EM-COGLOAD: An investigation into age and cognitive load detection using eye tracking and deep learning.
Computational and structural biotechnology journal(2024)
摘要
Alzheimer's Disease is the most prevalent neurodegenerative disease, and is a leading cause of disability among the elderly. Eye movement behaviour demonstrates potential as a non-invasive biomarker for Alzheimer's Disease, with changes detectable at an early stage after initial onset. This paper introduces a new publicly available dataset: EM-COGLOAD (available at https://osf.io/zjtdq/, DOI: 10.17605/OSF.IO/ZJTDQ). A dual-task paradigm was used to create effects of declined cognitive performance in 75 healthy adults as they carried out visual tracking tasks. Their eye movement was recorded, and time series classification of the extracted eye movement traces was explored using a range of deep learning techniques. The results of this showed that convolutional neural networks were able to achieve an accuracy of 87.5% when distinguishing between eye movement under low and high cognitive load, and 76% when distinguishing between the oldest and youngest age groups.
更多查看译文
关键词
Time series classification,Eye movement,Deep learning,Cognitive load,Age
AI 理解论文
溯源树
样例
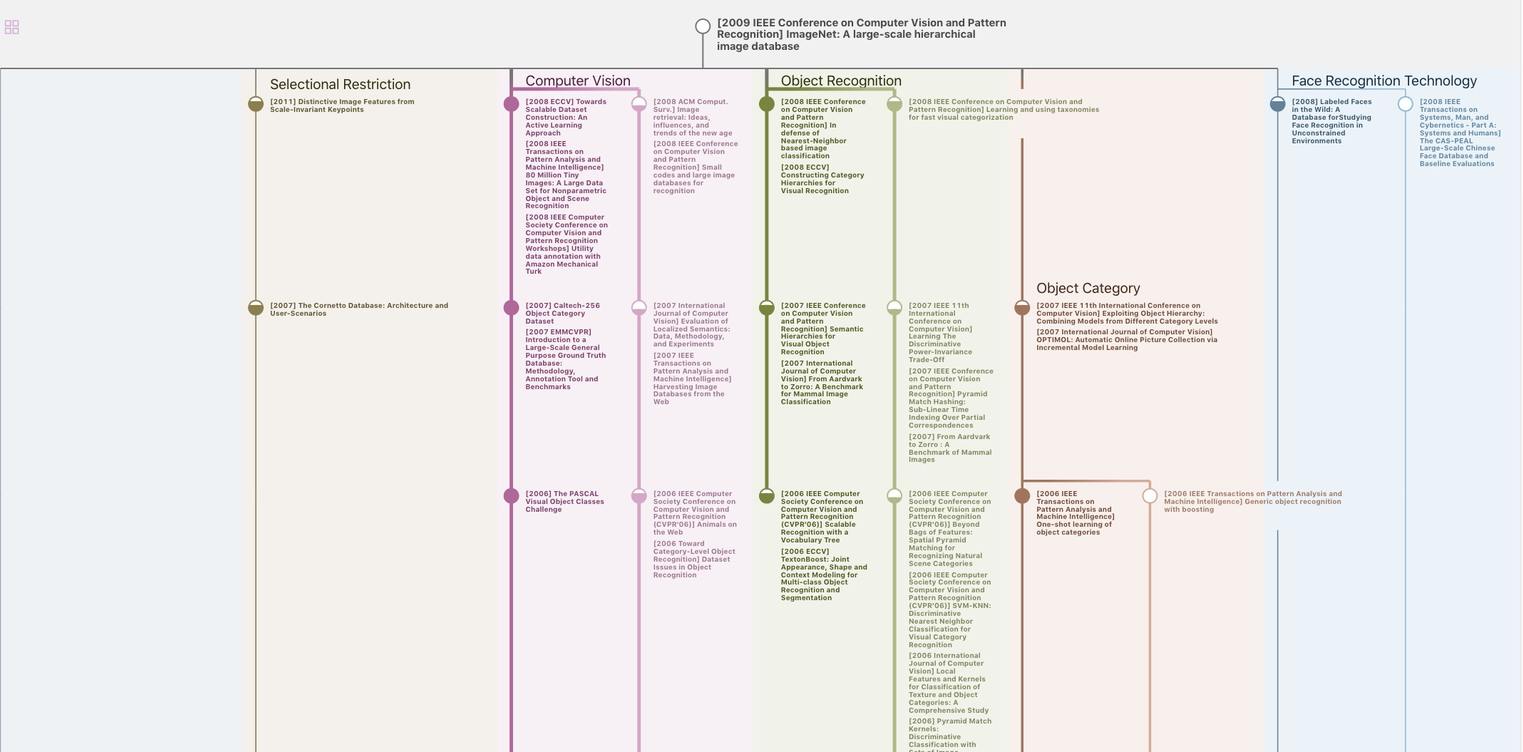
生成溯源树,研究论文发展脉络
Chat Paper
正在生成论文摘要