Differentially Private Distributed Nonconvex Stochastic Optimization with Quantized Communications
arxiv(2024)
摘要
This paper proposes a new distributed nonconvex stochastic optimization
algorithm that can achieve privacy protection, communication efficiency and
convergence simultaneously. Specifically, each node adds time-varying privacy
noises to its local state to avoid information leakage, and then quantizes its
noise-perturbed state before transmitting to improve communication efficiency.
By employing the subsampling method controlled through the sample-size
parameter, the proposed algorithm reduces the impact of privacy noises, and
enhances the differential privacy level. When the global cost function
satisfies the Polyak-Lojasiewicz condition, the mean and high-probability
convergence rate and the oracle complexity of the proposed algorithm are given.
Importantly, the proposed algorithm achieves both the mean convergence and a
finite cumulative differential privacy budget over infinite iterations as the
sample-size goes to infinity. A numerical example of the distributed training
on the "MNIST" dataset is given to show the effectiveness of the algorithm.
更多查看译文
AI 理解论文
溯源树
样例
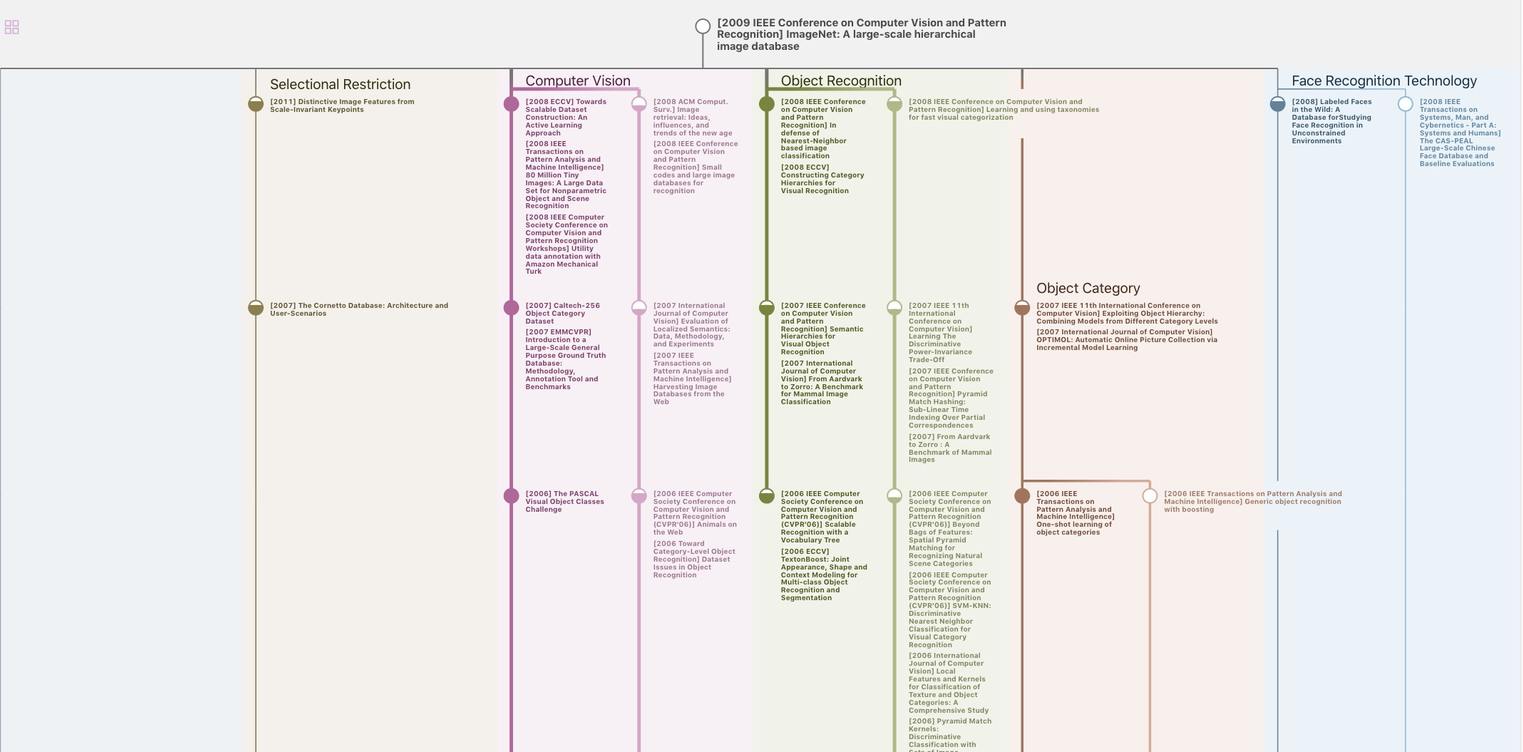
生成溯源树,研究论文发展脉络
Chat Paper
正在生成论文摘要