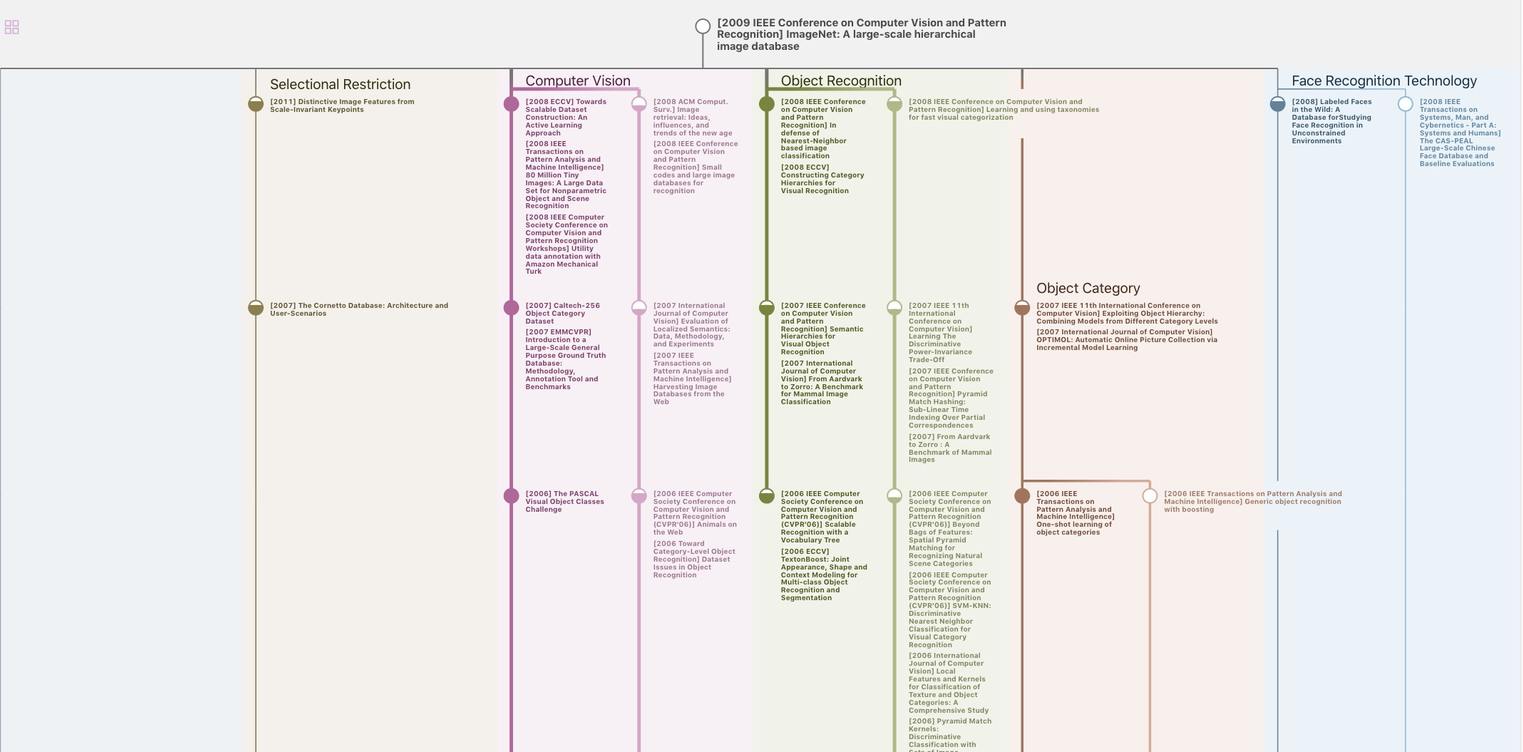
Generate MRT to find the research sequence of this paper
Chat Paper
Summary is being generated by the instructions you defined