Fine-grained resource adjustment of edge server in cloud-edge collaborative environment
Cluster Computing(2024)
摘要
In the cloud-edge collaborative environment, the edge server manager will divide the physical resources based on virtualization technology, so as to deploy multiple applications on the same server. However, due to the imperfect virtualization technology and the complexity and dynamics of the applications deployed on virtual machines (VMs), it is difficult for cloud service providers to evaluate the performance of VMs and thus cannot implement dynamic resource management effectively. To address this problem, this paper proposes an adaptive resource allocation approach. Firstly, we use the profiling tools to collect hardware counters and corresponding performance that reflect the resource usage in real time. Then, we select the data instances that contribute more to the performance prediction based on Gradient-based One Side Sampling (GOSS) to build a VM performance prediction model. When the prediction results indicate the performance cannot meet users’ requirements, we further apply one of the reinforcement learning framework-Deep Deterministic Policy Gradient (DDPG) to optimize the allocation of fine-grained resources. Our proposed method enables adaptive allocation of fine-grained resources in cloud environment, and the extensive experiments demonstrate that the average accuracy of performance prediction by our proposed method surpasses 95
更多查看译文
关键词
Cloud-edge collaboration,Edge server,Fine-grained resource adjustment,Dynamical adjustment,Deep deterministic policy gradient
AI 理解论文
溯源树
样例
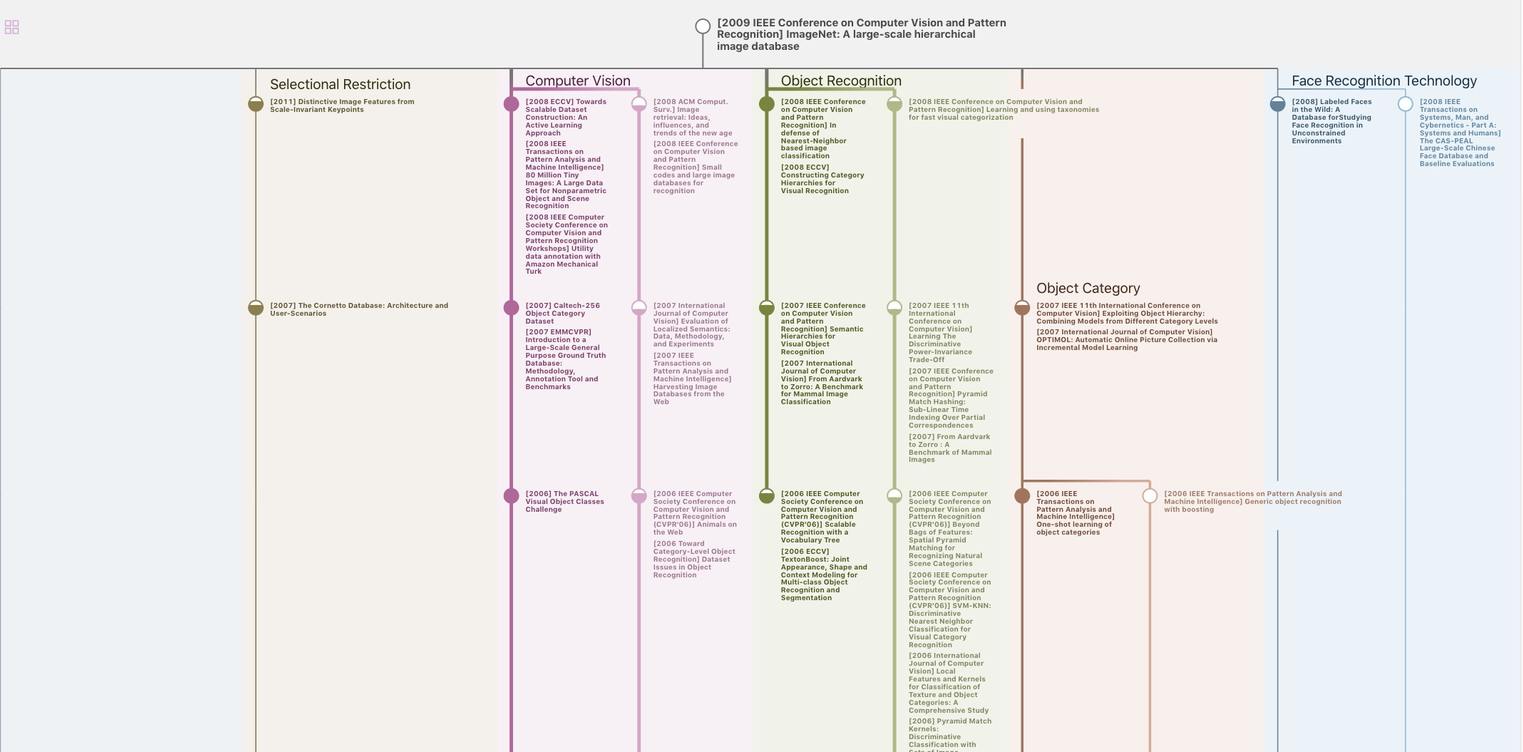
生成溯源树,研究论文发展脉络
Chat Paper
正在生成论文摘要