Noninvasive Assessment of hiPSC Differentiation toward Cardiomyocytes Using Pretrained Convolutional Neural Networks and the Channel Pruning Algorithm
ACS BIOMATERIALS SCIENCE & ENGINEERING(2024)
摘要
Human induced pluripotent stem cell (hiPSC)-derived cardiomyocytes (hiPSC-CMs) offer versatile applications in tissue engineering and drug screening. To facilitate the monitoring of hiPSC cardiac differentiation, a noninvasive approach using convolutional neural networks (CNNs) was explored. HiPSCs were differentiated into cardiomyocytes and analyzed using the quantitative real-time polymerase chain reaction (qRT-PCR). The bright-field images of the cells at different time points were captured to create the dataset. Six pretrained models (AlexNet, GoogleNet, ResNet 18, ResNet 50, DenseNet 121, VGG 19-BN) were employed to identify different stages in differentiation. VGG 19-BN outperformed the other five CNNs and exhibited remarkable performance with 99.2% accuracy, recall, precision, and F1 score and 99.8% specificity. The pruning process was then applied to the optimal model, resulting in a significant reduction of model parameters while maintaining high accuracy. Finally, an automation application using the pruned VGG 19-BN model was developed, facilitating users in assessing the cell status during the myocardial differentiation of hiPSCs.
更多查看译文
关键词
hiPSC-derived cardiomyocytes,differentiation,convolutional neural networks,model pruning,hyperparameters
AI 理解论文
溯源树
样例
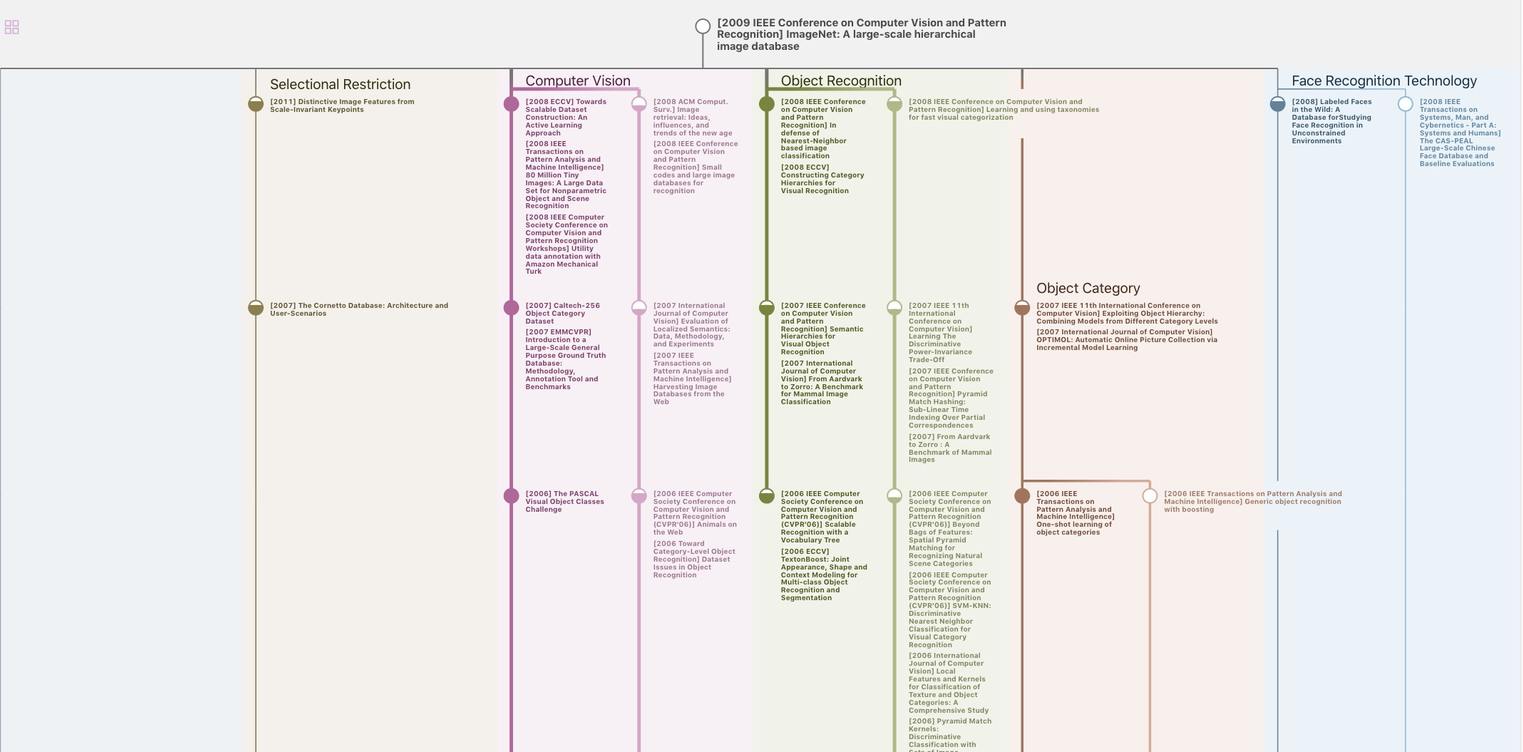
生成溯源树,研究论文发展脉络
Chat Paper
正在生成论文摘要