Enhancing the genomic prediction accuracy of swine agricultural economic traits using an expanded one-hot encoding in CNN models1
Journal of Integrative Agriculture(2024)
Abstract
Deep learning (DL) methods like Multilayer Perceptrons (MLPs) and Convolutional Neural Networks (CNNs) have been applied to predict the complex traits in animal and plant breeding. However, improving the genomic prediction accuracy still presents significant challenges. In this study, we applied CNNs to predict swine traits using previously published data. Specifically, we extensively evaluated the CNN model’s performance by employing various sets of Single Nucleotide Polymorphisms (SNPs) and concluded that the CNN model achieved optimal performance when utilizing SNP sets comprising 1,000 SNPs. Furthermore, we adopted a novel approach using the one-hot encoding method that transforms the 16 different genotypes into sets of eight binary variables. This innovative encoding method significantly enhanced the CNN’s prediction accuracy for swine traits, outperforming the traditional one-hot encoding techniques. Our findings suggest that the expanded one-hot encode method can improve the accuracy of DL methods in the genomic prediction of swine agricultural economic traits. This discovery has significant implications for swine breeding programs, where genomic prediction is pivotal in improving breeding strategies. Furthermore, future research endeavors can explore additional enhancements to DL methods by incorporating advanced data pre-processing techniques.
MoreTranslated text
Key words
Swine,Agricultural economic traits,Genomic prediction,Deep learning,One-hot encoding,Convolutional Neural Networks
AI Read Science
Must-Reading Tree
Example
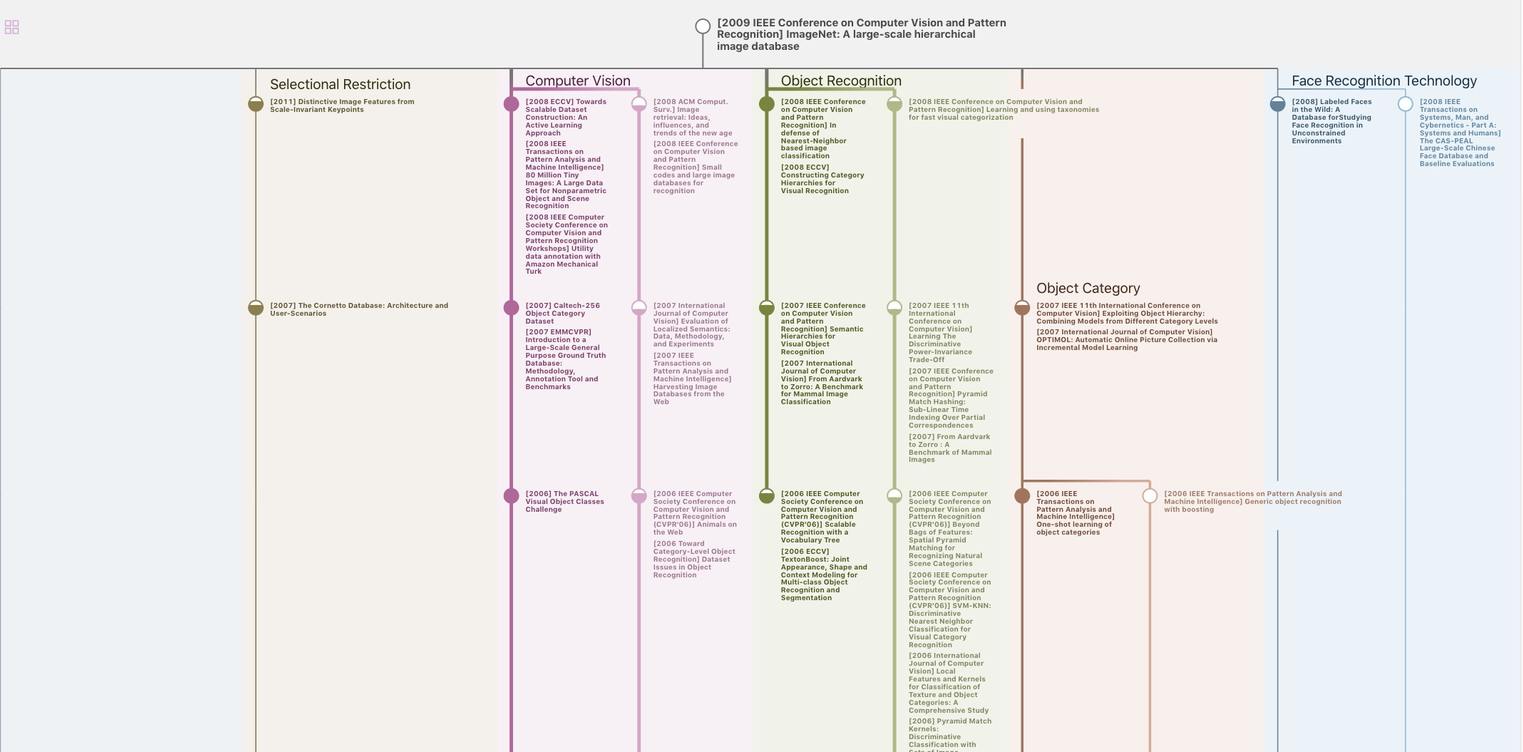
Generate MRT to find the research sequence of this paper
Chat Paper
Summary is being generated by the instructions you defined