Study on identification method of driver fatigue considering individual ECG differences
Cognition, Technology and Work(2024)
Abstract
Driver fatigue is one of the main causes of road traffic accidents. Because of the individual differences between drivers, the common characteristics of driver fatigue are not clear, and existing fatigue identification algorithms cannot meet the prospective requirements of fatigue identification. In this study, based on a driver's electrocardiogram signal, 17 feature indices were extracted as candidate features for driver fatigue classification from three aspects: frequency domain features, time domain features and non-linear features. In order to improve the generalization ability of the driver fatigue classification and recognition model and to reduce the negative impact of individual variability on the generalized model, the support vector machine (SVM) discriminant function information was introduced as the ranking evaluation criterion, the recursive feature elimination (SVM-RFE) algorithm was used as the search strategy, and the correlation bias reduction algorithm was introduced to avoid the evaluation bias, and the importance ranking of the fatigue feature indicators was realized. A ranked list of the importance of the candidate features in the fatigue sample of each test driver was generated. Based on the ranked list of features for each test driver, an evaluation matrix K of driver fatigue feature importance was proposed, and the feature importance index V a was defined. To quantify the importance of candidate features for overall fatigue identification, eight key features that significantly characterize driver fatigue were selected as the optimal feature subset. Finally, a two- class identification model of the driver fatigue state based on the SVM algorithm was designed to verify the effectiveness of the identification model considering individual differences. The results show that the average classification accuracy of the proposed identification model was 87.04%, which was 9.20% higher than that of a model that did not consider individual driver differences. The performance of the model was significantly improved by selecting features that were less affected by individual differences in the driver fatigue identification model.
MoreTranslated text
Key words
Traffic safety,Driver fatigue,ECG signals,Individual differences,SVM
AI Read Science
Must-Reading Tree
Example
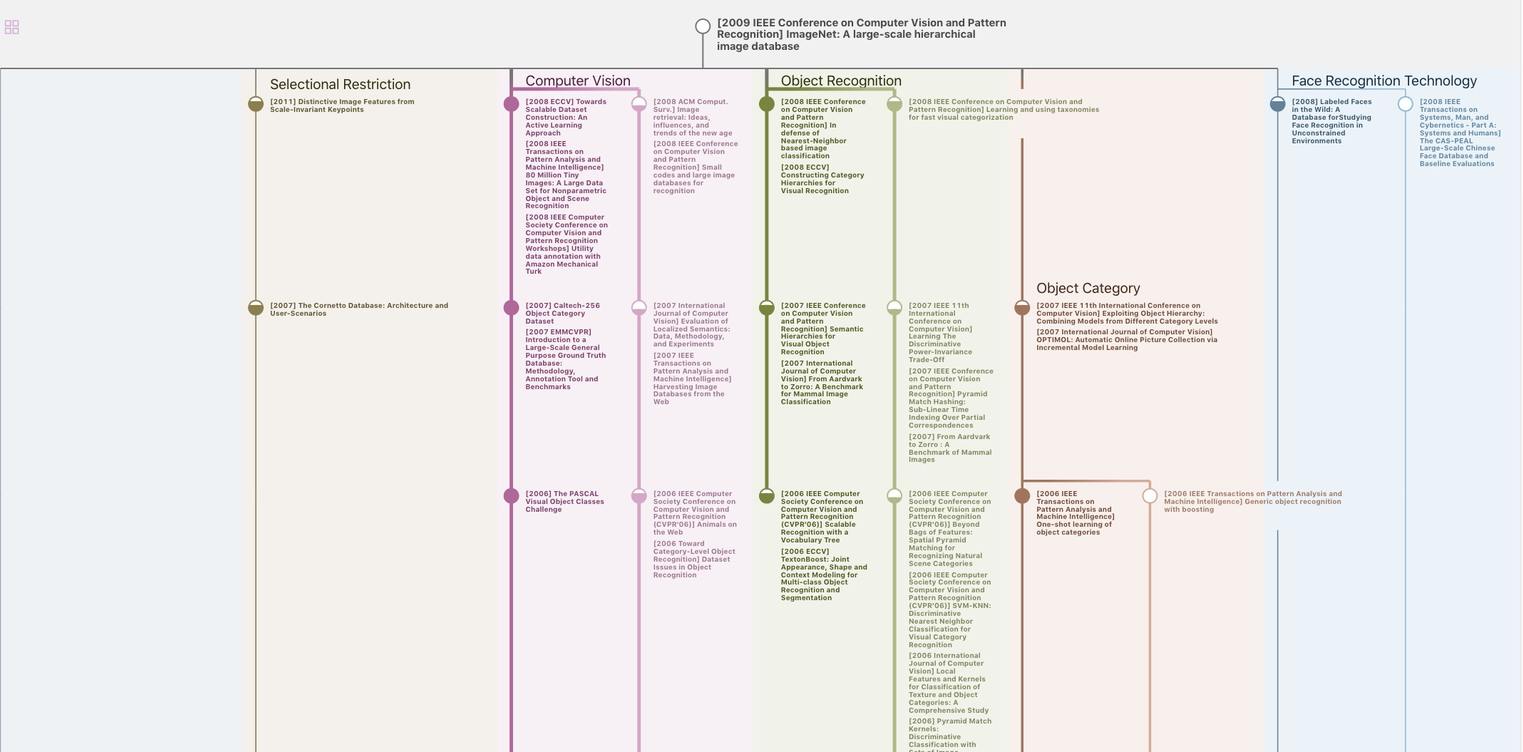
Generate MRT to find the research sequence of this paper
Chat Paper
Summary is being generated by the instructions you defined