Generalizable and Discriminative Representations for Adversarially Robust Few-Shot Learning
IEEE TRANSACTIONS ON NEURAL NETWORKS AND LEARNING SYSTEMS(2024)
摘要
Few-shot image classification (FSIC) is beneficial for a variety of real-world scenarios, aiming to construct a recognition system with limited training data. In this article, we extend the original FSIC task by incorporating defense against malicious adversarial examples. This can be an arduous challenge because numerous deep learning-based approaches remain susceptible to adversarial examples, even when trained with ample amounts of data. Previous studies on this problem have predominantly concentrated on the meta-learning framework, which involves sampling numerous few-shot tasks during the training stage. In contrast, we propose a straightforward but effective baseline via learning robust and discriminative representations without tedious meta-task sampling, which can further be generalized to unforeseen adversarial FSIC tasks. Specifically, we introduce an adversarial-aware (AA) mechanism that exploits feature-level distinctions between the legitimate and the adversarial domains to provide supplementary supervision. Moreover, we design a novel adversarial reweighting training strategy to ameliorate the imbalance among adversarial examples. To further enhance the adversarial robustness without compromising discriminative features, we propose the cyclic feature purifier during the postprocessing projection, which can reduce the interference of unforeseen adversarial examples. Furthermore, our method can obtain robust feature embeddings that maintain superior transferability, even when facing cross-domain adversarial examples. Extensive experiments and systematic analyses demonstrate that our method achieves state-of-the-art robustness as well as natural performance among adversarially robust FSIC algorithms on three standard benchmarks by a substantial margin.
更多查看译文
关键词
Robustness,Training,Task analysis,Metalearning,Benchmark testing,Standards,Feature extraction,Adversarial example,few-shot image classification (FSIC),meta-learning,robust representation
AI 理解论文
溯源树
样例
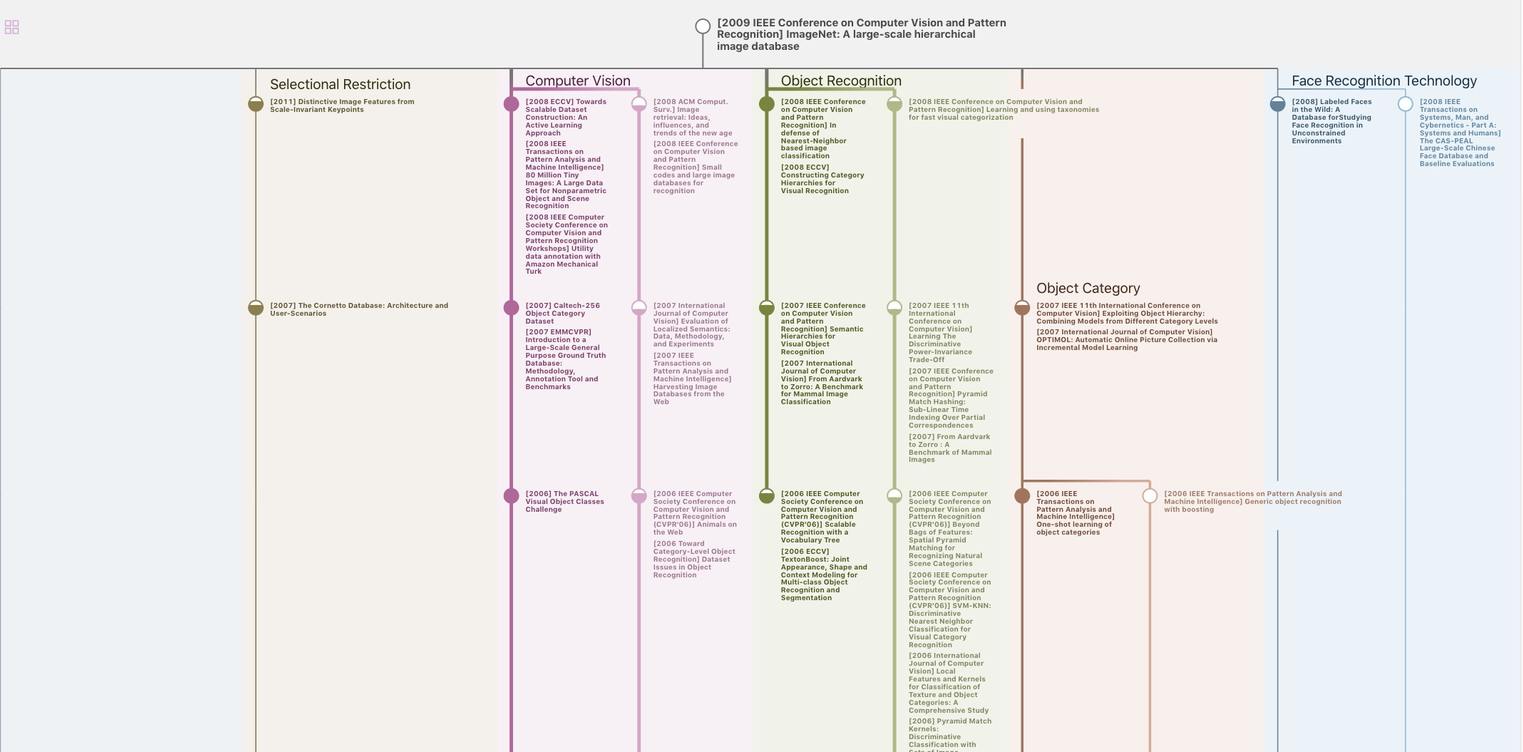
生成溯源树,研究论文发展脉络
Chat Paper
正在生成论文摘要