VT-Grapher: Video Tube Graph Network with Self-Distillation for Human Action Recognition
IEEE Sensors Journal(2024)
摘要
The proliferation of videos captured by sensor-based cameras has driven the application of human action recognition (HAR) task. As the fundamental video application in human-computer interaction devices, HAR aims to identify human action in video clips, where lightweight networks are crucial. In this field, the convolutional neural networks and transformers have shown great potential for feature representation in Euclidean space, but ignoring more flexible non-Euclidean manifolds. To address this issue, we interpret a video as a set of graph nodes, and propose a Video Tube Graph network (VT-Grapher) for action recognition task. As the first lightweight graph neural network for RGB-based action recognition, our VT-Grapher contains three main components: 1) three spatial-temporal learning strategies for effectively mining the relationships between video visual features and semantics, where the Tube-in-Embedding Spatial-Temporal (TE-ST) strategy demonstrates the best balance between performance and computing; 2) the Video Tube Generation block with temporal center loss for generating the multiple granular video tubes based on temporal similarity and pushing away video tubes with low semantic similarity; 3) adversarial self-distillation method for enhancing the multi-granularity information aggregation capabilities of VT-Grapher. The proposed VT-Grapher network works in a plug-and-play way and can be integrated with vision graph neural networks, such as ViG and Mobile ViG. Extensive experiments are carried out on the Mini-Kinetics (Top-1 76.1%), Kinetics-400 (Top-1 73.7%), UCF101 (Acc 94.5%), and the multi-modal N-UCLA datasets (Top-1 99.7%), showing the effectiveness of VT-Grapher.
更多查看译文
关键词
Video Action Recognition,Vision Graph Neural Network,Temporal Cluster,Self-Distillation
AI 理解论文
溯源树
样例
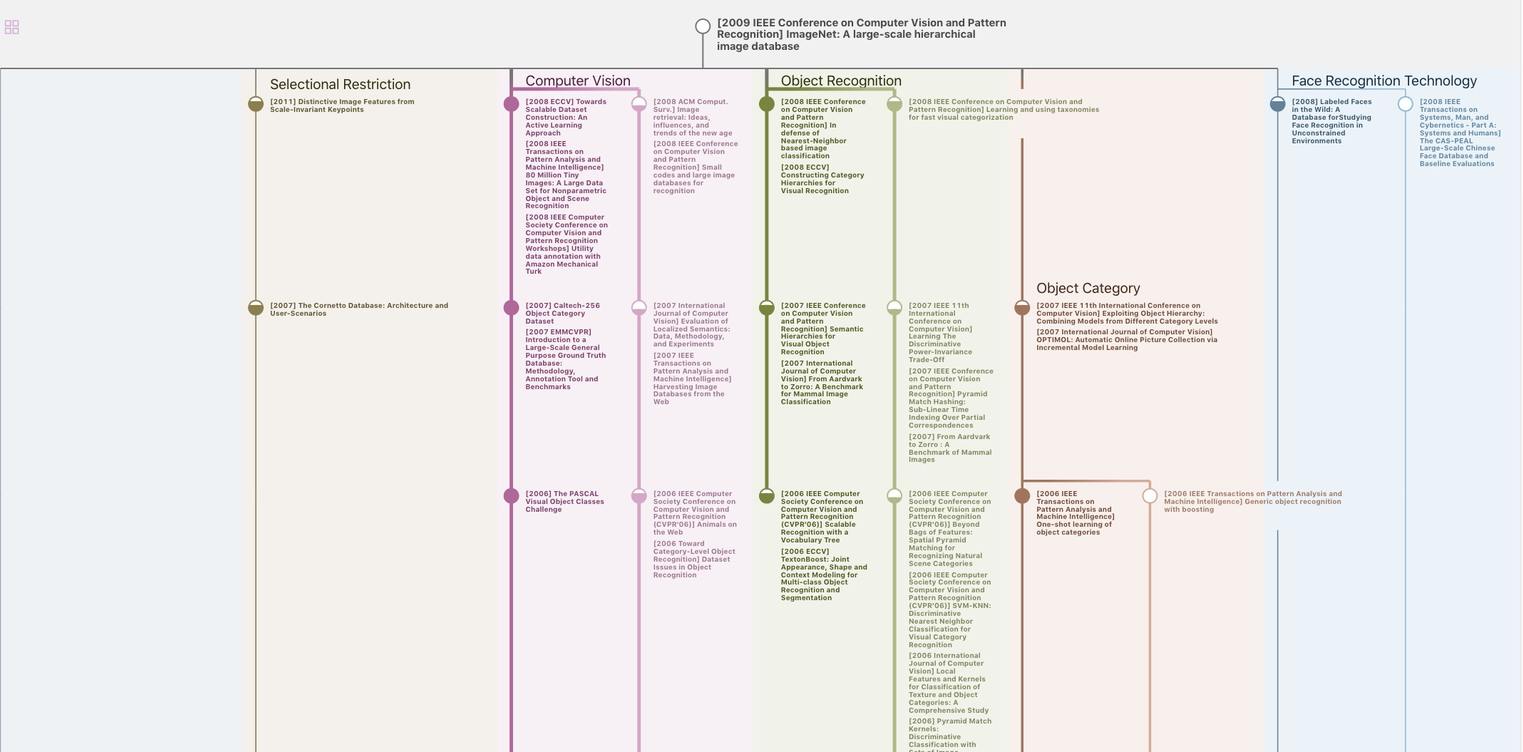
生成溯源树,研究论文发展脉络
Chat Paper
正在生成论文摘要