Collaborative Parking Vacancy Prediction for Cities With Partial Sensors Missing
IEEE TRANSACTIONS ON INTELLIGENT TRANSPORTATION SYSTEMS(2024)
摘要
City-wide short-term parking vacancy (PV) prediction is essential to transportation management. In many cities, only partial parking lots are equipped with sensors, causing severe data missing issues. Predicting PVs for parking lots without sensors is attractive but challenging. First, PV prediction itself is a nontrivial task since spatial dependencies among different regions in a city are complex and dynamic. By connecting parking lots based on geographical closeness using pre-designed rules, state-of-the-art PV prediction models achieve reasonable performance. However, those connectivities are not able to adapt to capture the changing dependencies in the graph. Second, node-to-node-based spatial and temporal dependencies will no longer be reliable since neighboring parking lot sensors may be intensely missing, which deteriorates the ability of the model to accurately impute PVs, leveraging the complex dependencies. To this end, we propose a novel framework named Collaborative graph Learning for pArking vacancy Prediction (CLaP). Specifically, to overcome the limitation of traditional pre-designed node connections, we propose a collaborative training method incorporating node attributes into graph augmentation, thus enhancing the ability to capture dynamic spatial dependencies through message aggregation. Besides, a recurrent PV recovering module is developed to impute missing embeddings by deeply coupling spatial-temporal dependencies. Experimental results on two real-world datasets demonstrate that CLaP outperforms state-of-the-art PV prediction models on city-wide PV prediction and imputation precision for parking lots without sensors.
更多查看译文
关键词
Sensors,Feature extraction,Collaboration,Urban areas,Intelligent sensors,Real-time systems,Predictive models,Parking vacancy prediction,graph neural network,traffic prediction with data missing
AI 理解论文
溯源树
样例
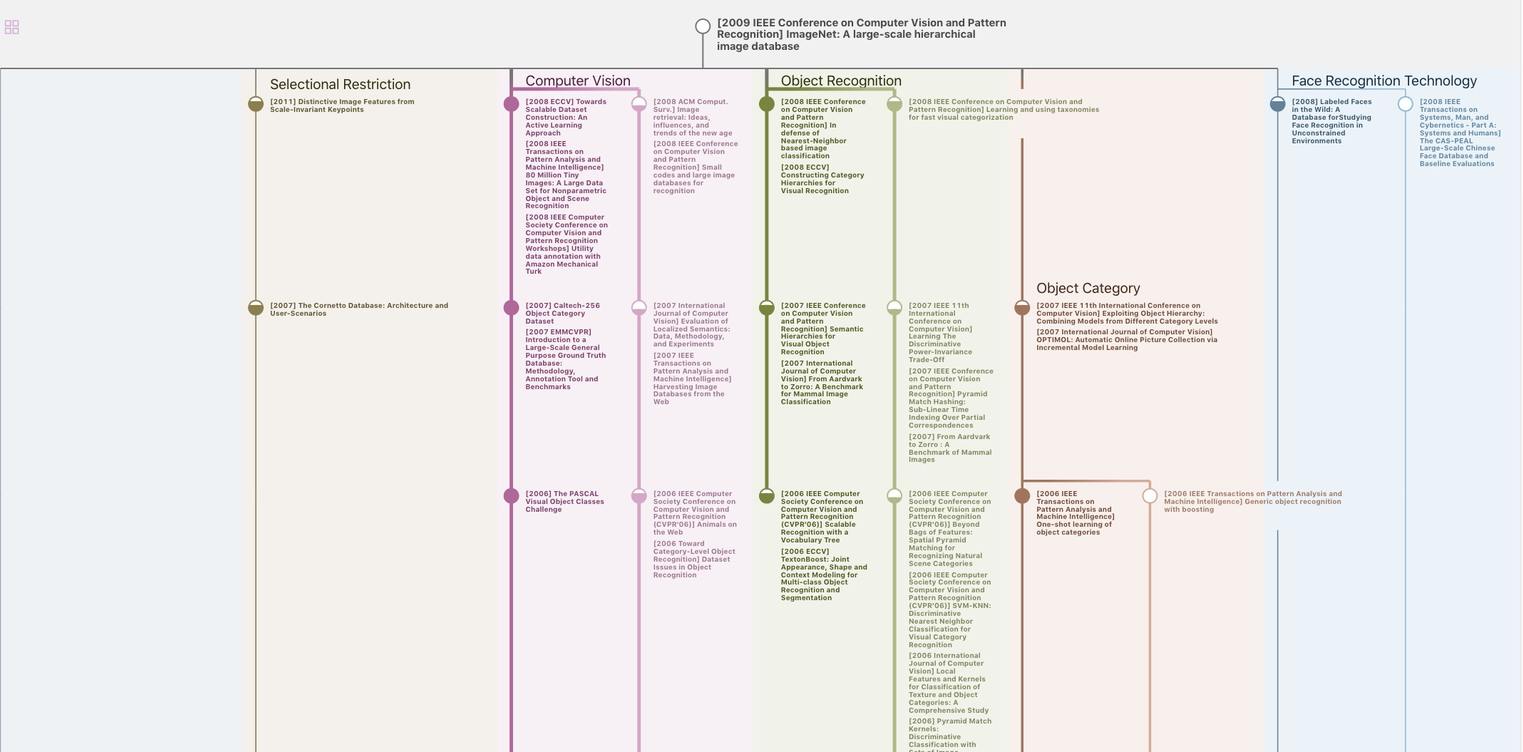
生成溯源树,研究论文发展脉络
Chat Paper
正在生成论文摘要