Dual-student Feature Fusion Knowledge Distillation Model for Wheel Tread Anomaly Detection
2023 IEEE Far East NDT New Technology & Application Forum (FENDT)(2023)
Abstract
The defect of the wheel tread is an important factor affecting the normal work of railway train wheels. Traditional deep learning object detection algorithms require a large number of samples to train models, and their detection performance is limited by the type and quantities of abnormal samples. Faced with the lack of ab-normal samples of wheel tread defects, this paper proposes to use an unsupervised knowledge distillation anomaly detection model to complete the task of anomaly detection on wheel treads. In order to reduce the influence of the non-tread area on the anomaly detection model, the tread area is segmented by Unet, and then a dual-student feature fusion network is designed to improve the reconstruction ability of the student decoder for normal features in the reverse knowledge distillation structure. In training, the two student networks jointly learn the knowledge of normal features from the teacher network, cooperatively memorize the features of normal samples, and play the advantage of fusion features in reasoning to strengthen the reconstruction of normal features by the student network. Experiments show that the improved model can achieve 92.3% AUC, 85.3% precision, 95.3% recall and 88.0% accuracy on the railway wheel tread dataset. Compared to the unimproved model, the model detection performance is greatly improved.
MoreTranslated text
AI Read Science
Must-Reading Tree
Example
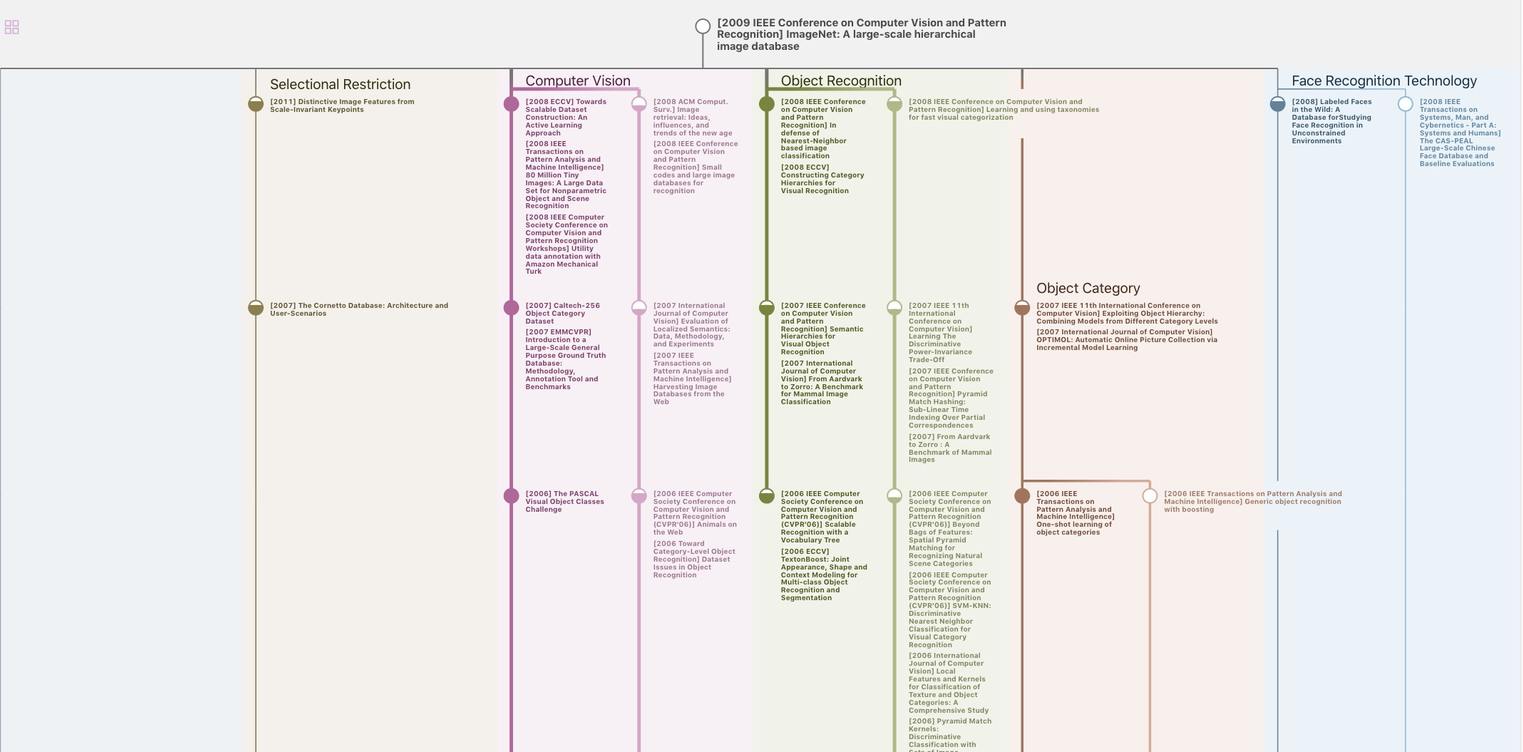
Generate MRT to find the research sequence of this paper
Chat Paper
Summary is being generated by the instructions you defined