IMUNet: Efficient Regression Architecture for Inertial IMU Navigation and Positioning
IEEE Transactions on Instrumentation and Measurement(2024)
摘要
Data-driven method for inertial navigation and positioning has absorbed attention in recent years and it outperforms all its competitor methods in terms of accuracy and efficiency. This paper introduces a new neural architecture framework called IMUNet which is accurate and efficient for inertial position estimation on the edge device implementation receiving a sequence of raw Inertial Measurement Unit (IMU) measurements. The architecture has been compared with the one-dimension version of the state-of-the-art Convolutional Neural Network (CNN) networks that have been introduced recently for edge device implementation in terms of accuracy and efficiency. Moreover, a new method of collecting a dataset using IMU sensors on cell phones and Google ARCore Application Programming Interface (API) has been proposed and a publicly available dataset has been recorded. A comprehensive evaluation using four different datasets as well as the proposed dataset has been done to prove the performance of the proposed architecture. Our experiments show that the proposed framework outperforms state-of-the-art CNN networks in terms of efficiency on a variety of datasets while preserving the accuracy. All the code in both Pytorch and Tensorflow framework as well as the Android application code for data collection has been shared to improve further research.
更多查看译文
关键词
Deep Learning,IMU Measurement,Inertial Navigation,Sensor Fusion,Data-Driven Methods
AI 理解论文
溯源树
样例
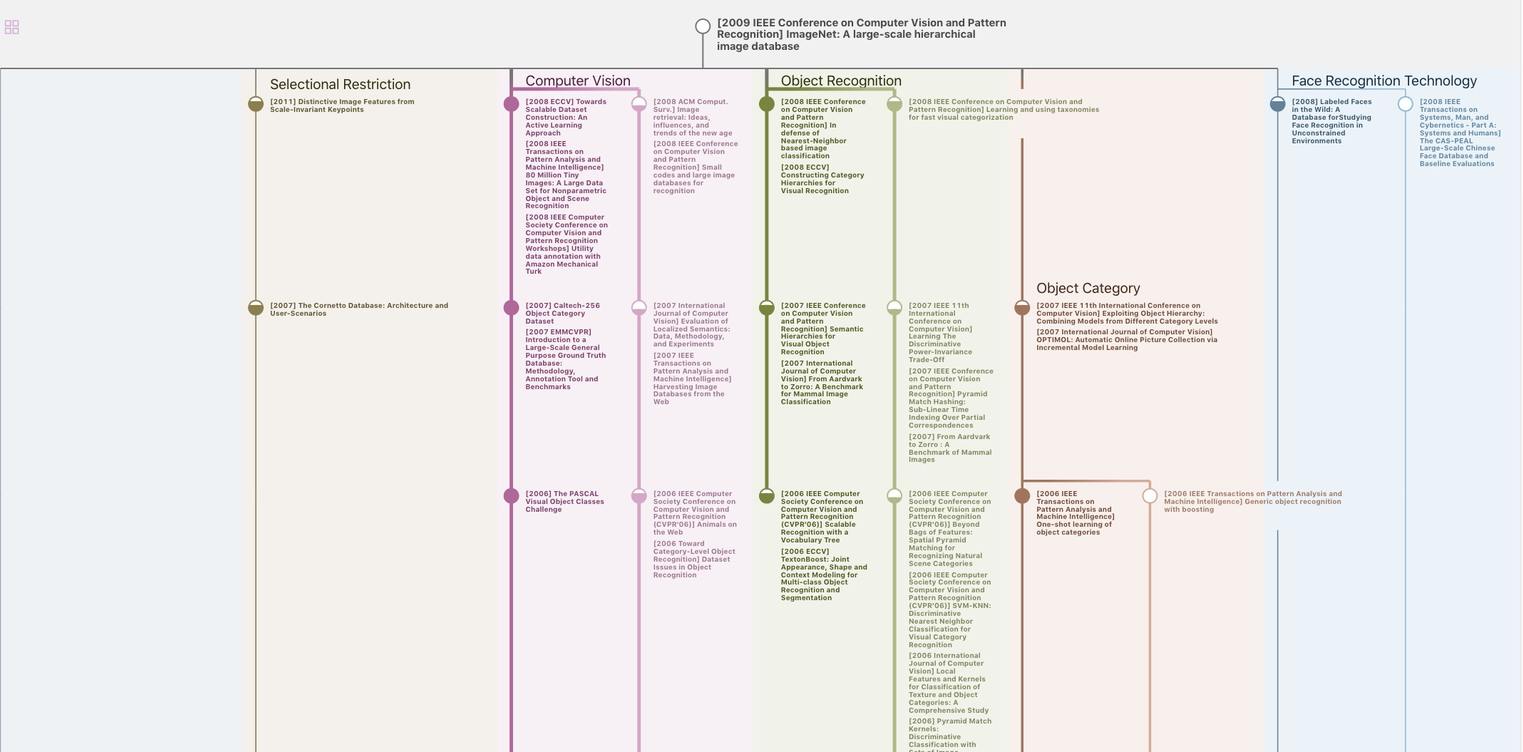
生成溯源树,研究论文发展脉络
Chat Paper
正在生成论文摘要