Production Capacity Prediction and Optimization in the Glycerin Purification Process: A Simulation-Assisted Few-Shot Learning Approach
PROCESSES(2024)
摘要
Chemical process control relies on a tightly controlled, narrow range of margins for critical variables, ensuring process stability and safeguarding equipment from potential accidents. The availability of historical process data is limited to a specific setpoint of operation. This challenge raises issues for process monitoring in predicting and adjusting to deviations outside of the range of operational parameters. Therefore, this paper proposes simulation-assisted deep transfer learning for predicting and optimizing the final purity and production capacity of the glycerin purification process. The proposed network is trained by the simulation domain to generate a base feature extractor, which is then fine-tuned using few-shot learning techniques on the target learner to extend the working domain of the model beyond historical practice. The result shows that the proposed model improved prediction performance by 24.22% in predicting water content and 79.72% in glycerin prediction over the conventional deep learning model. Additionally, the implementation of the proposed model identified production and product quality improvements for enhancing the glycerin purification process.
更多查看译文
关键词
glycerin purification,few-shot learning,production optimization,simulation-assisted
AI 理解论文
溯源树
样例
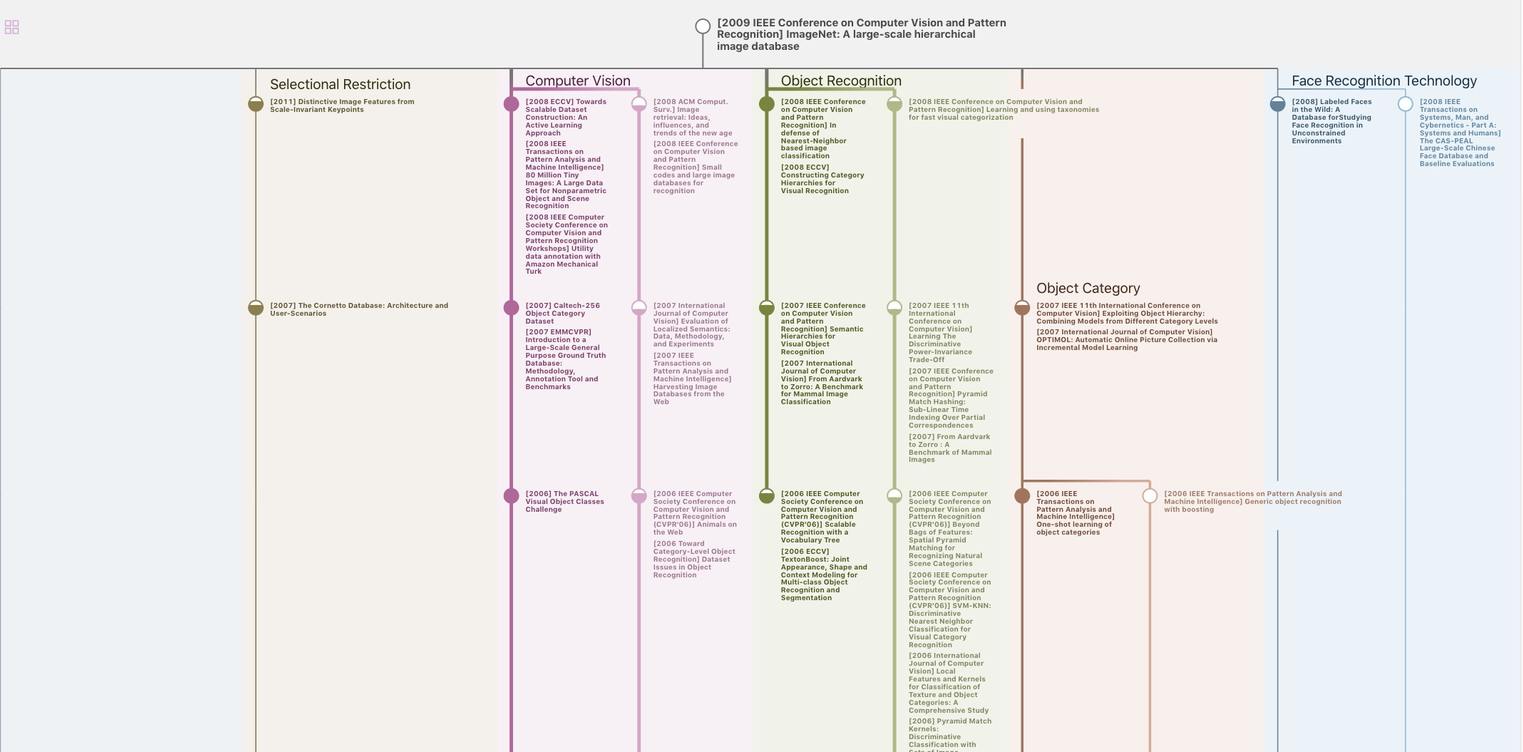
生成溯源树,研究论文发展脉络
Chat Paper
正在生成论文摘要