Structured-Anomaly Pursuit of Network Traffic via Corruption-Robust Low-Rank Tensor Decomposition.
IEEE Trans. Netw. Sci. Eng.(2024)
摘要
Accurately pursuing network traffic anomalies is crucial to network maintenance and management. However, existing methods generally focus on detecting uniformly distributed sparse noises and therefore fail to deal with non-uniform or sequential anomalies with effect. In this paper, a novel corruption-robust low-rank tensor decomposition (Cr-LTD) method is proposed for accurate and efficient structured-anomaly pursuit even in presence of sparse corruptions. For the intrinsically low-rank network traffic observation, Cr-LTD models it as a three-way tensor and formulates the traffic anomaly pursuit as a low-rank tensor decomposition problem. The intrinsically low-rank structure of network traffic tensor is depicted via a novel tensor nuclear norm which is a tight convex surrogate of tensor tubal rank.
${l}_{2,1}$
-norm and
${l}_1$
-norm are also introduced in Cr-LTD respectively for effective characterization on structured-anomaly and strong robustness to sparse corruption. Equipped with tensor nuclear norm and two regularizations, Cr-LTD achieves the low-rank tensor decomposition via solving and accelerating a convex program, thereby pursuing structured-anomaly robustly. Extensive experiments are conducted using a set of synthetic data and real-world network traffic datasets. Experiment results verify the superior performance of Cr-LTD over the state-of-the-art methods in terms of pursuit accuracy and corruption robustness.
更多查看译文
关键词
Network traffic,Anomaly pursuit,Low-rank decomposition,Tensor theories and methods
AI 理解论文
溯源树
样例
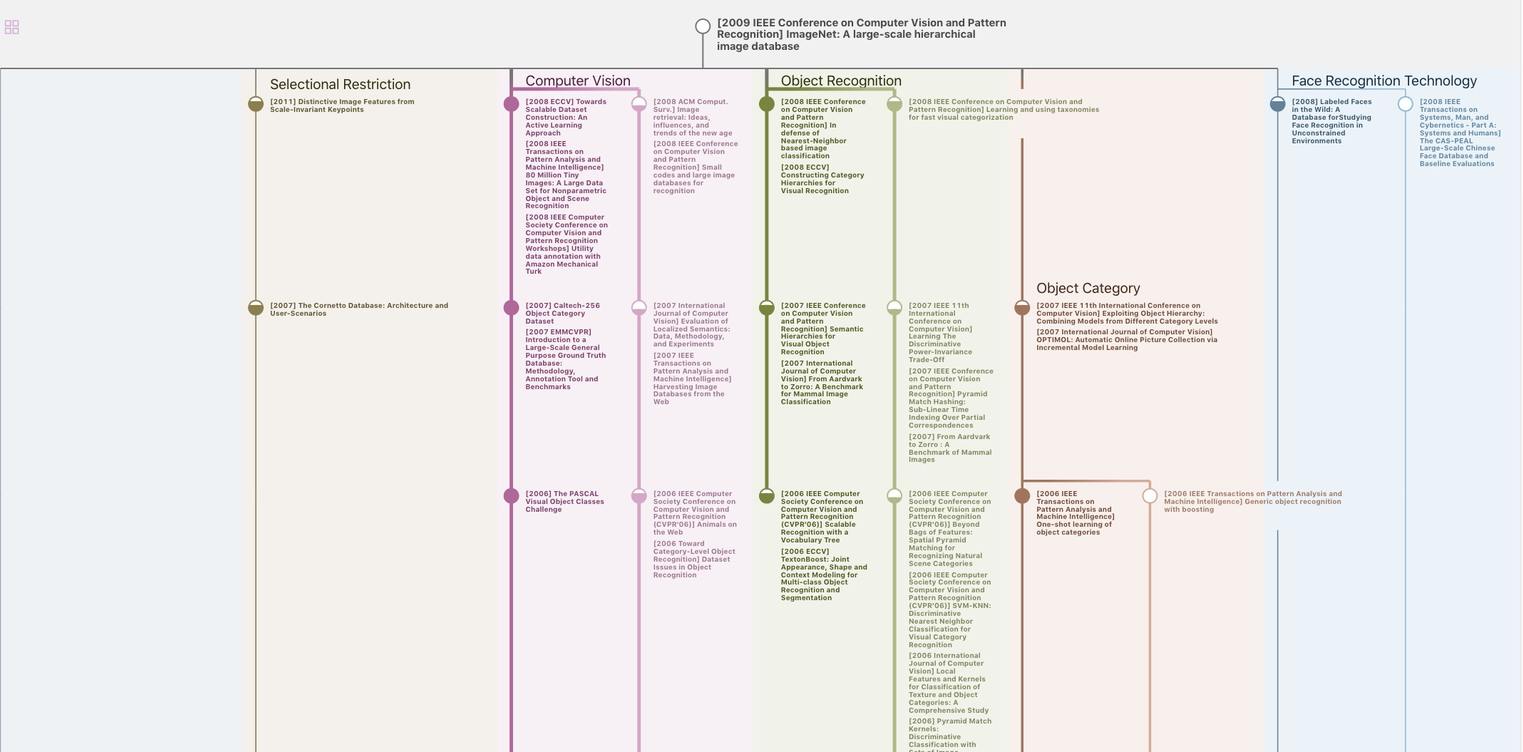
生成溯源树,研究论文发展脉络
Chat Paper
正在生成论文摘要