A Comparative Performance Analysis of Android Malware Classification Using Supervised and Semi-supervised Deep Learning.
International Conference on Security of Information and Networks(2023)
摘要
Mobile phones were originally designed for commu-nication, wherein they have evolved into multifunctional devices used for financial transactions, social media, and more, making them an integral mode of communication for the dweller's world. However, the mostly used OS in mobile devices, Android consist of various vulnerabilities and lack sufficient security measures, leaving them susceptible to malware injection. Moreover, the attackers develop sophisticated malware, which is challenging to detect by traditional detection approaches. Henceforth, an effective malware detection method is imperative to ensure the safety and security of Android systems. In this study, we address the dynamic analysis of Android malware using supervised and semi-supervised deep neural network techniques to tackle existing challenges. Our investigation is conducted on the CCCS-CIC-AndMal-2020 dataset and the results reveal that our proposed supervised models (1D CNN, MLP, RNN and LSTM) outperform state-of-the-art supervised models significantly with an accuracy of 99.76%. Additionally, we explore semi-supervised approach using limited label data, where our label spreading approach showcases a highly effective detection accuracy of 97.26%, approaching that of a fully supervised approach.
更多查看译文
AI 理解论文
溯源树
样例
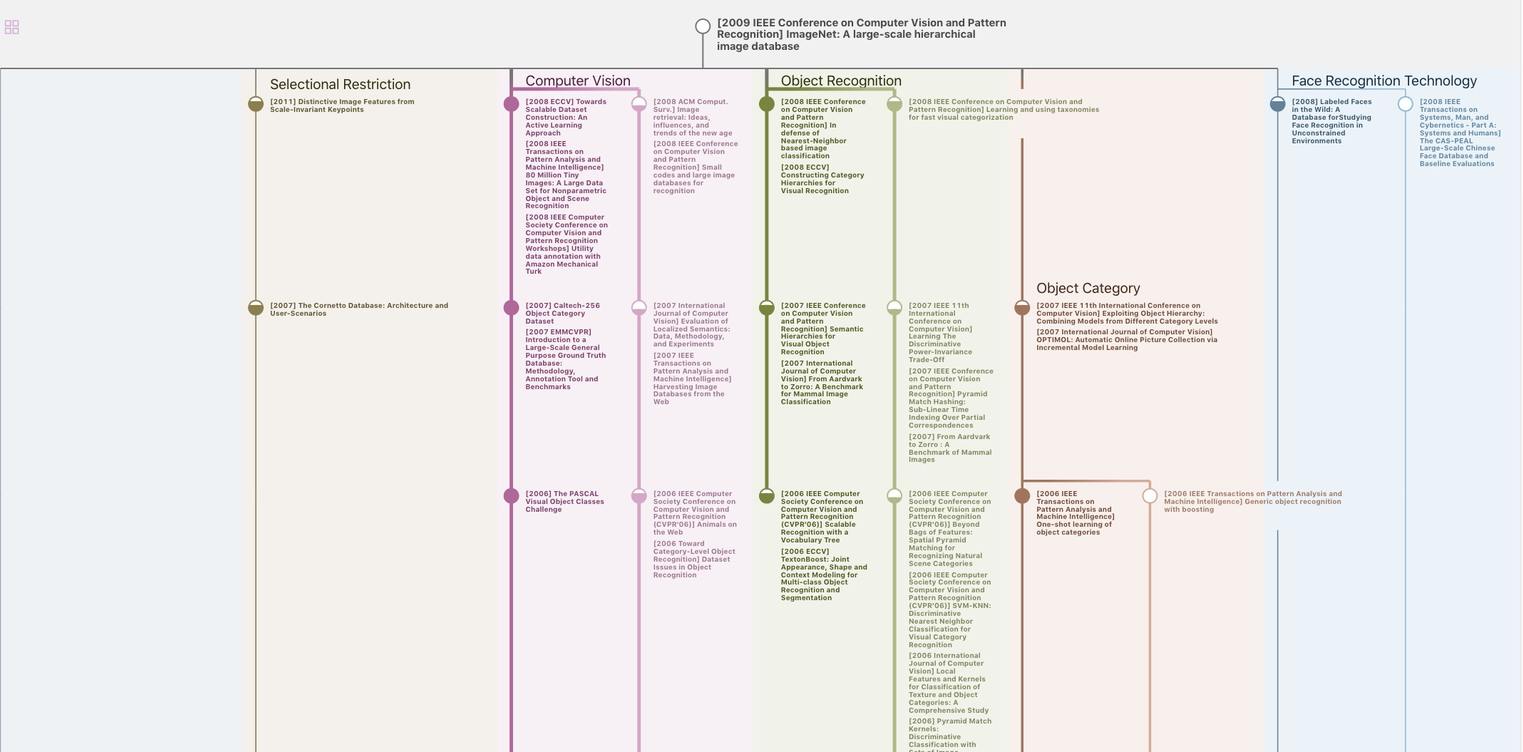
生成溯源树,研究论文发展脉络
Chat Paper
正在生成论文摘要