A feature-enhanced hybrid attention network for traffic sign recognition in real scenes
IET IMAGE PROCESSING(2024)
摘要
Currently, traffic sign recognition techniques have been brought into the assistive driving of automobiles. However, small traffic sign recognition in real scenes is still a challenging task due to the class imbalance issue and the size limit of the traffic signs. To address the above issues, a feature-enhanced hybrid attention network is proposed based on YOLOv5s for a small, fast, and accurate traffic sign detector. First, a series of online data augmentation strategies are designed in the preprocessing module for the model training. Second, the hybrid channel and spatial attention module CSAM are integrated into the backbone for a better feature extraction ability. Third, the channel attention module CAM is used in the detection head for a more efficient feature fusion ability. To validate the approach, extensive experiments are conducted based on the Tsinghua-Tencent 100K dataset. It is found that the novel method achieves state-of-the-art performance with only negligible increases in the model parameter and computational overhead. Specifically, the mAP@0.5$mAP@0.5$, parameters, and FLOPs are 85.8%, 7.13 M, and 16.1 G, respectively. We have developed a series of effective online data augmentation strategies for the traffic sign recognition dataset, which are able to improve the model performance without any extra computational overhead during the prediction process; To enhance the feature extraction ability of the backbone almost without extra model complexity, we have developed an efficient CSAM module placed at the beginning of the backbone, with the help of the hybrid channel and spatial attention mechanism and the residual bottleneck structure; To make better use of the features extracted by the backbone, we combined the channel attention module CAM with the feature pyramid network (FPN) and path aggregation network (PAN) structure for a multi-scale attention feature fusion detection head. image
更多查看译文
关键词
computer vision,deep learning,object detection,traffic sign recognition
AI 理解论文
溯源树
样例
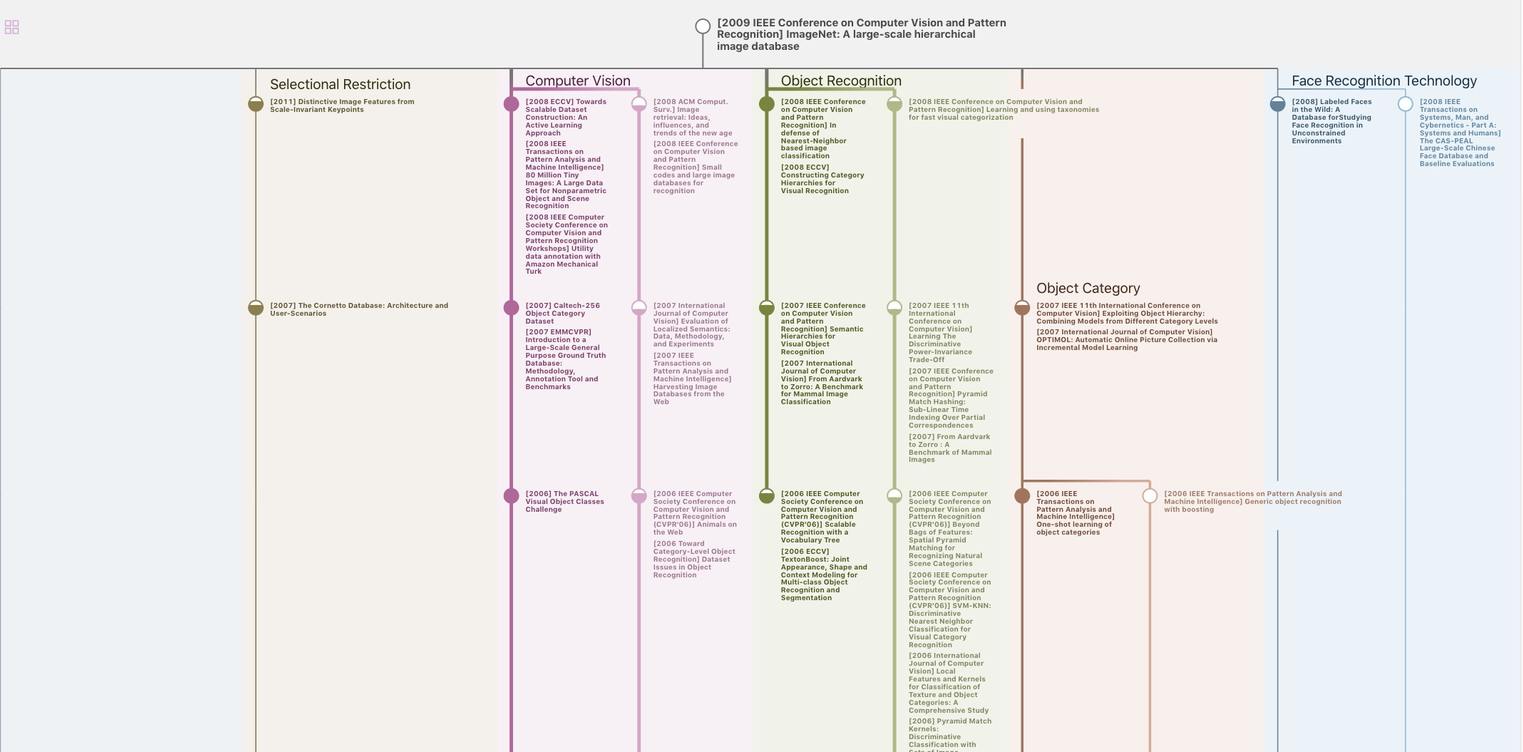
生成溯源树,研究论文发展脉络
Chat Paper
正在生成论文摘要