Five percent weight loss is a significant 1-year predictor and an optimal 5-year cut-off for reducing the number of obesity-related cardiovascular disease risk components: the Japan Obesity and Metabolic Syndrome Study
FRONTIERS IN ENDOCRINOLOGY(2024)
摘要
Objective: This study aimed to identify the amount of weight loss needed in patients with obesity to improve metabolic syndrome (MetS), a risk factor for cardiovascular disease (CVD), over a long period of time. Methods: A total of 576 patients with obesity were enrolled in this study. Effects of continuous physician-supervised weight loss on the cumulative MetS components excluding abdominal circumference (defined as obesity-related CVD risk score) were investigated during a 5-year follow-up period. The extent of weight loss required to reduce the obesity-related CVD risk components was assessed using receiver operating characteristic (ROC) curve analyses. Results: Of the 576 participants, 266 completed 5-year follow-up, with 39.1% and 24.1% of them achieving >= 5.0% and >= 7.5% weight loss at the 5-year follow-up, respectively. The area under the ROC curve for reducing the obesity-related CVD risk components was 0.719 [0.662-0.777] at 1 year and 0.694 [0.613-0.775] at 5 years. The optimal cut-off value for weight loss was 5.0% (0.66 sensitivity and 0.69 specificity) and the value with 0.80 specificity was 7.5% (0.45 sensitivity) at 5 years. Greater reductions in weight were associated with greater improvements in the obesity-related CVD risk score at all follow-up periods (P-trend <0.001). Obesity-related CVD risk score was significantly improved by 5.0-7.5% and >= 7.5% weight loss at 1 year (P = 0.029 and P < 0.001, respectively) and >= 7.5% weight loss at 5 years (P = 0.034). Conclusions: A weight loss of >= 5.0% at 1 year and >= 7.5% at 5 years could reduce the number of obesity-related CVD risk components in patients with obesity.
更多查看译文
关键词
obesity,weight loss,metabolic syndrome,cohort study,5-year follow-up
AI 理解论文
溯源树
样例
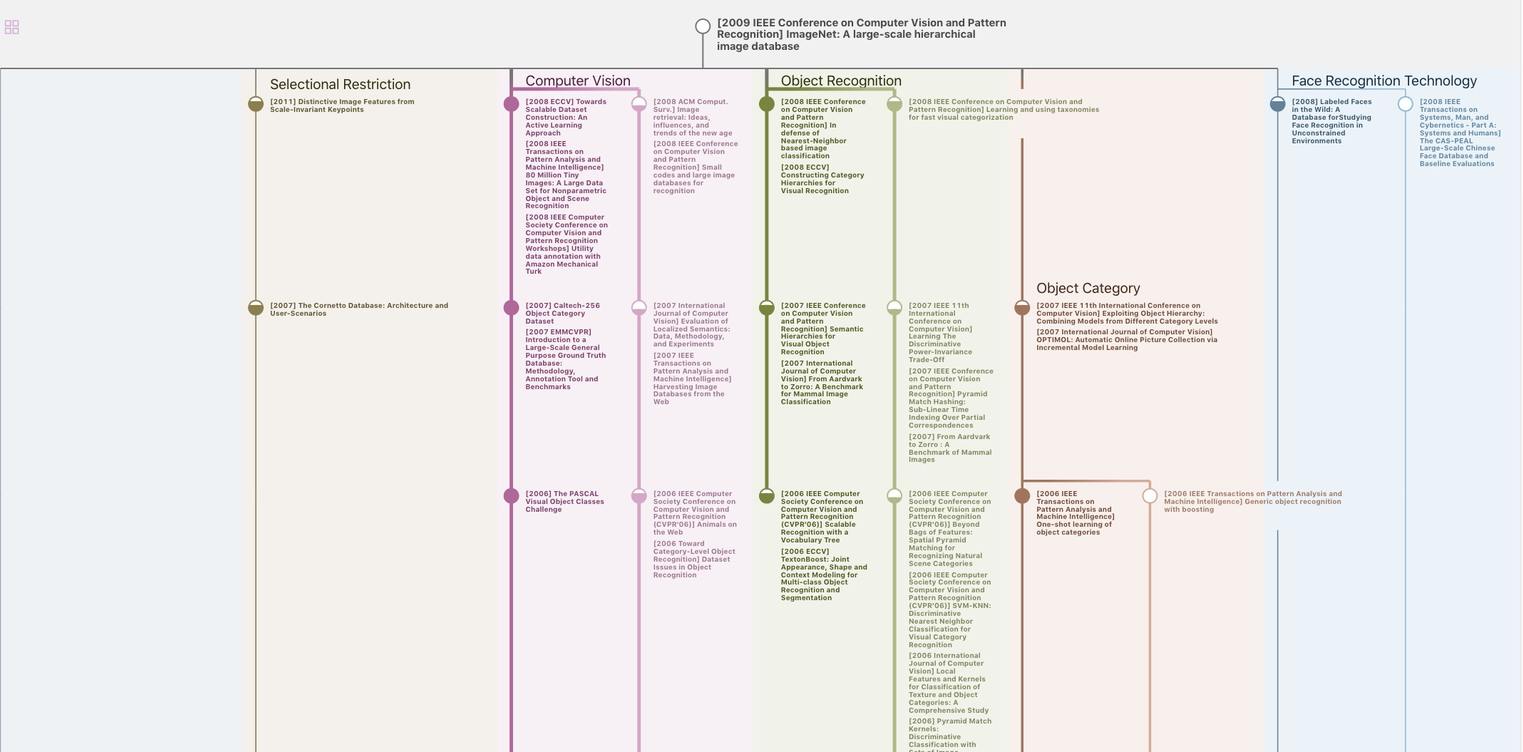
生成溯源树,研究论文发展脉络
Chat Paper
正在生成论文摘要