Analyzing Biomedical Datasets with Symbolic Tree Adaptive Resonance Theory
INFORMATION(2024)
摘要
Biomedical datasets distill many mechanisms of human diseases, linking diseases to genes and phenotypes (signs and symptoms of disease), genetic mutations to altered protein structures, and altered proteins to changes in molecular functions and biological processes. It is desirable to gain new insights from these data, especially with regard to the uncovering of hierarchical structures relating disease variants. However, analysis to this end has proven difficult due to the complexity of the connections between multi-categorical symbolic data. This article proposes symbolic tree adaptive resonance theory (START), with additional supervised, dual-vigilance (DV-START), and distributed dual-vigilance (DDV-START) formulations, for the clustering of multi-categorical symbolic data from biomedical datasets by demonstrating its utility in clustering variants of Charcot-Marie-Tooth disease using genomic, phenotypic, and proteomic data.
更多查看译文
关键词
adaptive resonance theory,biomedical data,categorical data,ontologies,knowledge graphs
AI 理解论文
溯源树
样例
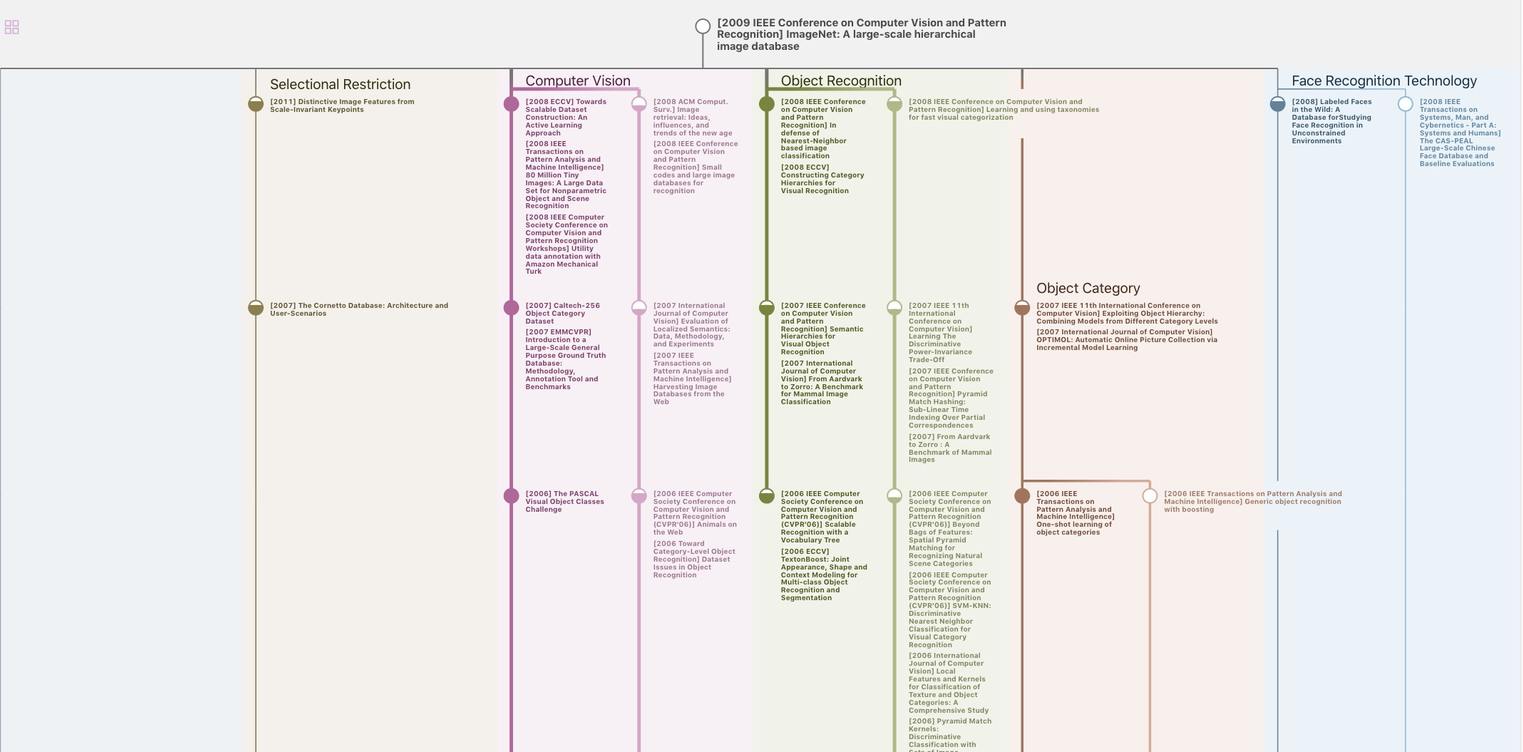
生成溯源树,研究论文发展脉络
Chat Paper
正在生成论文摘要