Frequency-Aware Degradation Modeling for Real-World Thermal Image Super-Resolution
ENTROPY(2024)
摘要
The supervised super-resolution (SR) methods based on simple degradation assumptions (e.g., bicubic downsampling) have unsatisfactory generalization ability on real-world thermal images. To enhance the SR effect of real-world sceneries, we introduce an unsupervised SR framework for thermal images, incorporating degradation modeling and corresponding SR. Inspired by the physical prior that high frequency affects details and low frequency affects thermal contrast, we propose a frequency-aware degradation model, named TFADGAN. The model achieves image quality migration between thermal detectors of different resolutions by degrading different frequency components of the image from high-resolution (HR) to low-resolution (LR). Specifically, by adversarial learning with unpaired LR thermal images, the complex degradation processes of HR thermal images at low and high frequencies are modeled separately. Benefiting from the thermal characteristics mined from real-world images, the degraded images generated by TFADGAN are similar to LR thermal ones in terms of detail and contrast. Then, the SR model is trained based on the pseudo-paired data consisting of degraded images and HR images. Extensive experimental results demonstrate that the degraded images generated by TFADGAN provide reliable alternatives to real-world LR thermal images. In real-world thermal image experiments, the proposed SR framework can improve the peak signal-to-noise ratio (PSNR) and structural similarity degree (SSIM) by 1.28 dB and 0.02, respectively.
更多查看译文
关键词
real-world thermal image,super-resolution,degradation modeling,frequency-aware
AI 理解论文
溯源树
样例
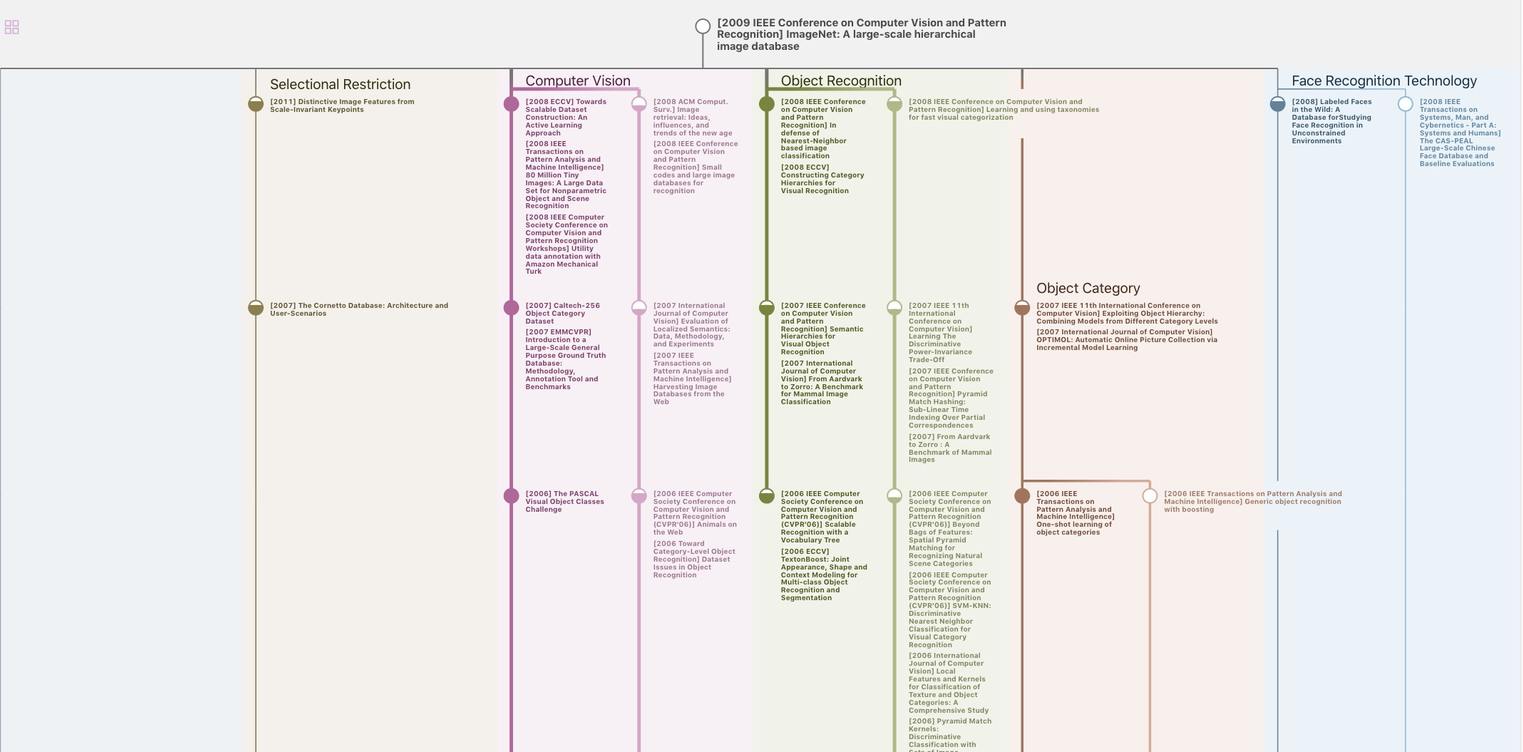
生成溯源树,研究论文发展脉络
Chat Paper
正在生成论文摘要