#122 : Extrapolating Endometriosis Diagnosis Using Imaging and Machine Learning: The Imagendo Project
Fertility & Reproduction(2023)
摘要
Background and Aims: Currently, it takes an average of 6.4 years to obtain a diagnosis for endometriosis. To address this delay, the IMAGENDO project combines ultrasounds (eTVUS) and Magnetic Resonance Images (eMRI) using Artificial Intelligence (AI). Our preliminary data has shown that a multimodal AI approach objectively assesses imaging data from eTVUS and eMRIs and improves diagnostic accuracy for endometriosis. Our first example shows how automated detection of Pouch of Douglas (POD) obliteration can be augmented by a novel multimodal approach. Aim: To improve eMRI detection of POD obliteration, by leveraging detection results from unpaired eTVUS data. Method: Retrospective specialist private and public imaging datasets of the female pelvis were obtained. After pre-training a machine learning model using 8,984 MRIs from a public dataset, we fine-tuned the algorithm using 88 private eMRIs, to detect POD obliteration. We then introduced another 749 unpaired eTVUSs to further improve our diagnostic model. Results: To resolve confounding problems with our eMRI datasets due to artefacts, mislabelling, and misreporting, we used model checking, student auditing and expert radiology review. Our results illustrated effective multimodal analysis methods which improved the POD obliteration detection accuracy from eMRI datasets. This model improved the Area Under the Curve (AUC) from 65.0% to 90.6%. Conclusion: We have been able to improve the accuracy of diagnosing endometriosis from eMRIs using a novel POD obliteration detection method. Our method extracts knowledge from unpaired eTVUSs and applies it to eMRI datasets. The detection of POD obliteration is automated from eMRI data. Combining images for corresponding endometriosis signs using algorithms, is the first step in improving the imaging diagnosis of endometriosis, when compared to surgery, and allows extrapolation of results when either imaging modality is missing. This will enable women to obtain a faster diagnosis, where adequate scanning is available, prior to undertaking surgery.
更多查看译文
AI 理解论文
溯源树
样例
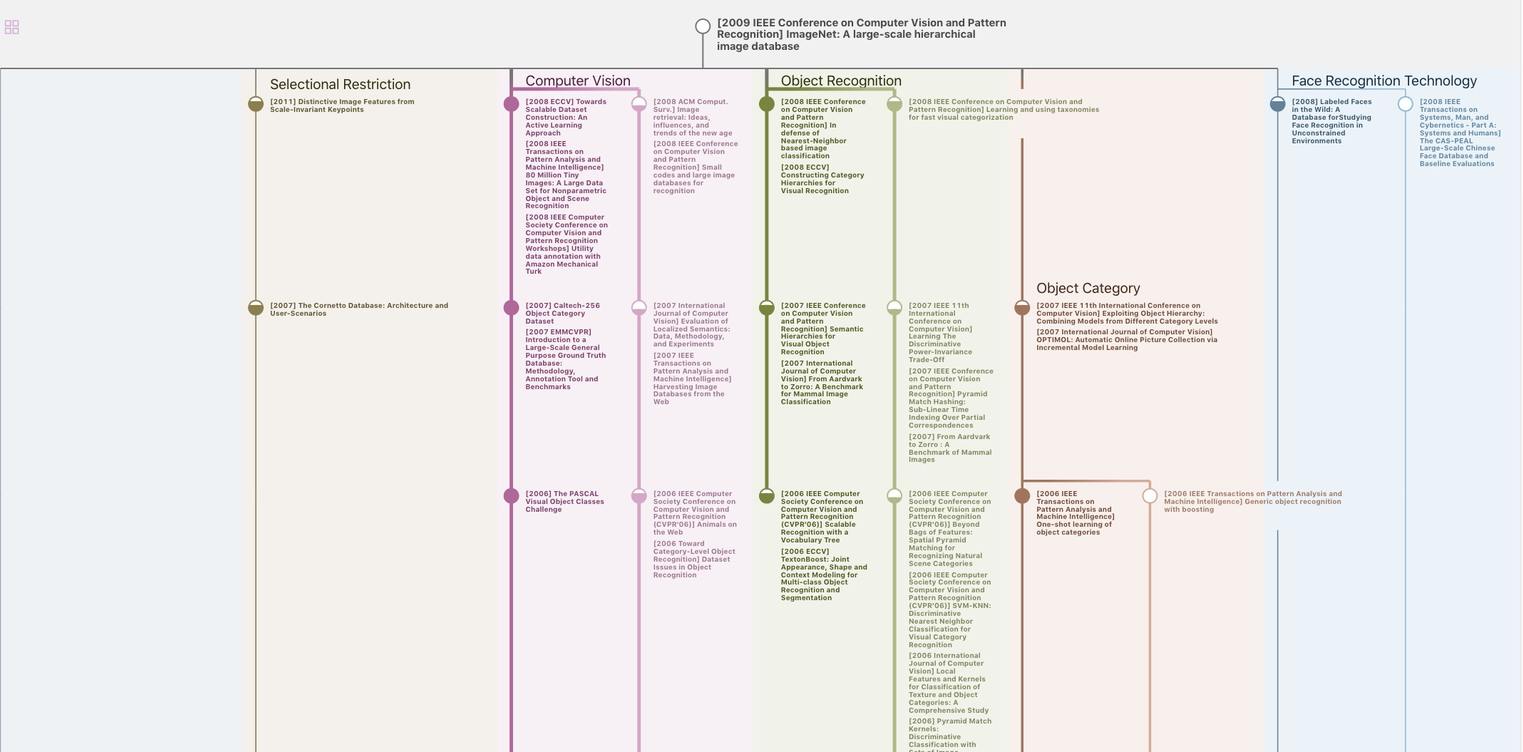
生成溯源树,研究论文发展脉络
Chat Paper
正在生成论文摘要