Incentive Mechanism Against Bounded Rationality for Federated Learning-Enabled Internet of UAVs: A Prospect Theory-Based Approach
IEEE Internet of Things Journal(2024)
摘要
Unmanned aerial vehicles (UAVs) equipped with high definition (HD) cameras, intelligent sensors, computing, and communication modules can be deployed to execute crowdsensing tasks by leveraging federated learning (FL), e.g., air quality perception and ground target detection. FL can reduce transmission stress and protect data privacy when training models, which is suitable for resource constrained Internet of UAVs. Nevertheless, the incentive issues about information asymmetry and bounded rationality impede the applications of FL-enabled Internet of UAVs. The existing FL incentive approaches focus on the risk-free condition, where task publishers are capable of making decisions with complete rationality by utilizing expected utility theory. In fact, task publishers under risk conditions are often bounded rational, whose risk-awareness makes the utility models more sophisticated. To overcome the above problems, we present a prospect theory based incentive mechanism for FL-enabled Internet of UAVs. We first leverage prospect theory to model the task publishers risk-awareness behavior and construct the subjective utility model. Thereafter, we utilize the framing effect of prospect theory to design the optimal contract to maximize the subjective utility. Simulation results demonstrate that, compared with the baseline method, the proposed incentive mechanism has better performance.
更多查看译文
AI 理解论文
溯源树
样例
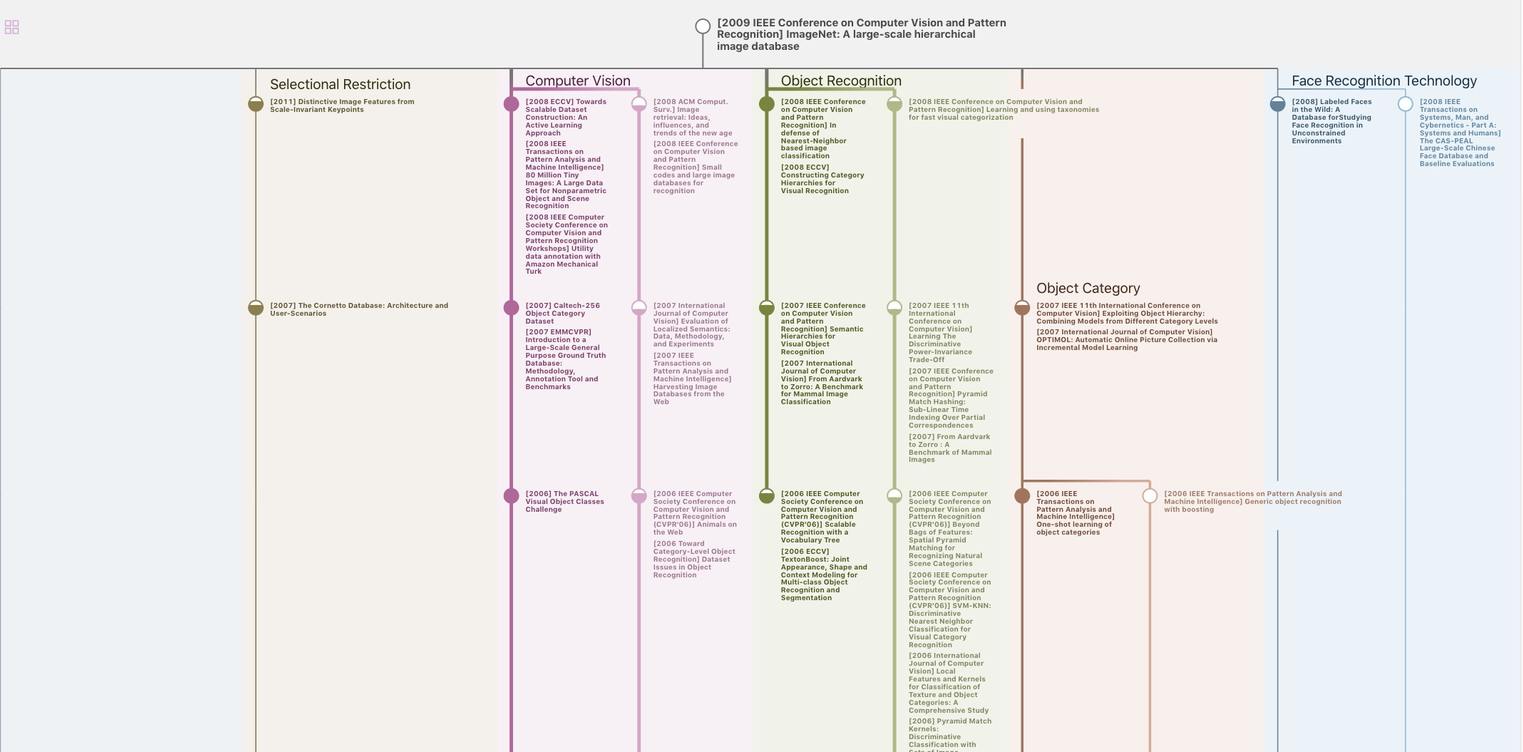
生成溯源树,研究论文发展脉络
Chat Paper
正在生成论文摘要