Resilience of Federated Learning Against False Data Injection Attacks in Energy Forecasting
2024 International Conference on Green Energy, Computing and Sustainable Technology (GECOST)(2024)
摘要
Federated learning (FL) has established itself as a communication-efficient, privacy-aware, and cost-effective technique for training machine learning models in energy forecasting. This approach enables simultaneous model training across multiple smart grids while keeping data decentralized at edge nodes. However, FL is not immune to backdoor adversarial attacks, such as data and model poisoning. In this paper, we scrutinize the impact of two data poisoning techniques: scaling and random noise effects. The attack was initiated on one client among ten. As the attack percentage increases, the Mean Absolute Percentage Error (MAPE) of the local model also rises. Our simulation results reveal that the scaling effect elevated MAPE from 0.193% to 32.72%, while random noise increased MAPE from 0.183% to 129.75% as the attacked percentage rose from 10% to 100%. It is concluded that data poisoning solely affects the local model and does not significantly impact the global model; hence, it can provide more resilience than centralized machine learning models.
更多查看译文
AI 理解论文
溯源树
样例
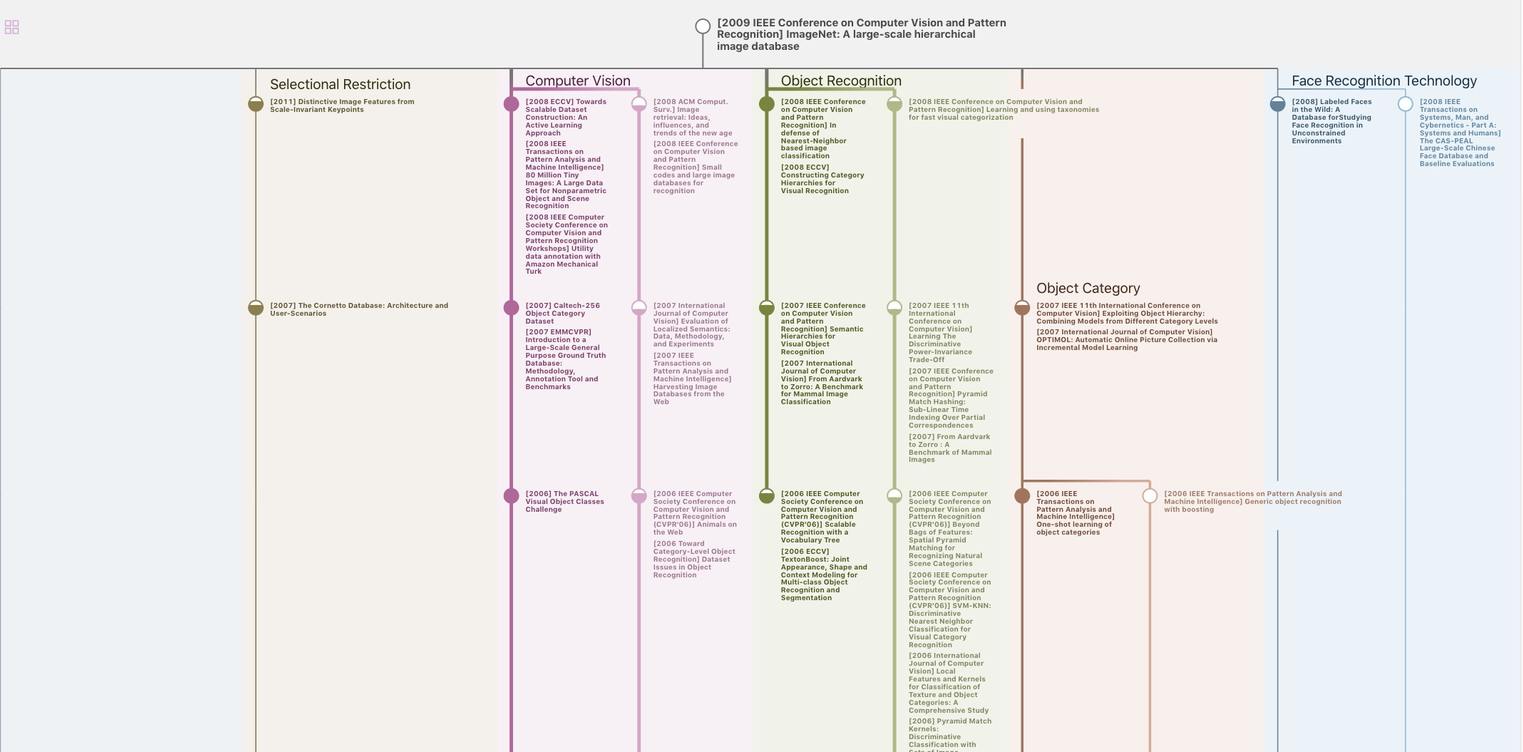
生成溯源树,研究论文发展脉络
Chat Paper
正在生成论文摘要